Learning deep feature fusion for traffic light detection
JOURNAL OF ENGINEERING RESEARCH(2023)
摘要
Traffic light detection in real-world conditions is challenging because of the positioning of lights, variety in shapes and scales, and similarity with other objects. The paper presents a deep learning-based traffic light detection system by learning the fusion of handcrafted features. The handcrafted features for object detection focus on specific attributes such as shape, color, or texture. The objective of this work is to incorporate handcrafted features into the network learning process such that the resulting detector parameters are robust to input variations, sensor noise, and atmospheric noise. The proposed detection framework is based on the latest You only look once (YOLO) architecture, trained with the fusion of different information channels in the Integral Channel Features (ICF). The approach demonstrates a qualitative approach for identifying the optimal layer for additional feature injection in the network, and the selection of ICF channels to be applied for fusion. The validation of the proposed detector on the Bosch small traffic light dataset achieved the best mAP score of 55.7 % on the testing set. Further, a qualitative comparison of the proposed detector's performance with that of other recent methods is presented, along with an analysis using auxiliary experiments.
更多查看译文
关键词
Object Detection,Traffic Light Detection,YOLO,Handcrafted Features,ICF
AI 理解论文
溯源树
样例
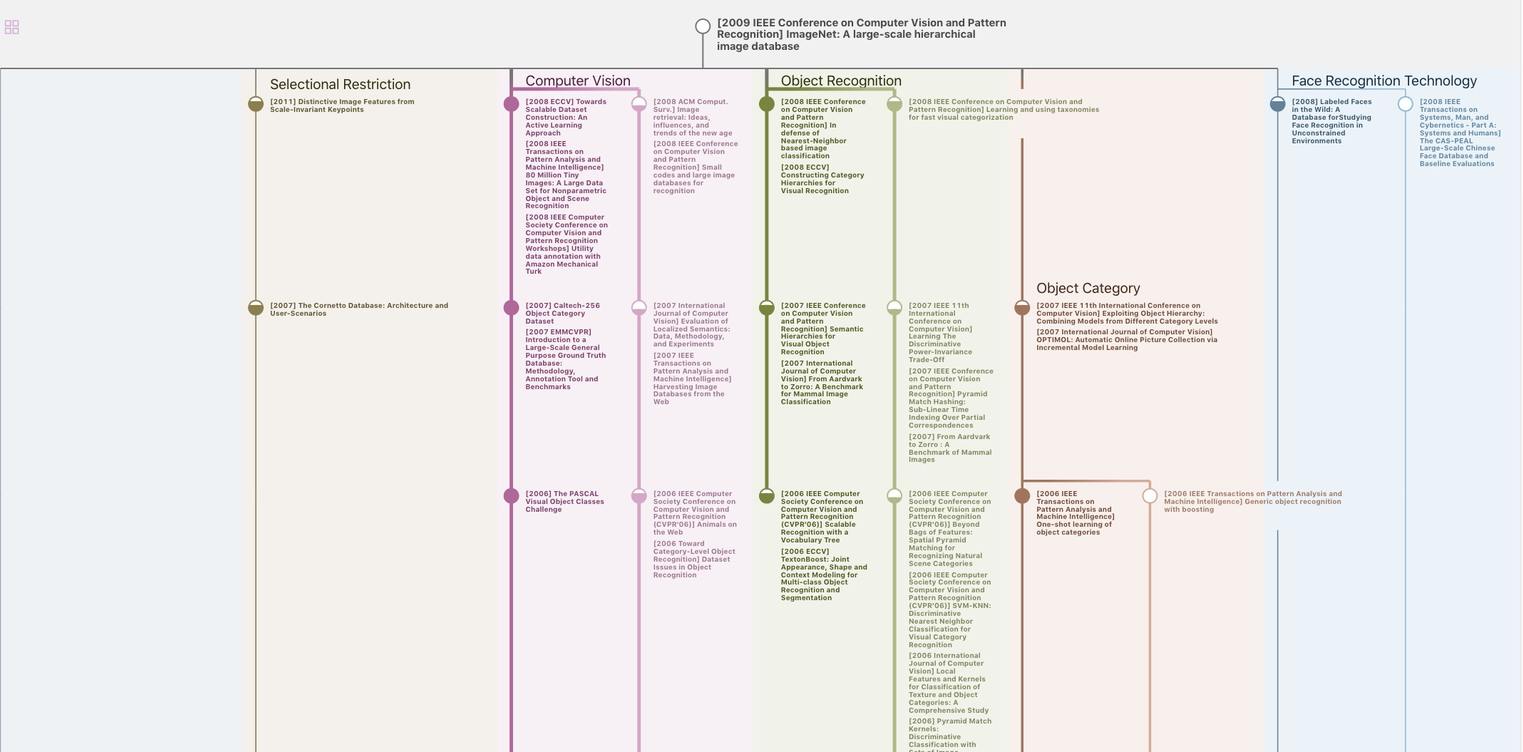
生成溯源树,研究论文发展脉络
Chat Paper
正在生成论文摘要