PASS: A Parameter Audit-Based Secure and Fair Federated Learning Scheme Against Free-Rider Attack.
IEEE Internet of Things Journal(2024)
摘要
Federated learning (FL) as a secure distributed learning framework gains interests in Internet of Things (IoT) due to its capability of protecting the privacy of participant data. However, traditional FL systems are vulnerable to free-rider (FR) attacks, which causes unfairness, privacy leakage and inferior performance to FL systems. The prior defense mechanisms against FR attacks assumed that malicious clients (namely, adversaries) declare less than 50% of the total amount of clients. Moreover, they aimed for anonymous FR (AFR) attacks and lost effectiveness in resisting selfish FR (SFR) attacks. In this article, we propose a parameter audit-based secure and fair FL scheme (PASS) against FR attack. PASS has the following key features: 1) prevent from privacy leakage with less accuracy loss; 2) be effective in countering both AFR and SFR attacks; and 3) work well no matter whether AFR and SFR adversaries occupy the majority of clients or not. Extensive experimental results validate that PASS: 1) has the same level as the state-of-the-art method in mean square error against privacy leakage; 2) defends against AFR and SFR attacks in terms of a higher defense success rate, lower false positive rate, and higher F1-score; and 3) is still effective where adversaries exceed 50%, with F1-score 89% against AFR attack and F1-score 87% against SFR attack. Note that PASS produces no negative effect on FL accuracy when there is no FR adversary.
更多查看译文
关键词
fair federated learning scheme,secure,audit-based,free-rider
AI 理解论文
溯源树
样例
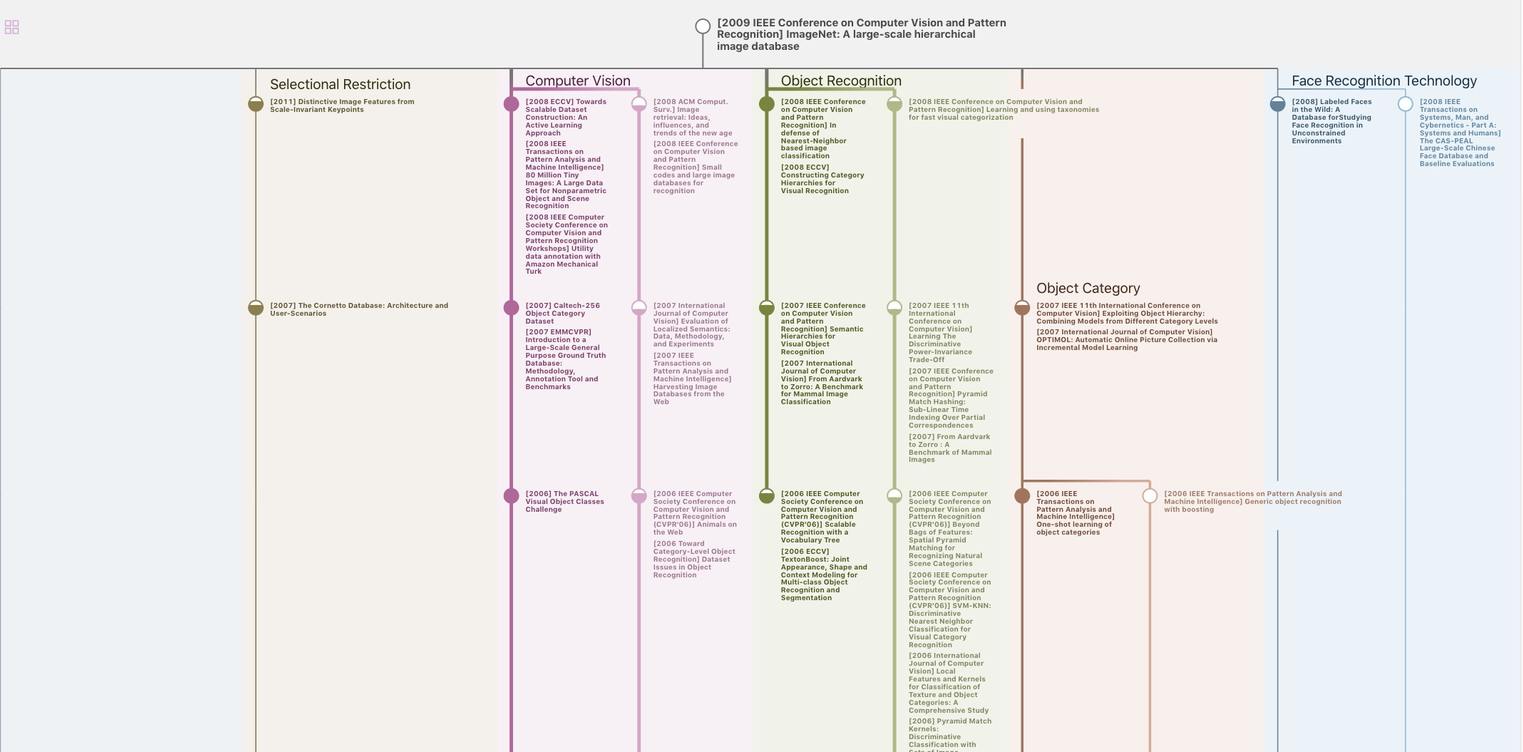
生成溯源树,研究论文发展脉络
Chat Paper
正在生成论文摘要