Verification of Generalizability in Software Log Anomaly Detection Models
Artificial intelligence(2023)
摘要
With recent rapid technological advances, the automatic analysis of software logs has received particular attention. Currently, there is much research on the use of Deep Learning in the field of software log anomaly detection, and they have reported high accuracy of more than 0.9 in the f1-score. On the other hand, there are reports that it has not been used in the field of software development. We conducted a generalized evaluation against representative models for log anomaly detection to elucidate the cause of this problem. Five models were used in the subject: four representative models (two supervised and two unsupervised) and our proposed Neocortical Algorithm (supervised). We used the commonly used Blue Gene/L supercomputer log(BGL) dataset. The learning curves and cross-validation showed a tendency toward overfitting in all models. In addition, a survey of the frequency of log patterns confirmed the need for a more diverse dataset, as many of the patterns are a series of specific logs.
更多查看译文
关键词
generalizability,detection
AI 理解论文
溯源树
样例
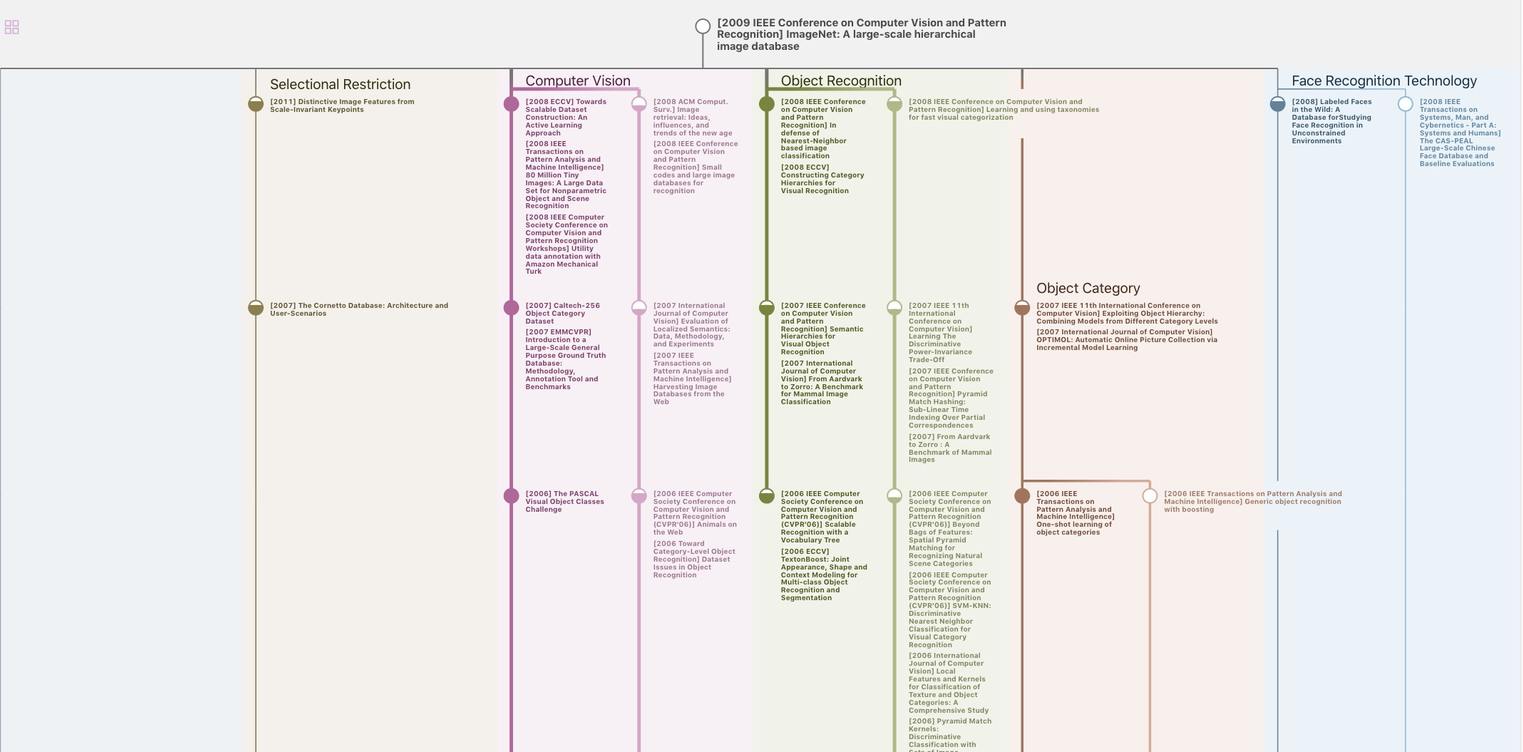
生成溯源树,研究论文发展脉络
Chat Paper
正在生成论文摘要