Above-Ground Biomass Estimation Based on Multi-Angular L-Band Measurements of Brightness Temperatures
IEEE Journal of Selected Topics in Applied Earth Observations and Remote Sensing(2023)
摘要
There is growing interest in using passive microwave observations and Vegetation Optical Depth (VOD) to study the above ground biomass (AGB) and carbon stocks evolution. L-band observations in particular have been shown to be very sensitive to AGB. Here, and thanks to the multi-angle capabilities of the SMOS mission, a new approach to estimate AGB directly from multi-angular L-band brightness temperatures (TBs) is proposed, thus surpassing the use of intermediate variables like VOD. The European Space Agency (ESA) Climate Change Initiative (CCI) Biomass maps for years 2010, 2017 and 2018 are used as AGB reference. AGB estimates from Artificial Neural Networks (ANN) using a purely data-driven approach explained up to 88% of AGB variability globally; Even so, a decrease in retrieval's performance was observed when models are applied to data from years different than the year used for their training. A new training methodology based on multi-year training sets is presented, leading to results showing more stability for temporal analyses. The best set of predictors and an optimal learning data-set configuration are proposed based on an assessment of the accuracy of the estimates. The ANN methodology using brightness temperatures is a promising alternative with respect to the common method of using a parametric function to estimate AGB from VOD. ANNs AGB estimates showed higher correlation with CCI AGB maps (R 2 $\sim$ 0.87 instead of $\sim$ 0.84) and presented a stronger agreement with their spatial structure and less differences in residual maps.
更多查看译文
关键词
Above-ground biomass (AGB),forest biomass,<named-content xmlns:xlink="http://www.w3.org/1999/xlink" xmlns:ali="http://www.niso.org/schemas/ali/1.0/" xmlns:mml="http://www.w3.org/1998/Math/MathML" xmlns:xsi="http://www.w3.org/2001/XMLSchema-instance" content-type="math" xlink:type="simple"> <inline-formula> <tex-math notation="LaTeX">$L$</tex-math> </inline-formula> </named-content>-band,machine learning,neural networks,passive microwaves (PMWs)
AI 理解论文
溯源树
样例
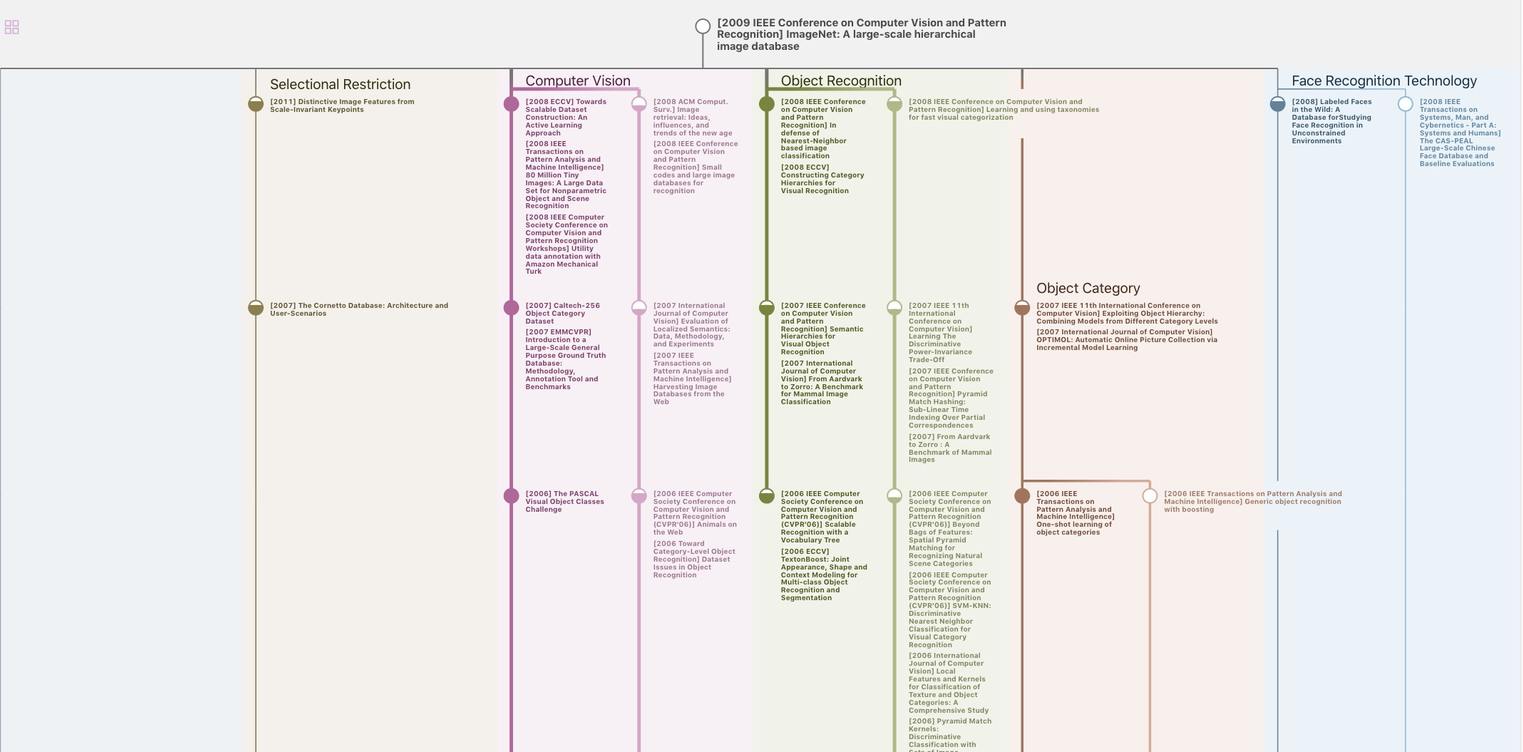
生成溯源树,研究论文发展脉络
Chat Paper
正在生成论文摘要