Fine-Grained Self-Supervision for Generalizable Semantic Segmentation
IEEE TRANSACTIONS ON CIRCUITS AND SYSTEMS FOR VIDEO TECHNOLOGY(2024)
摘要
Unsupervised domain adaptative semantic segmentation is a powerful solution for the distribution shift problem between the source and target domains. However, such methods need specified target domain data that may be unavailable in actual applications due to excess expensive collection. Generalizable semantic segmentation as a new paradigm appears in recent research, which aims to generalize well on distinct unseen domains only using source domain data. The existing methods focus on learning domain-invariant features by using global distribution alignment strategies, which may lead to a decreased discriminability of the model. To cope with this challenge, we propose a fine-grained self-supervision (FGSS) framework for generalizable semantic segmentation that takes into account both discriminability and generalizability from the perspective of the intra-class relationship. The FGSS framework contains single-view and multi-view versions. In the single-view version, we propose a fine-grained self-supervision strategy to distinguish the sub-parts of the semantic class for better class discriminability. In the multi-view version, we propose a class prototype feature enhancement strategy to generate another view (i.e. another representation of the original representation). Then, we propose a multi-view mutual supervision loss to enforce consistency between different views and further enhance the generalizability of the model. Experimental results on five widely-used datasets, i.e., GTAV, SYNTHIA, BDD100K, Cityscapes, and Mapillary, demonstrate that our FGSS framework achieves superior performance compared to state-of-the-art methods.
更多查看译文
关键词
Semantics,Semantic segmentation,Task analysis,Prototypes,Automobiles,Annotations,Training,domain generalization,fine-grained,self-supervision,intra-class relationship
AI 理解论文
溯源树
样例
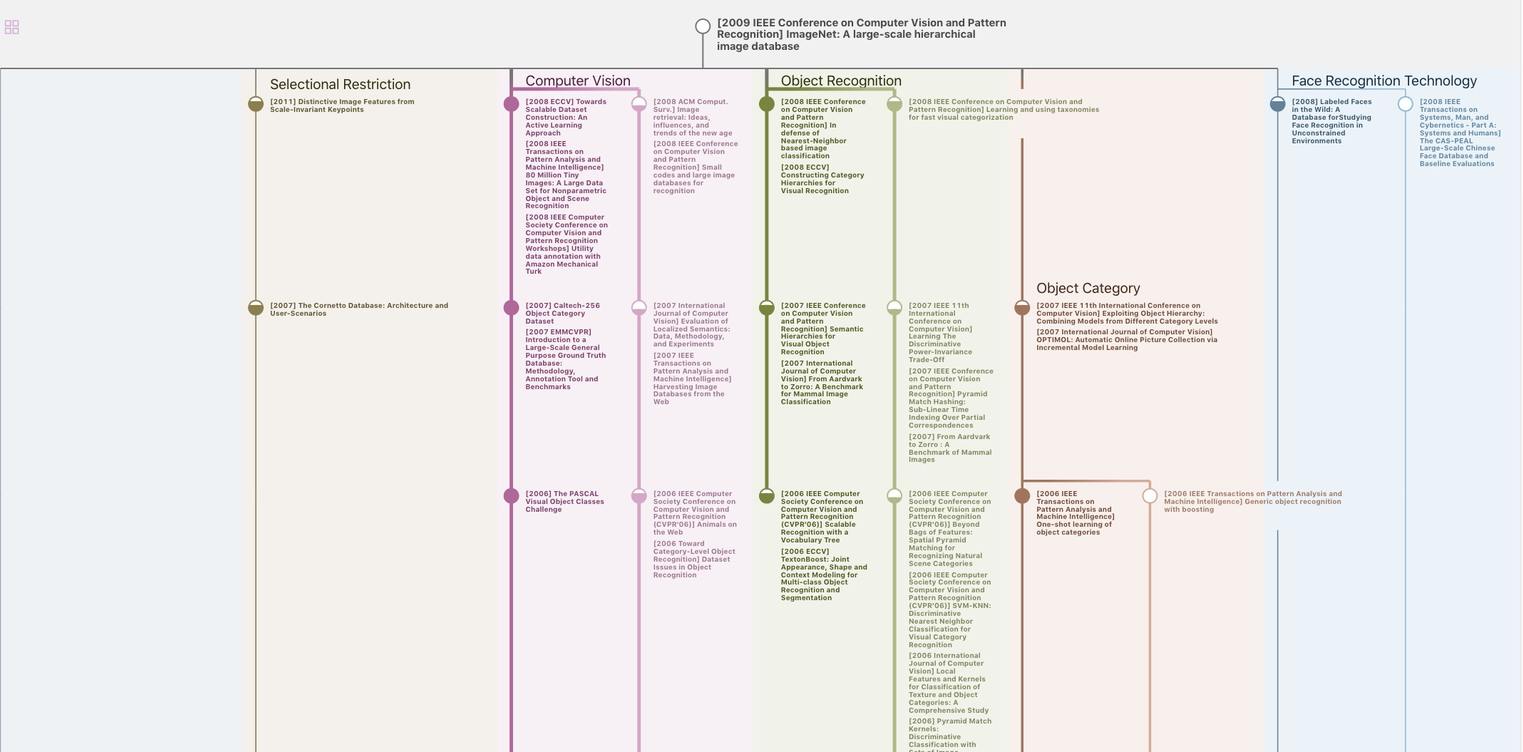
生成溯源树,研究论文发展脉络
Chat Paper
正在生成论文摘要