FaaSwap: SLO-Aware, GPU-Efficient Serverless Inference via Model Swapping
arXiv (Cornell University)(2023)
摘要
The dynamic request patterns of machine learning (ML) inference workloads have driven an increasing trend towards exploiting serverless computing for scalable ML model serving. However, today's serverless platforms lack efficient support for GPUs -- provisioning functions on GPUs incurs extremely high overhead, forcing them to keep long-running even when idling for reduced cold starts. This leads to significant resource waste to perform ML inference and hinders the pay-per-use billing for GPUs. In this paper, we present FaaSwap, a serverless platform enabling fine-grained, request-level GPU sharing for resource-efficient ML inference. FaaSwap leverages model swapping to support fast inference execution at low resource cost. It keeps models in a host which has a large amount of cheap memory and quickly swaps models to GPUs when requested, reducing per-function keep-alive cost and enabling efficient GPU sharing across much more functions. FaaSwap also supports swapping models between GPUs for load balancing and improved inference performance. In FaaSwap, we design sophisticated request scheduling and memory management algorithms that efficiently exploit model swapping to reduce GPU cost and meet latency service-level objectives (SLOs) for all inference functions. We have implemented and integrated FaaSwap into Alibaba Cloud Function Compute (FC), one of the world's largest commercial serverless platform. Evaluation results show that FaaSwap can achieve low-latency model swapping, efficiently share a GPU across hundreds of functions, and satisfy per-function latency SLOs at scale.
更多查看译文
关键词
inference,faaswap,slo-aware,gpu-efficient
AI 理解论文
溯源树
样例
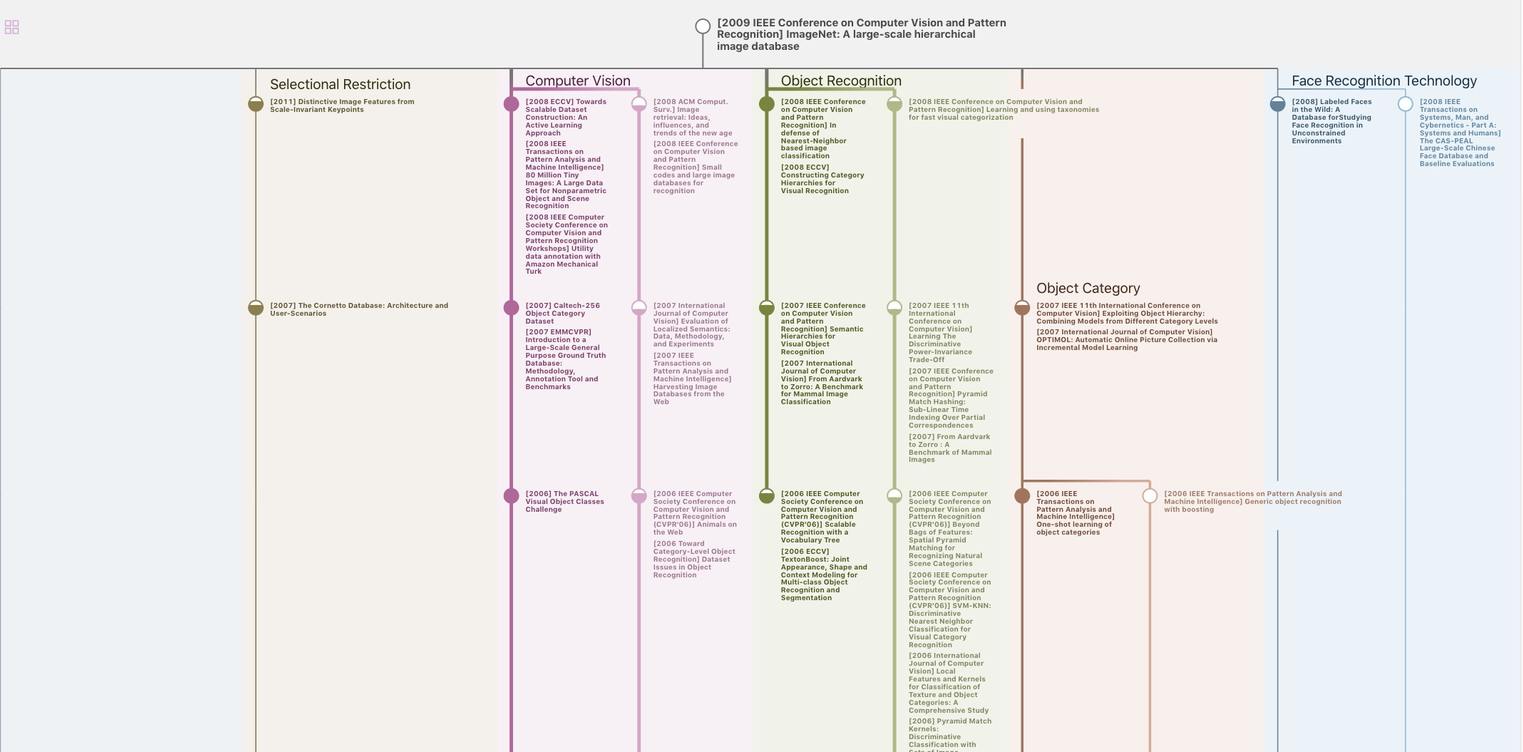
生成溯源树,研究论文发展脉络
Chat Paper
正在生成论文摘要