Variational Monte Carlo with Large Patched Transformers
arXiv (Cornell University)(2023)
摘要
Large language models, like transformers, have recently demonstrated immense powers in text and image generation. This success is driven by the ability to capture long-range correlations between elements in a sequence. The same feature makes the transformer a powerful wavefunction ansatz that addresses the challenge of describing correlations in simulations of qubit systems. We consider two-dimensional Rydberg atom arrays to demonstrate that transformers reach higher accuracies than conventional recurrent neural networks for variational ground state searches. We further introduce large, patched transformer models, which consider a sequence of large atom patches, and show that this architecture significantly accelerates the simulations. The proposed architectures reconstruct ground states with accuracies beyond state-of-the-art quantum Monte Carlo methods, allowing for the study of large Rydberg systems in different phases of matter and at phase transitions. Our high-accuracy ground state representations at reasonable computational costs promise new insights into general large-scale quantum many-body systems.
更多查看译文
关键词
monte
AI 理解论文
溯源树
样例
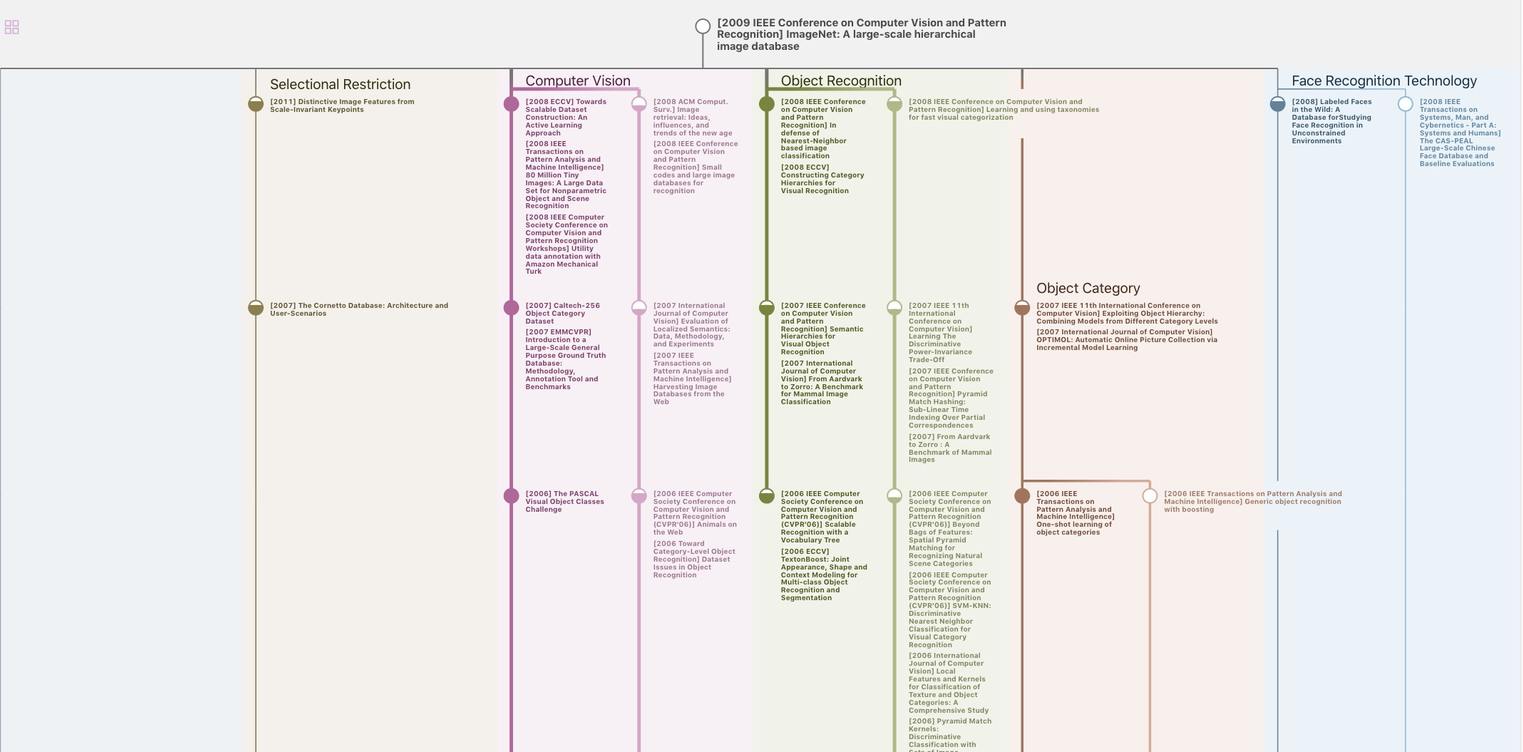
生成溯源树,研究论文发展脉络
Chat Paper
正在生成论文摘要