Investigating Navigation Strategies in the Morris Water Maze through Deep Reinforcement Learning
arXiv (Cornell University)(2023)
摘要
Navigation is a complex skill with a long history of research in animals and humans. In this work, we simulate the Morris Water Maze in 2D to train deep reinforcement learning agents. We perform automatic classification of navigation strategies, analyze the distribution of strategies used by artificial agents, and compare them with experimental data to show similar learning dynamics as those seen in humans and rodents. We develop environment-specific auxiliary tasks and examine factors affecting their usefulness. We suggest that the most beneficial tasks are potentially more biologically feasible for real agents to use. Lastly, we explore the development of internal representations in the activations of artificial agent neural networks. These representations resemble place cells and head-direction cells found in mouse brains, and their presence has correlation to the navigation strategies that artificial agents employ.
更多查看译文
关键词
morris water maze,navigation strategies,reinforcement learning
AI 理解论文
溯源树
样例
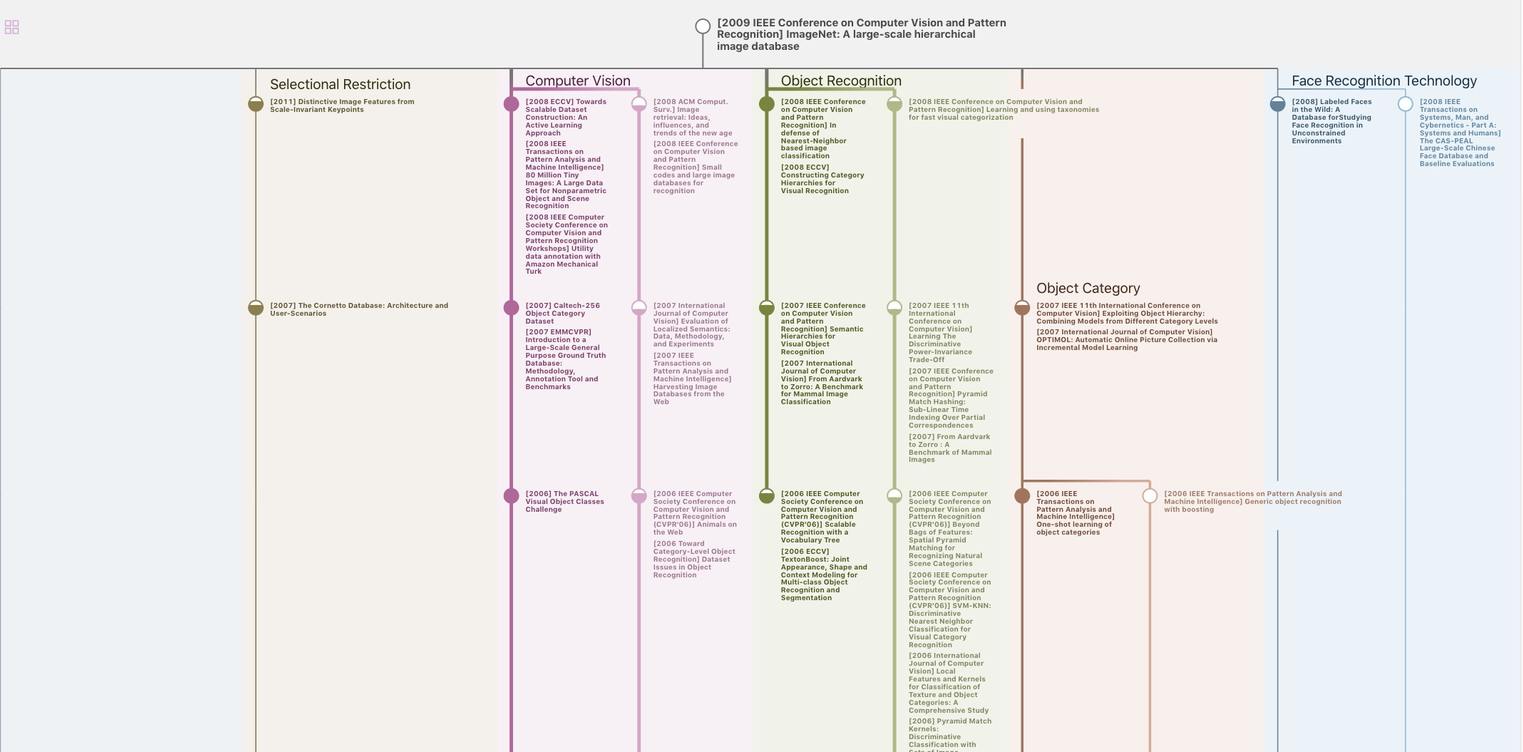
生成溯源树,研究论文发展脉络
Chat Paper
正在生成论文摘要