End-Edge-Cloud Collaboration Based False Data Injection Attack Detection in Distribution Networks
IEEE TRANSACTIONS ON INDUSTRIAL INFORMATICS(2023)
摘要
False data injection attack (FDIA) can pose a severe threat to the distribution networks (DN), and the accurate detection of FDIA plays a key role in the safe and reliable operation of the DN. In this paper, an end-edge-cloud collaboration-based detection framework is proposed to detect FDIA in the DN. First, in order to effectively preserve the privacy of different stakeholders in the DN and solve the problem of data island, a federated learning (FL)-based edge-cloud collaboration mechanism is designed according to the proposed end-edge-cloud collaboration framework to jointly train the local FDIA detection models and eventually build a comprehensive FDIA detection model. Then, considering the temporal-spatial correlation of measurement data, a local data-driven FDIA detection model is proposed based on a novel temporal-spatial graph convolutional network (TSGCN) which can extract temporal-spatial features of the measurement data and improve the FDIA detection performance. In general, compared with the traditional centralized FDIA detection methods, the proposed method can make full use of the computational capacity of distributed edge devices and reduce the pressure of computation on the control centre. Finally, simulation results based on the modified IEEE 14-bus and IEEE 118-bus distribution systems indicate that the proposed method can effectively improve the accuracy of FDIA detection compared with other methods.
更多查看译文
关键词
Distribution networks,false data injection attack,attack detection,end-edge-cloud collaboration,graph convolutional network,federated learning
AI 理解论文
溯源树
样例
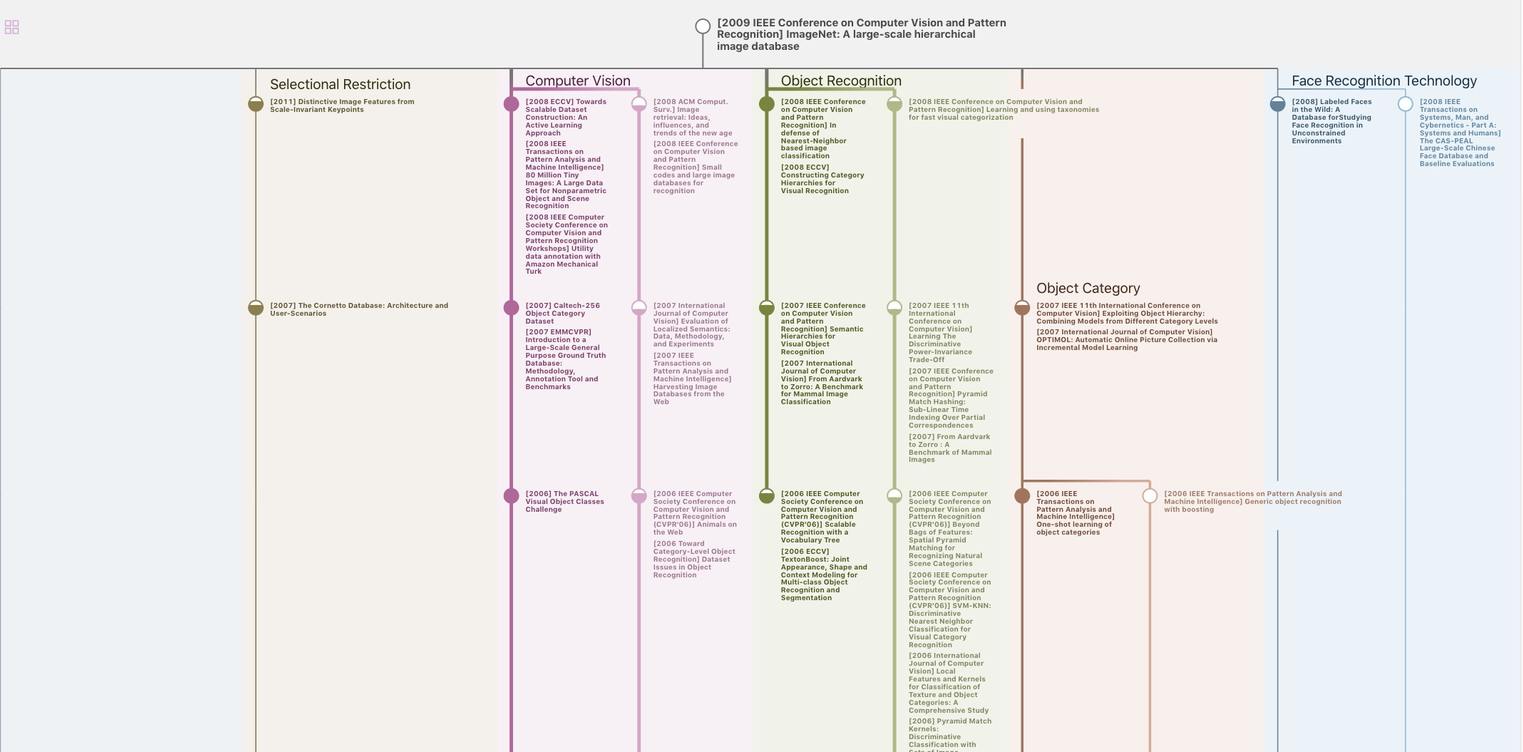
生成溯源树,研究论文发展脉络
Chat Paper
正在生成论文摘要