Video Killed the HD-Map: Predicting Multi-Agent Behavior Directly From Aerial Images.
arXiv (Cornell University)(2023)
摘要
The development of algorithms that learn multi-agent behavioral models using human demonstrations has led to increasingly realistic simulations in the field of autonomous driving. In general, such models learn to jointly predict trajectories for all controlled agents by exploiting road context information such as drivable lanes obtained from manually annotated high-definition (HD) maps. Recent studies show that these models can greatly benefit from increasing the amount of human data available for training. However, the manual annotation of HD maps which is necessary for every new location puts a bottleneck on efficiently scaling up human traffic datasets. We propose an aerial image-based map (AIM) representation that requires minimal annotation and provides rich road context information for traffic agents like pedestrians and vehicles. We evaluate multi-agent trajectory prediction using the AIM by incorporating it into a differentiable driving simulator as an image-texture-based differentiable rendering module. Our results demonstrate competitive multi-agent trajectory prediction performance especially for pedestrians in the scene when using our AIM representation as compared to models trained with rasterized HD maps.
更多查看译文
关键词
Aerial Images,Multi-agent Behavior,Predictive Performance,Behavioral Model,Competitive Performance,Manual Annotation,Realistic Simulation,Simulated Field,Map Representation,Trajectory Prediction,Rich Context,Rich Contextual Information,Convolutional Neural Network,Probabilistic Model,Validation Dataset,Recurrent Neural Network,Intersection Over Union,Minimum Error,Graph Neural Networks,Graph Convolutional Network,Traffic Conditions,Bird’s Eye,Traffic Light,Ground Truth Trajectory,Displacement Error,Texture Map,Types Of Agents,Covariate Shift
AI 理解论文
溯源树
样例
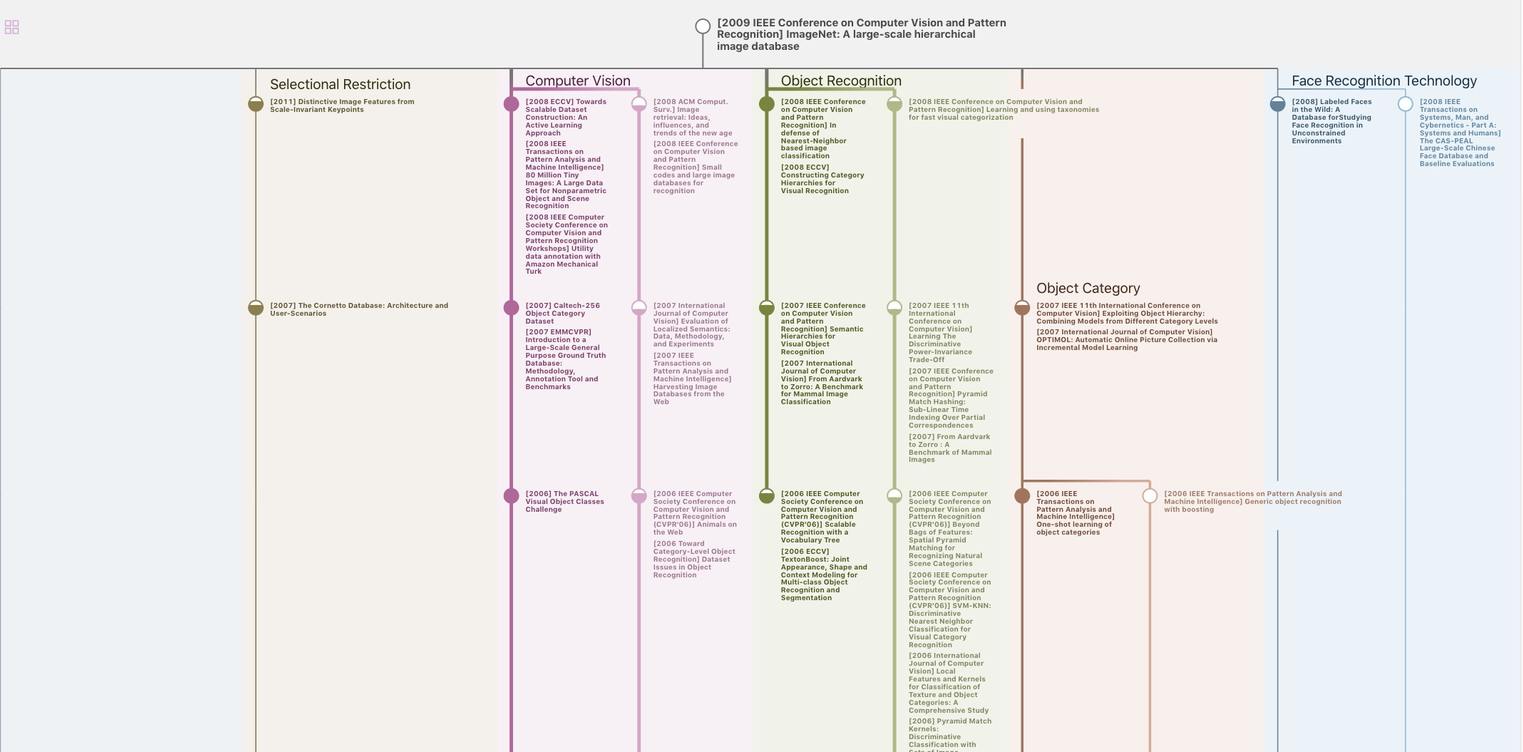
生成溯源树,研究论文发展脉络
Chat Paper
正在生成论文摘要