Latent Representation Prediction Networks
International Journal of Pattern Recognition and Artificial Intelligence(2021)
摘要
Deeply-learned planning methods are often based on learning representations that are optimized for unrelated tasks. For example, they might be trained on reconstructing the environment. These representations are then combined with predictor functions for simulating rollouts to navigate the environment. We find this principle of learning representations unsatisfying and propose to learn them such that they are directly optimized for the task at hand: to be maximally predictable for the predictor function. This results in representations that are by design optimal for the downstream task of planning, where the learned predictor function is used as a forward model. To this end, we propose a new way of jointly learning this representation along with the prediction function, a system we dub Latent Representation Prediction Network (LARP). The prediction function is used as a forward model for search on a graph in a viewpoint-matching task and the representation learned to maximize predictability is found to outperform a pre-trained representation. Our approach is shown to be more sample-efficient than standard reinforcement learning methods and our learned representation transfers successfully to dissimilar objects.
更多查看译文
关键词
prediction,networks,representation
AI 理解论文
溯源树
样例
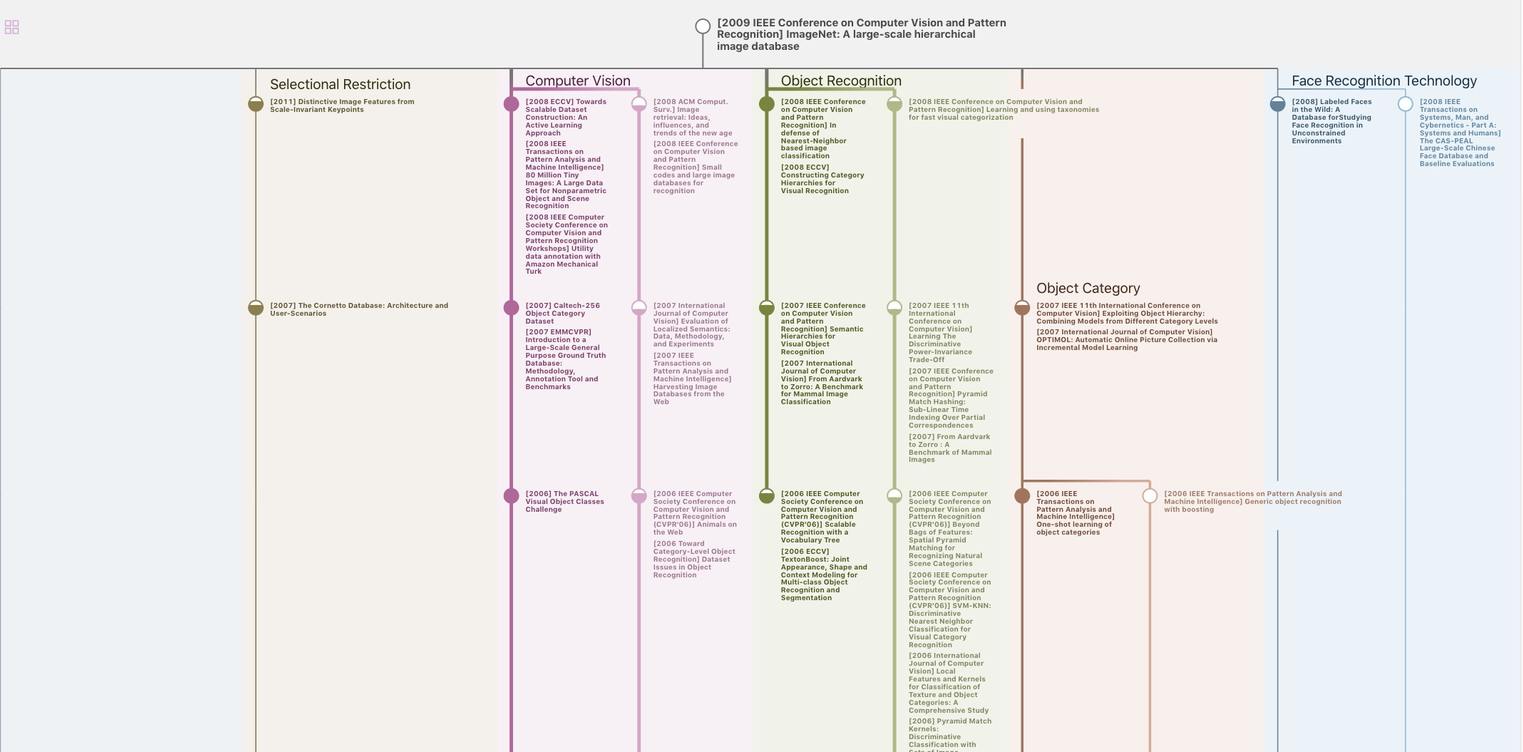
生成溯源树,研究论文发展脉络
Chat Paper
正在生成论文摘要