Using Deep Learning to Detect Pediatric Congenital Heart Disease by Chest Radiography
Research Square (Research Square)(2021)
摘要
Abstract Purpose: To detect congenital heart disease (CHD) patients is a challenging task in low-income areas with limited resources. Chest radiography is usually available in these areas, but the diagnostic accuracy needs to be improved. The aim of the study was to establish a deep learning-based diagnostic tool for paediatric CHD patients using chest radiography. Methods: Totally 11,105 chest radiographs from 11,105 paediatric patients in two centres were labeled, and a convolutional neural network (CNN) was trained for diagnosing CHD in the dataset from Hospital 1. To demonstrate the generalizability and the clinical usefulness of CNN, the accuracy in test set from two centres were both reported. We also trained another CNN to evaluate pulmonary blood flow (PBF) on chest radiographs of the patients. Moreover, the predictive results of both CNN were compared to the decisions of three experienced radiologists (range 10–35 years) without any patient information. Results: The accuracy of CNN for detecting CHD was 81.4% (95% confidence interval, [78.3%–84.2%]) in Hospital 2, while the accuracy was 85.6% (95% confidence interval, [84.0%–87.1%]) in Hospital 1. The overall accuracy of CNN for evaluating PBF was 78.9% (95% confidence interval, [74.3%–83.0%]). Compared to three experienced radiologists, CNN showed statistically non-inferiority at a 5% margin for the accuracy (P < 0.025) in detecting CHD and evaluating PBF. Conclusions: The deep learning-based diagnostic tool we developed could effectively detect CHD and evaluate for PBF on chest radiography. It can potentially aid the radiologists and cardiologists in the future.
更多查看译文
关键词
pediatric congenital heart disease,congenital heart disease,chest radiography,deep learning,heart disease
AI 理解论文
溯源树
样例
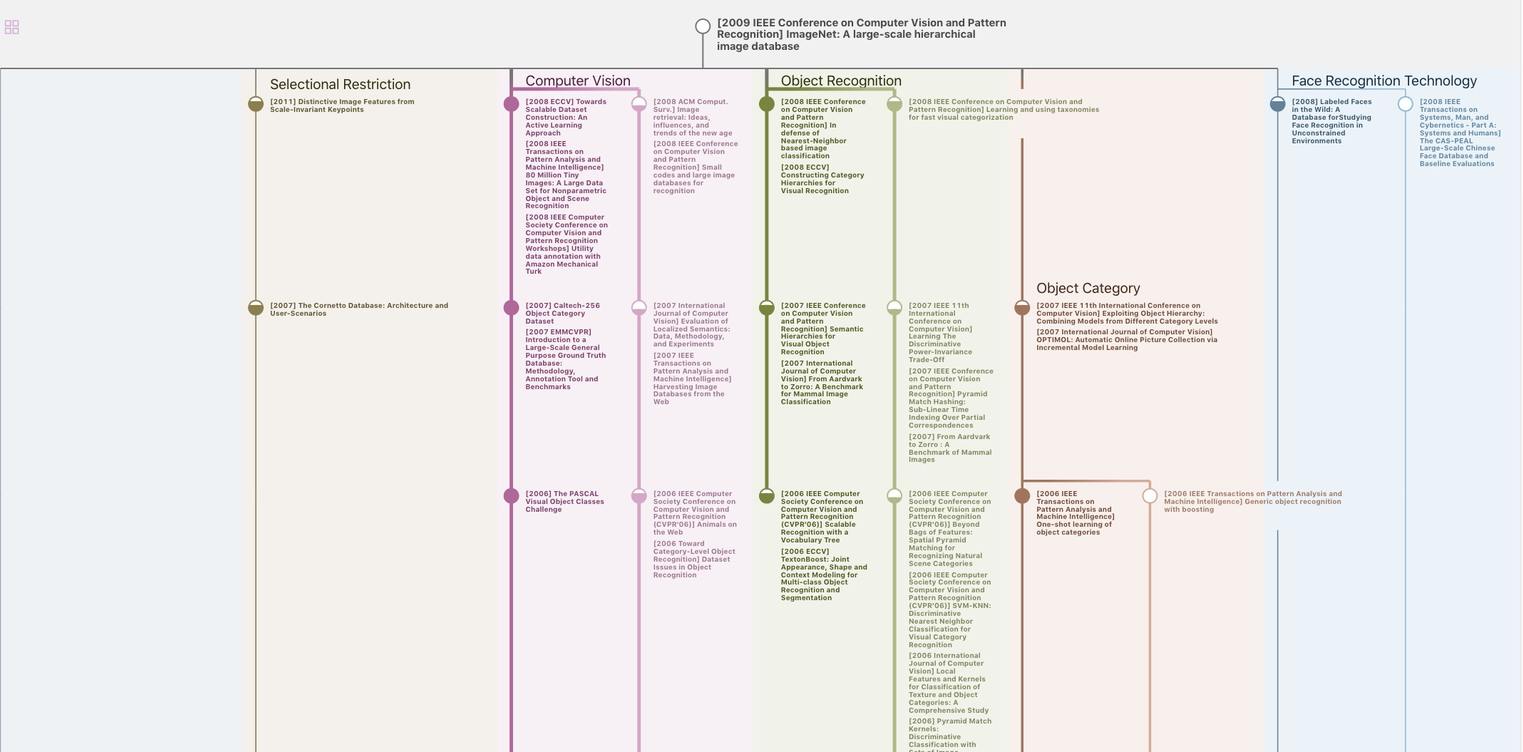
生成溯源树,研究论文发展脉络
Chat Paper
正在生成论文摘要