Grand rounds in methodology: key considerations for implementing machine learning solutions in quality improvement initiatives
BMJ QUALITY & SAFETY(2024)
摘要
Machine learning (ML) solutions are increasingly entering healthcare. They are complex, sociotechnical systems that include data inputs, ML models, technical infrastructure and human interactions. They have promise for improving care across a wide range of clinical applications but if poorly implemented, they may disrupt clinical workflows, exacerbate inequities in care and harm patients. Many aspects of ML solutions are similar to other digital technologies, which have well-established approaches to implementation. However, ML applications present distinct implementation challenges, given that their predictions are often complex and difficult to understand, they can be influenced by biases in the data sets used to develop them, and their impacts on human behaviour are poorly understood. This manuscript summarises the current state of knowledge about implementing ML solutions in clinical care and offers practical guidance for implementation. We propose three overarching questions for potential users to consider when deploying ML solutions in clinical care: (1) Is a clinical or operational problem likely to be addressed by an ML solution? (2) How can an ML solution be evaluated to determine its readiness for deployment? (3) How can an ML solution be deployed and maintained optimally? The Quality Improvement community has an essential role to play in ensuring that ML solutions are translated into clinical practice safely, effectively, and ethically.
更多查看译文
关键词
healthcare quality improvement,implementation science,information technology,quality improvement methodologies
AI 理解论文
溯源树
样例
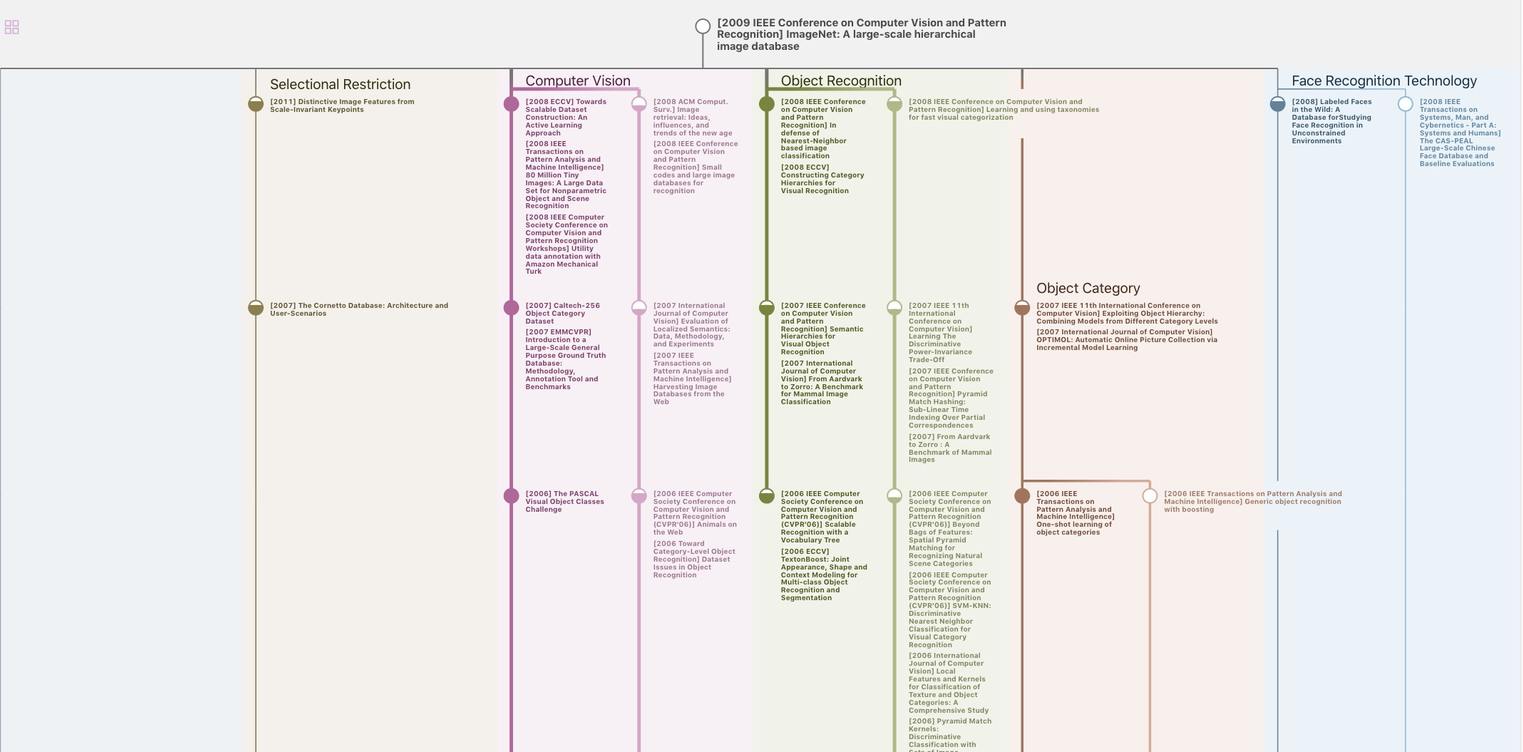
生成溯源树,研究论文发展脉络
Chat Paper
正在生成论文摘要