Causal Inference in Microbiomes Using Intervention Calculus
bioRxiv (Cold Spring Harbor Laboratory)(2020)
摘要
Abstract Inferring causal effects is critically important in biomedical research as it allows us to move from the typical paradigm of associational studies to causal inference, and can impact treatments and therapeutics. Association patterns can be coincidental and may lead to wrong inferences in complex systems. Microbiomes are highly complex, diverse, and dynamic environments. Microbes are key players in health and diseases. Hence knowledge of genuine causal relationships among the entities in a microbiome, and the impact of internal and external factors on microbial abundance and interactions are essential for understanding disease mechanisms and making treatment recommendations. In this paper, we investigate fundamental causal inference techniques to measure the causal effects of various entities in a microbiome. In particular, we show how to use these techniques on microbiome datasets to study the rise and impact of antibiotic-resistance in microbiomes. Our main contributions include the following. We introduce a novel pipeline for microbiome studies, new ideas for experimental design under weaker assumptions, and data augmentation by context embedding. Our pipeline is robust, different from traditional approaches, and able to predict interventional effects without any controlled experiments. Our work shows the advantages of causal inference in identifying potential pathogenic, beneficial, and antibiotic-resistant bacteria. We validate our results using results that were previously published.
更多查看译文
关键词
microbiomes,causal inference,intervention calculus
AI 理解论文
溯源树
样例
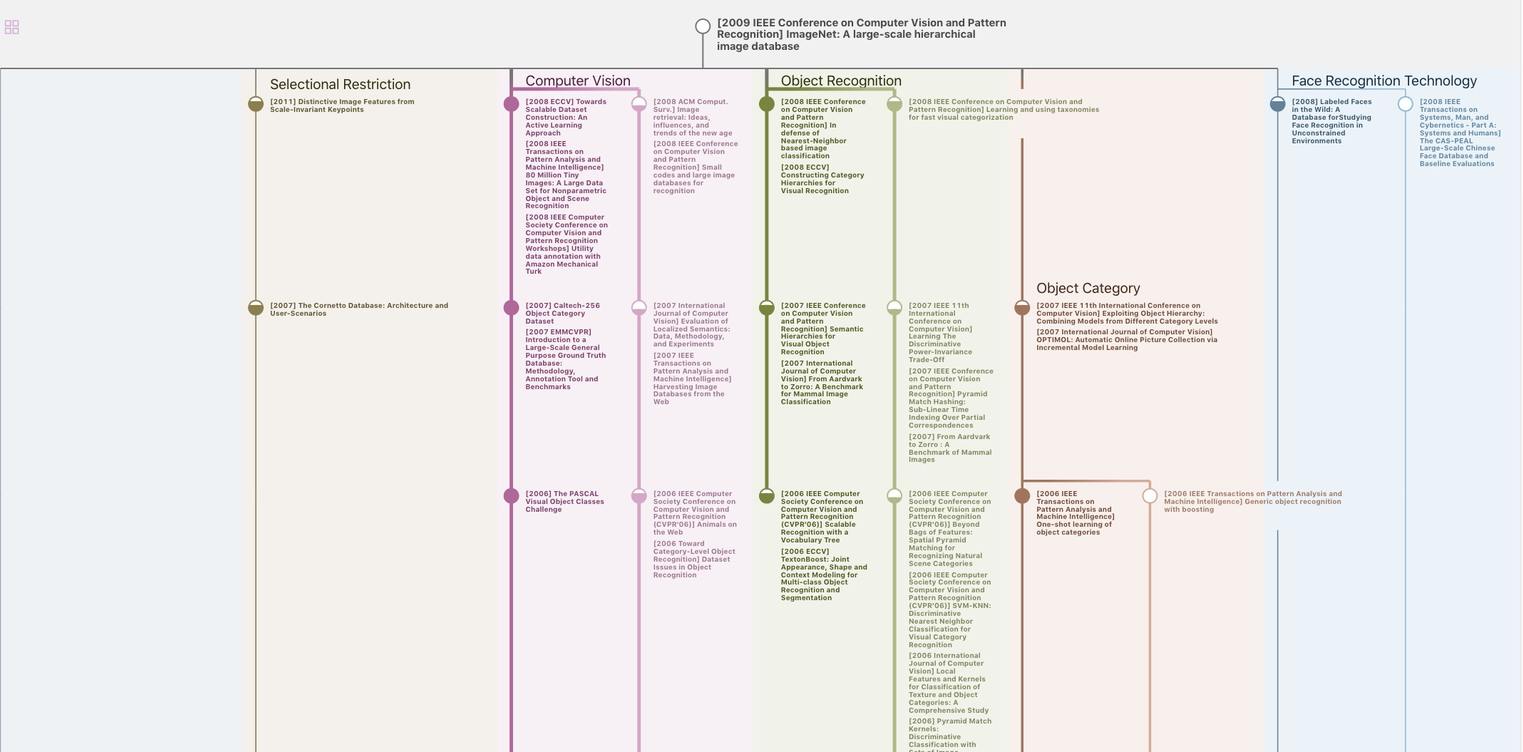
生成溯源树,研究论文发展脉络
Chat Paper
正在生成论文摘要