Unsupervised Self-Driving Attention Prediction via Uncertainty Mining and Knowledge Embedding
arXiv (Cornell University)(2023)
摘要
Predicting attention regions of interest is an important yet challenging task for self-driving systems. Existing methodologies rely on large-scale labeled traffic datasets that are labor-intensive to obtain. Besides, the huge domain gap between natural scenes and traffic scenes in current datasets also limits the potential for model training. To address these challenges, we are the first to introduce an unsupervised way to predict self-driving attention by uncertainty modeling and driving knowledge integration. Our approach's Uncertainty Mining Branch (UMB) discovers commonalities and differences from multiple generated pseudo-labels achieved from models pre-trained on natural scenes by actively measuring the uncertainty. Meanwhile, our Knowledge Embedding Block (KEB) bridges the domain gap by incorporating driving knowledge to adaptively refine the generated pseudo-labels. Quantitative and qualitative results with equivalent or even more impressive performance compared to fully-supervised state-of-the-art approaches across all three public datasets demonstrate the effectiveness of the proposed method and the potential of this direction. The code will be made publicly available.
更多查看译文
关键词
uncertainty mining
AI 理解论文
溯源树
样例
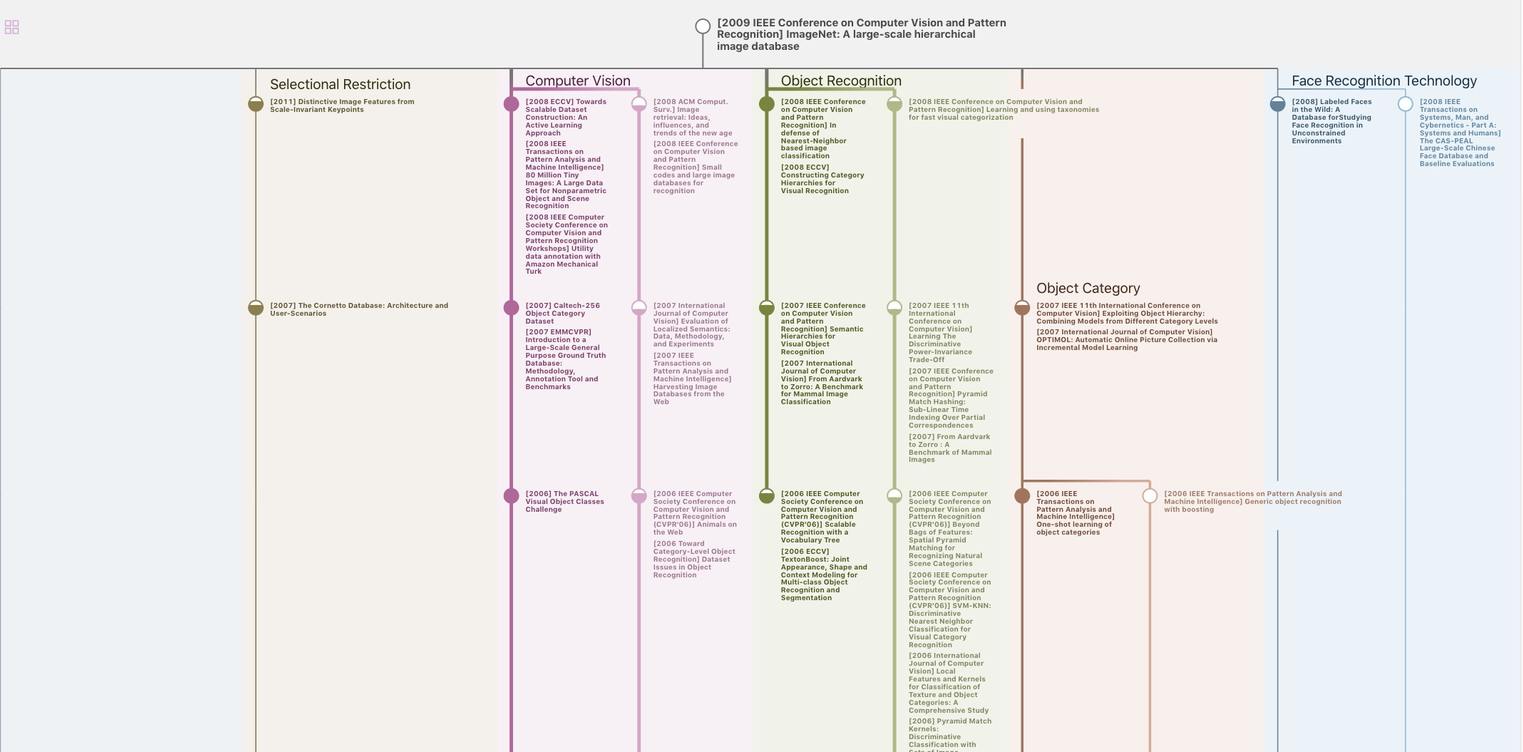
生成溯源树,研究论文发展脉络
Chat Paper
正在生成论文摘要