H-GAP: Humanoid Control with a Generalist Planner
ICLR 2024(2023)
摘要
Humanoid control is an important research challenge offering avenues for integration into human-centric infrastructures and enabling physics-driven humanoid animations. The daunting challenges in this field stem from the difficulty of optimizing in high-dimensional action spaces and the instability introduced by the bipedal morphology of humanoids. However, the extensive collection of human motion-captured data and the derived datasets of humanoid trajectories, such as MoCapAct, paves the way to tackle these challenges. In this context, we present Humanoid Generalist Autoencoding Planner (H-GAP), a state-action trajectory generative model trained on humanoid trajectories derived from human motion-captured data, capable of adeptly handling downstream control tasks with Model Predictive Control (MPC). For 56 degrees of freedom humanoid, we empirically demonstrate that H-GAP learns to represent and generate a wide range of motor behaviours. Further, without any learning from online interactions, it can also flexibly transfer these behaviors to solve novel downstream control tasks via planning. Notably, H-GAP excels established MPC baselines that have access to the ground truth dynamics model, and is superior or comparable to offline RL methods trained for individual tasks. Finally, we do a series of empirical studies on the scaling properties of H-GAP, showing the potential for performance gains via additional data but not computing. Code and videos are available at https://ycxuyingchen.github.io/hgap/.
更多查看译文
关键词
Generative Modelling,Humanoid Control,Model Predictive Control,Model-based Reinforcement Learning,Offline Reinforcement Learning
AI 理解论文
溯源树
样例
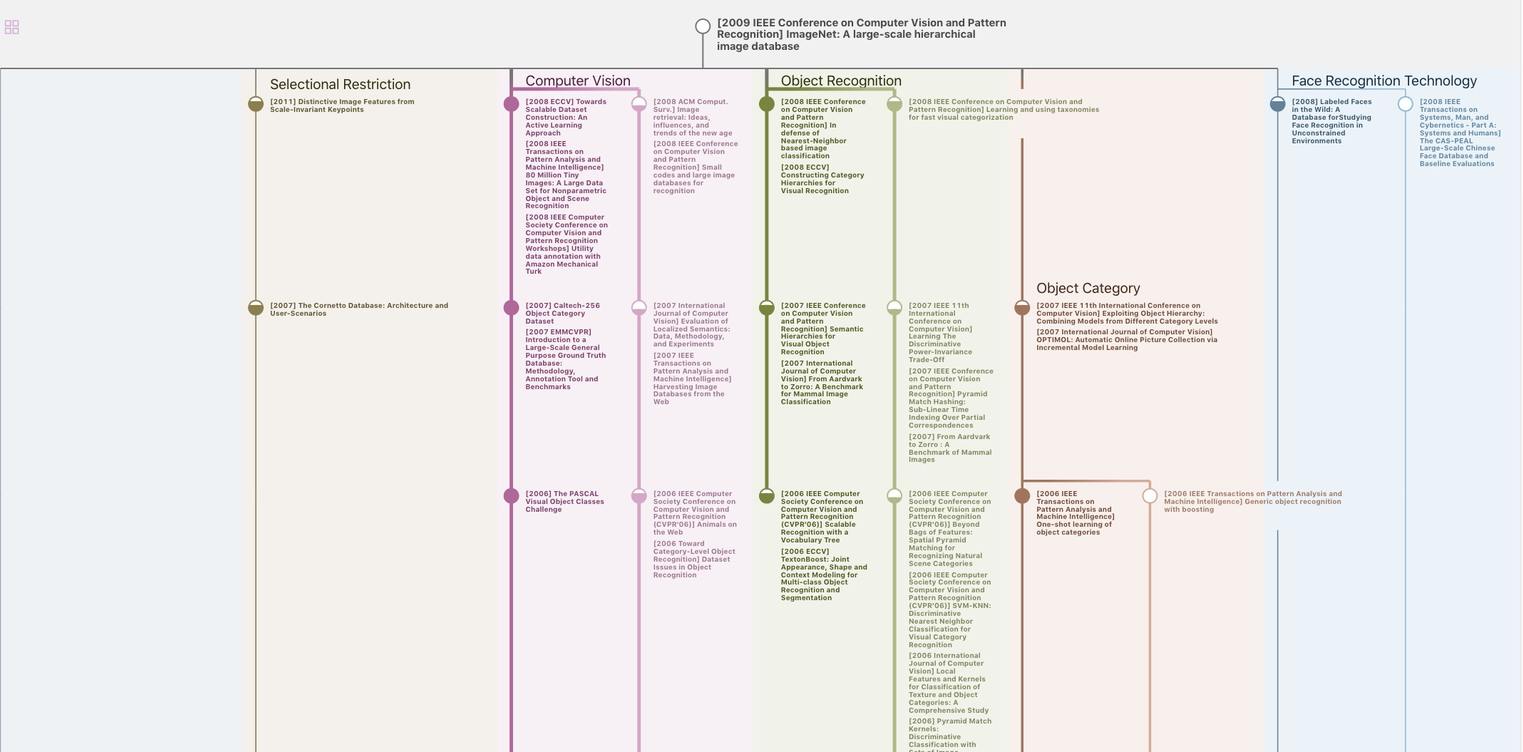
生成溯源树,研究论文发展脉络
Chat Paper
正在生成论文摘要