FLea: Improving federated learning on scarce and label-skewed data via privacy-preserving feature augmentation
CoRR(2023)
摘要
Learning a global model by abstracting the knowledge, distributed across
multiple clients, without aggregating the raw data is the primary goal of
Federated Learning (FL). Typically, this works in rounds alternating between
parallel local training at several clients, followed by model aggregation at a
server. We found that existing FL methods under-perform when local datasets are
small and present severe label skew as these lead to over-fitting and local
model bias. This is a realistic setting in many real-world applications. To
address the problem, we propose \textit{FLea}, a unified framework that tackles
over-fitting and local bias by encouraging clients to exchange
privacy-protected features to aid local training. The features refer to
activations from an intermediate layer of the model, which are obfuscated
before being shared with other clients to protect sensitive information in the
data. \textit{FLea} leverages a novel way of combining local and shared
features as augmentations to enhance local model learning. Our extensive
experiments demonstrate that \textit{FLea} outperforms the start-of-the-art FL
methods, sharing only model parameters, by up to $17.6\%$, and FL methods that
share data augmentations by up to $6.3\%$, while reducing the privacy
vulnerability associated with shared data augmentations.
更多查看译文
AI 理解论文
溯源树
样例
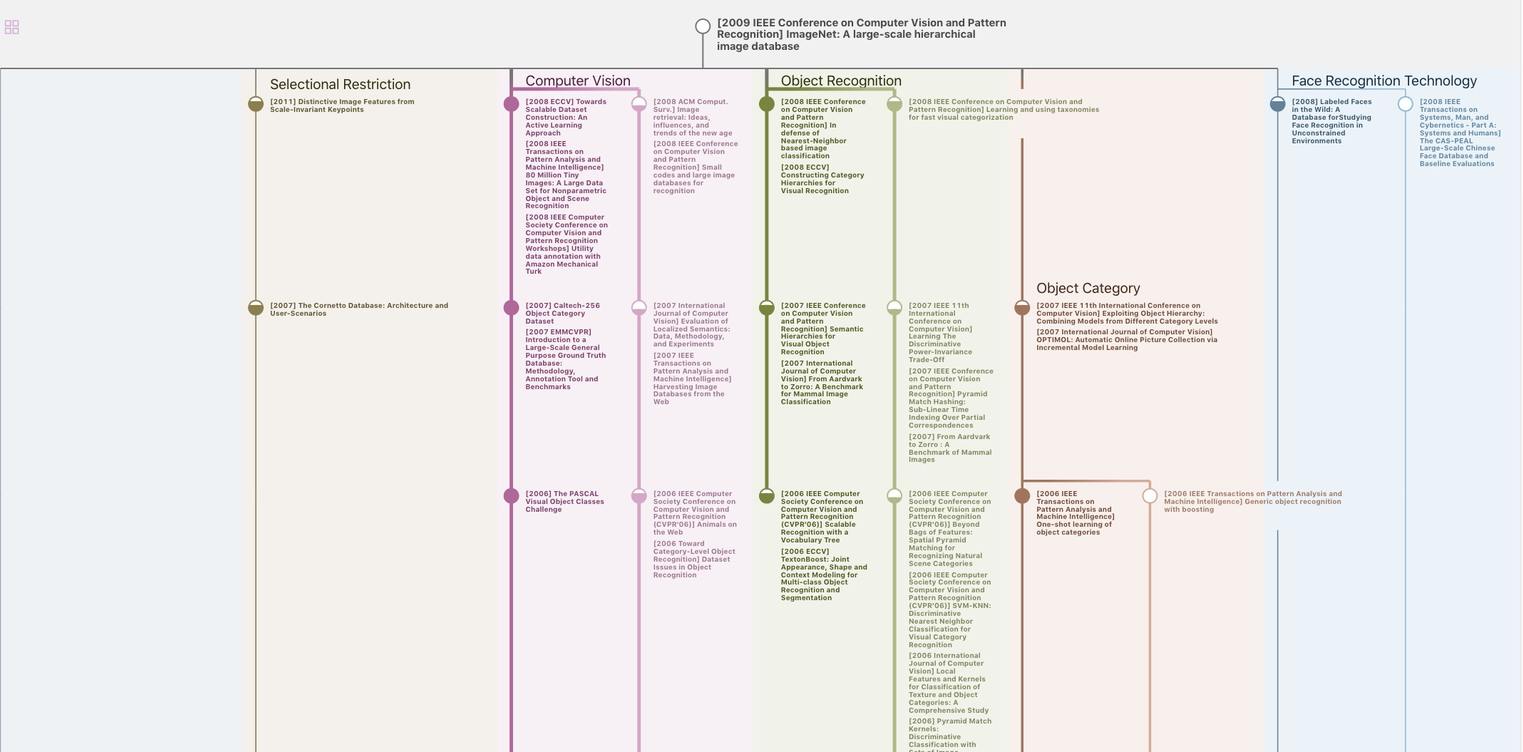
生成溯源树,研究论文发展脉络
Chat Paper
正在生成论文摘要