KNCM: Kernel Neutrosophic c-Means Clustering
Zenodo (CERN European Organization for Nuclear Research)(2019)
摘要
Abstract Data clustering is an important step in data mining and machine learning. It is especially crucial to analyze the data structures for further procedures. Recently a new clustering algorithm known as ‘neutrosophic c-means’ (NCM) was proposed in order to alleviate the limitations of the popular fuzzy c-means (FCM) clustering algorithm by introducing a new objective function which contains two types of rejection. The ambiguity rejection which concerned patterns lying near the cluster boundaries, and the distance rejection was dealing with patterns that are far away from the clusters. In this paper, we extend the idea of NCM for nonlinear-shaped data clustering by incorporating the kernel function into NCM. The new clustering algorithm is called Kernel Neutrosophic c-Means (KNCM), and has been evaluated through extensive experiments. Nonlinear-shaped toy datasets, real datasets and images were used in the experiments for demonstrating the efficiency of the proposed method. A comparison between Kernel FCM (KFCM) and KNCM was also accomplished in order to visualize the performance of both methods. According to the obtained results, the proposed KNCM produced better results than KFCM.
更多查看译文
关键词
clustering,kncm,c-means
AI 理解论文
溯源树
样例
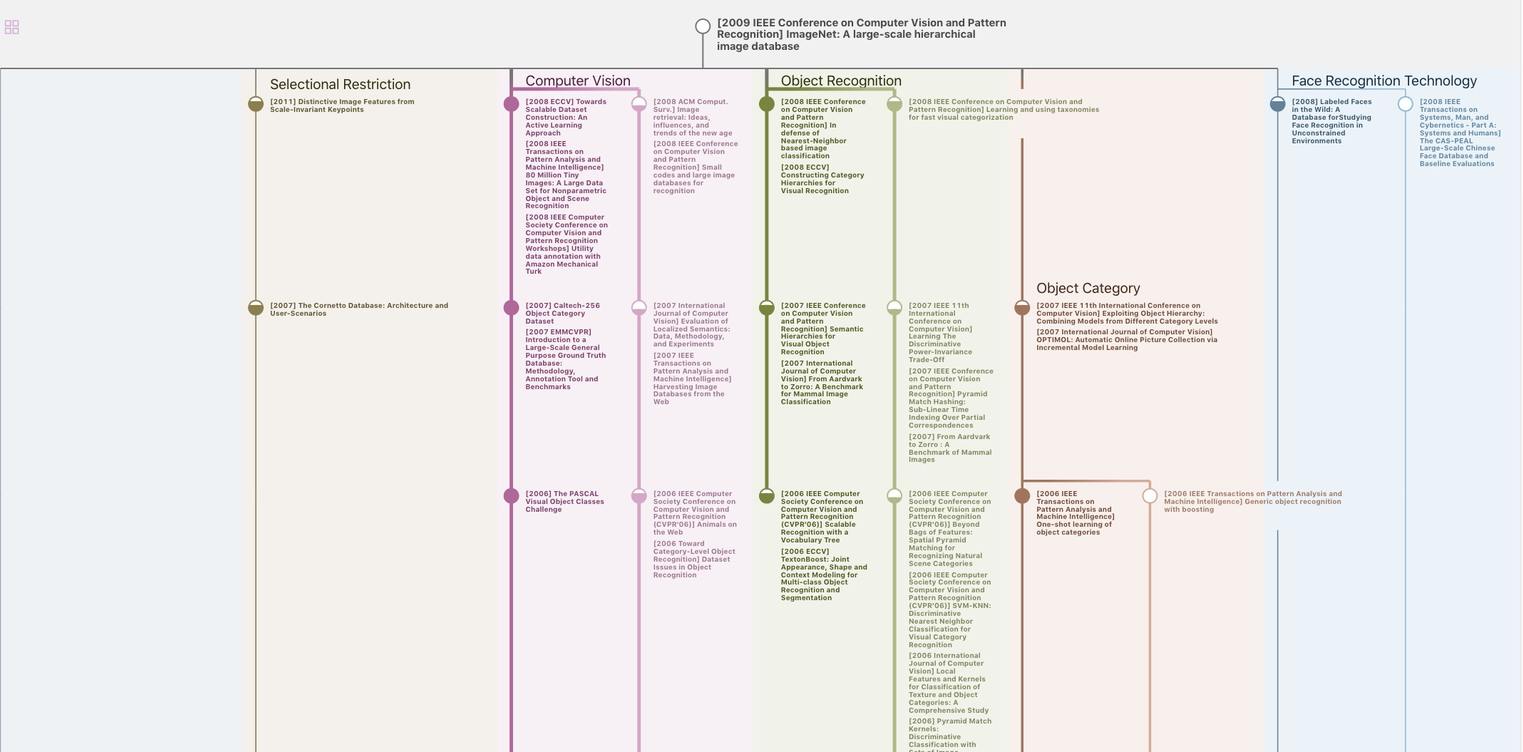
生成溯源树,研究论文发展脉络
Chat Paper
正在生成论文摘要