Noise Budgeting in Multiple-Kernel Word-Length Optimization
HAL (Le Centre pour la Communication Scientifique Directe)(2019)
摘要
Word-Length Optimization (WLO) is a key step in the implementation of Digital Signal Processing applications on hardware platforms. Existing approaches face scalability problems for applications with several kernels. In this paper, we present our work-in-progress to address the scalability problems when performing multi-kernel WLO. Our approach uses application-wide analysis to derive noise budgets for each kernel, followed by independent WLO. The main idea is to characterize the impact of approximating each kernel to the accuracy/cost through simulation and regression analysis. The constructed
models can be used to determine the appropriate noise budget for each kernel. When applied to WLO for two kernels in Image Signal Processor, the noise budgets given by our analysis closely match those found empirically with global WLO.
更多查看译文
关键词
optimization,budgeting,noise,multiple-kernel,word-length
AI 理解论文
溯源树
样例
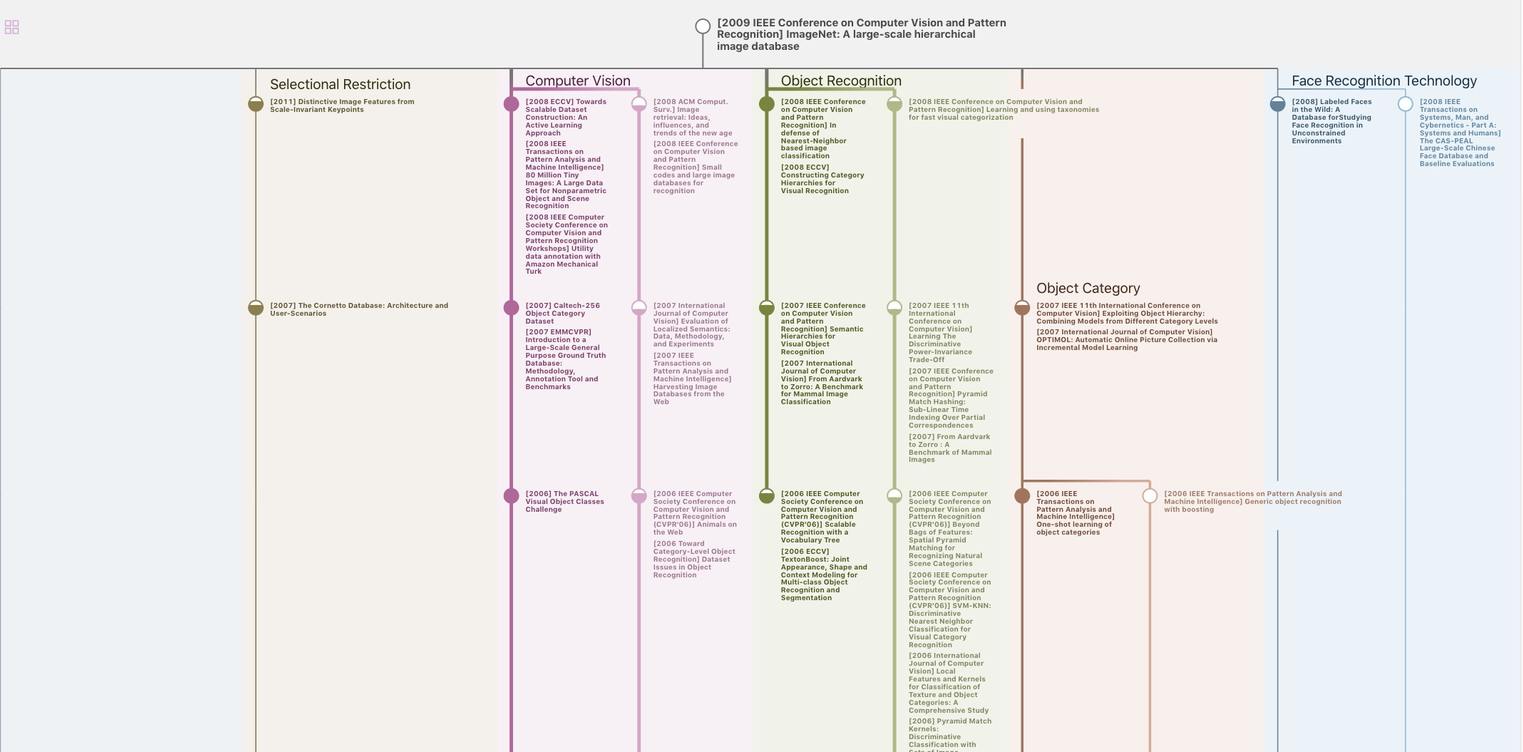
生成溯源树,研究论文发展脉络
Chat Paper
正在生成论文摘要