Online Load Balancing for Related Machines
arXiv (Cornell University)(2017)
摘要
In the load balancing problem, introduced by Graham in the 1960s (SIAM J. of Appl. Math. 1966, 1969), jobs arriving online have to be assigned to machines so to minimize an objective defined on machine loads. A long line of work has addressed this problem for both the makespan norm and arbitrary $\ell_q$-norms of machine loads. Recent literature (e.g., Azar et al., STOC 2013; Im et al., FOCS 2015) has further expanded the scope of this problem to vector loads, to capture jobs with multi-dimensional resource requirements in applications such as data centers. In this paper, we completely resolve the job scheduling problem for both scalar and vector jobs on related machines, i.e., where each machine has a given speed and the time taken to process a job is inversely proportional to the speed of the machine it is assigned on. We show the following results. For scalar scheduling, we give a constant competitive algorithm for optimizing any $\ell_q$-norm for related machines. The only previously known result was for the makespan norm. For vector scheduling, there are two natural variants for vector scheduling, depending on whether the speed of a machine is dimension-dependent or not. We show a sharp contrast between these two variants, proving that they are respectively equivalent to unrelated machines and identical machines for the makespan norm. We also extend these results to arbitrary $\ell_q$-norms of the machine loads. No previous results were known for vector scheduling on related machines.
更多查看译文
关键词
balancing,related machines,online,load
AI 理解论文
溯源树
样例
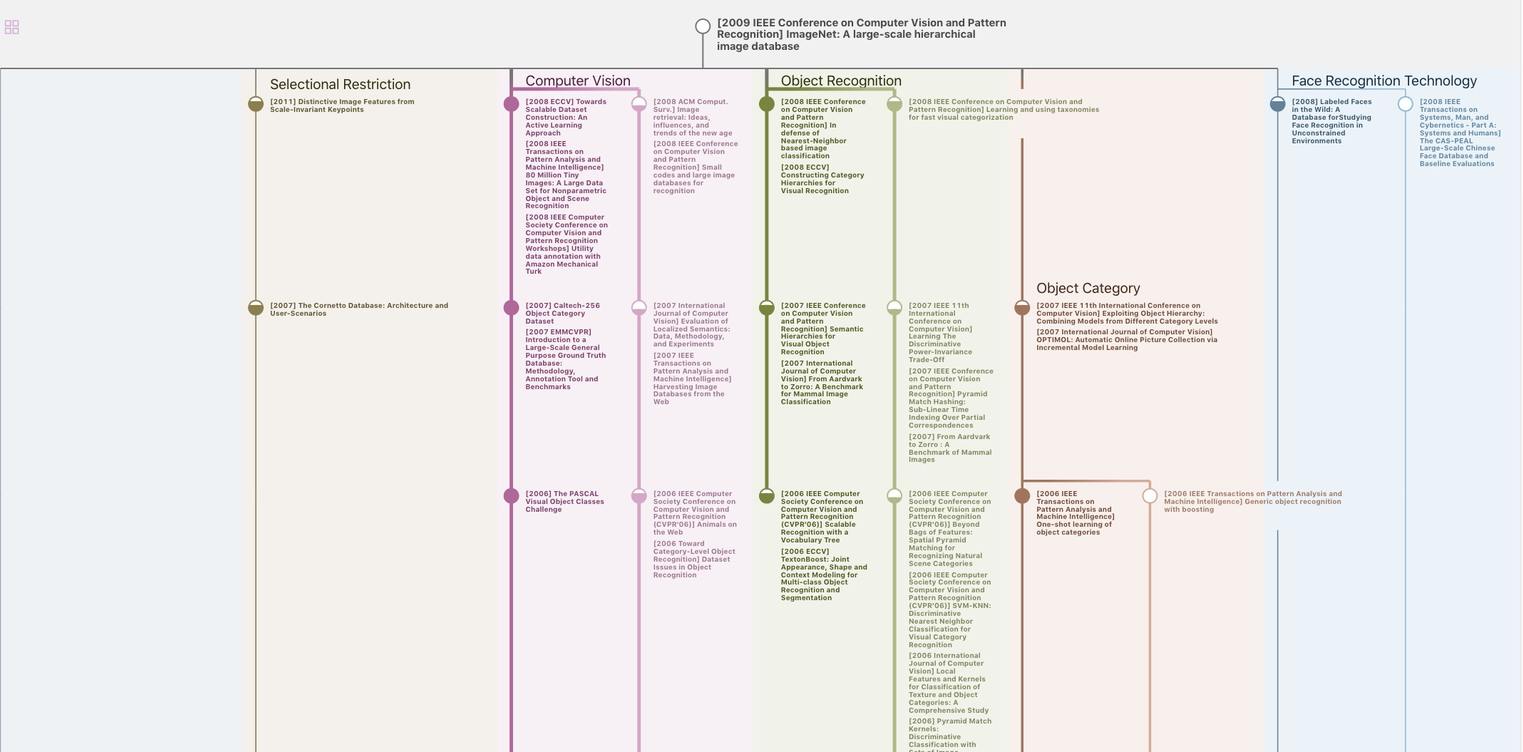
生成溯源树,研究论文发展脉络
Chat Paper
正在生成论文摘要