Machine Learning Methods for Inferring the Number of UAV Emitters via Massive MIMO Receive Array
arXiv (Cornell University)(2022)
摘要
To provide important prior knowledge for the DOA estimation of UAV emitters in future wireless networks, we present a complete DOA preprocessing system for inferring the number of emitters via massive MIMO receive array. Firstly, in order to eliminate the noise signals, two high-precision signal detectors, square root of maximum eigenvalue times minimum eigenvalue (SR-MME) and geometric mean (GM), are proposed. Compared to other detectors, SR-MME and GM can achieve a high detection probability while maintaining extremely low false alarm probability. Secondly, if the existence of emitters is determined by detectors, we need to further confirm their number. Therefore, we perform feature extraction on the the eigenvalue sequence of sample covariance matrix to construct feature vector and innovatively propose a multi-layer neural network (ML-NN). Additionally, the support vector machine (SVM), and naive Bayesian classifier (NBC) are also designed. The simulation results show that the machine learning-based methods can achieve good results in signal classification, especially neural networks, which can always maintain the classification accuracy above 70\% with massive MIMO receive array. Finally, we analyze the classical signal classification methods, Akaike (AIC) and Minimum description length (MDL). It is concluded that the two methods are not suitable for scenarios with massive MIMO arrays, and they also have much worse performance than machine learning-based classifiers.
更多查看译文
关键词
uav emitters,mimo,machine learning methods,machine learning
AI 理解论文
溯源树
样例
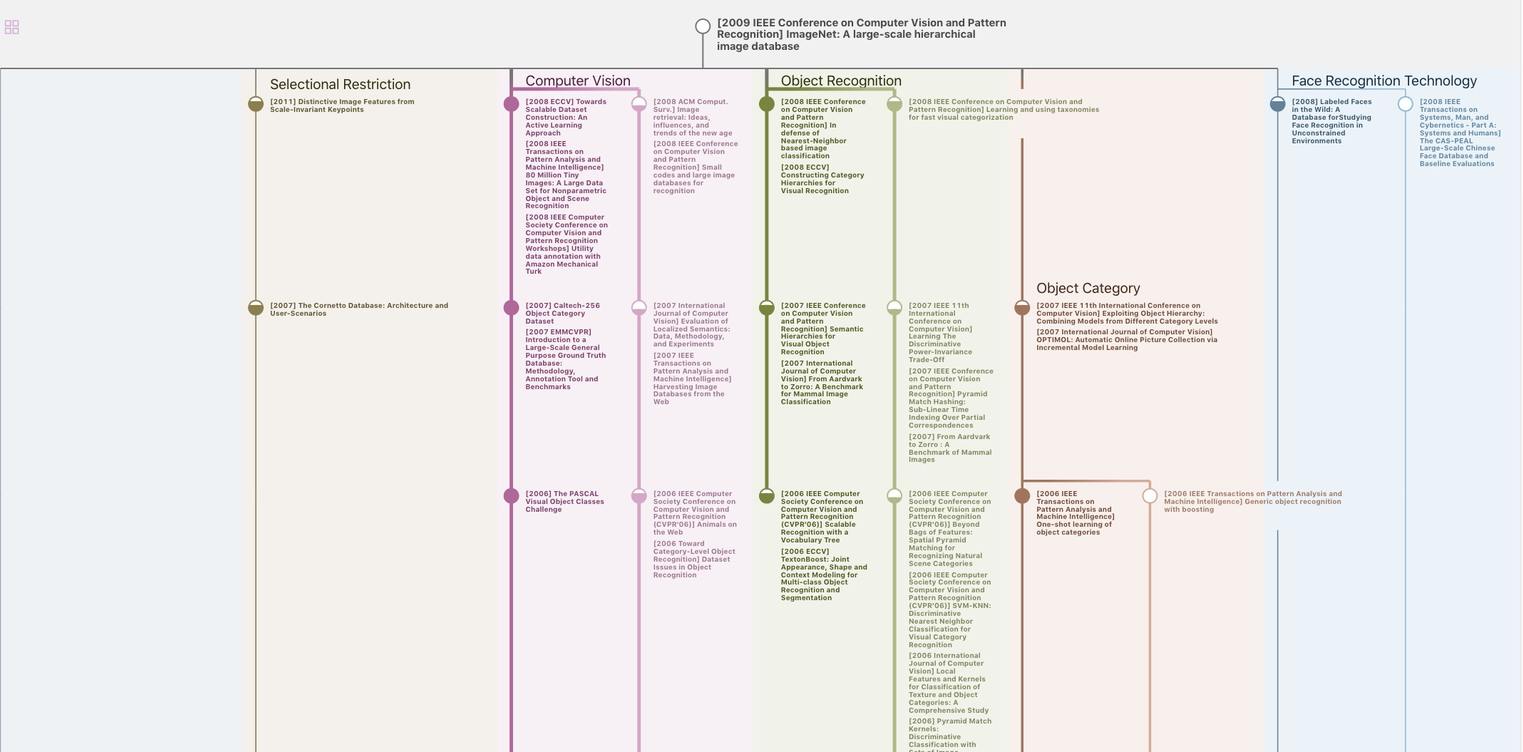
生成溯源树,研究论文发展脉络
Chat Paper
正在生成论文摘要