Multi-task Deep Learning for Glaucoma Detection From Color Fundus Images
Research Square (Research Square)(2022)
摘要
Abstract Glaucoma is an eye condition that, if not diagnosed in time, leads to loss of vision and blindness. While diagnosing campaigns are regularly launched, these require human experts to estimate in a limited time subtle changes in the shape of the optic disc from retinal fundus images. Automatic glaucoma detection methods are desirable to help with the screening of patients, reducing the diagnosis time by analyzing the increasing amount of images. Deep learning methods have been satisfactory to classify and segment diseases in retinal fundus images. To guarantee a good generalization, these methods require vast amounts of annotations, which can be highly problematic given that existing annotated glaucoma datasets contain at most few hundreds of samples. Furthermore, deep learning techniques are computationally greedy, which can be a problem in scenarios with limited resources. The objective of this work is to make optimal use of the few annotated data at disposal and obtain better generalization performance of glaucoma diagnosis deep learning models. We achieve this by designing and training a novel multi-task deep learning model that leverages the similarities of related eye-fundus tasks and measurements used in glaucoma diagnosis. The proposed multi-task deep learning model with independent optimizers (MTL-IO) simultaneously learns different segmentation and classification tasks, thus benefiting from their similarity. The framework uses a U-Net encoder-decoder convolutional network as backbone architecture. Our MTL-IO approach was trained in a retinal fundus glaucoma challenge dataset, including 1200 retinal fundus images (400 for training, 400 for validation, 400 for testing) from different cameras and medical centers. MTL-IO obtained a 92.61 ± 0.38 AUC performance compared to a 87.37 ± 1.51 obtained by the same backbone network trained to detect glaucoma, also outperforming two other state-of-the-art multi-task learning models. Our approach pairs with trained experts and uses ∼ 3.5 times fewer parameters than training each task separately. The data and the code for reproducing our results are publicly available.
更多查看译文
关键词
glaucoma detection,color fundus images,deep learning,multi-task
AI 理解论文
溯源树
样例
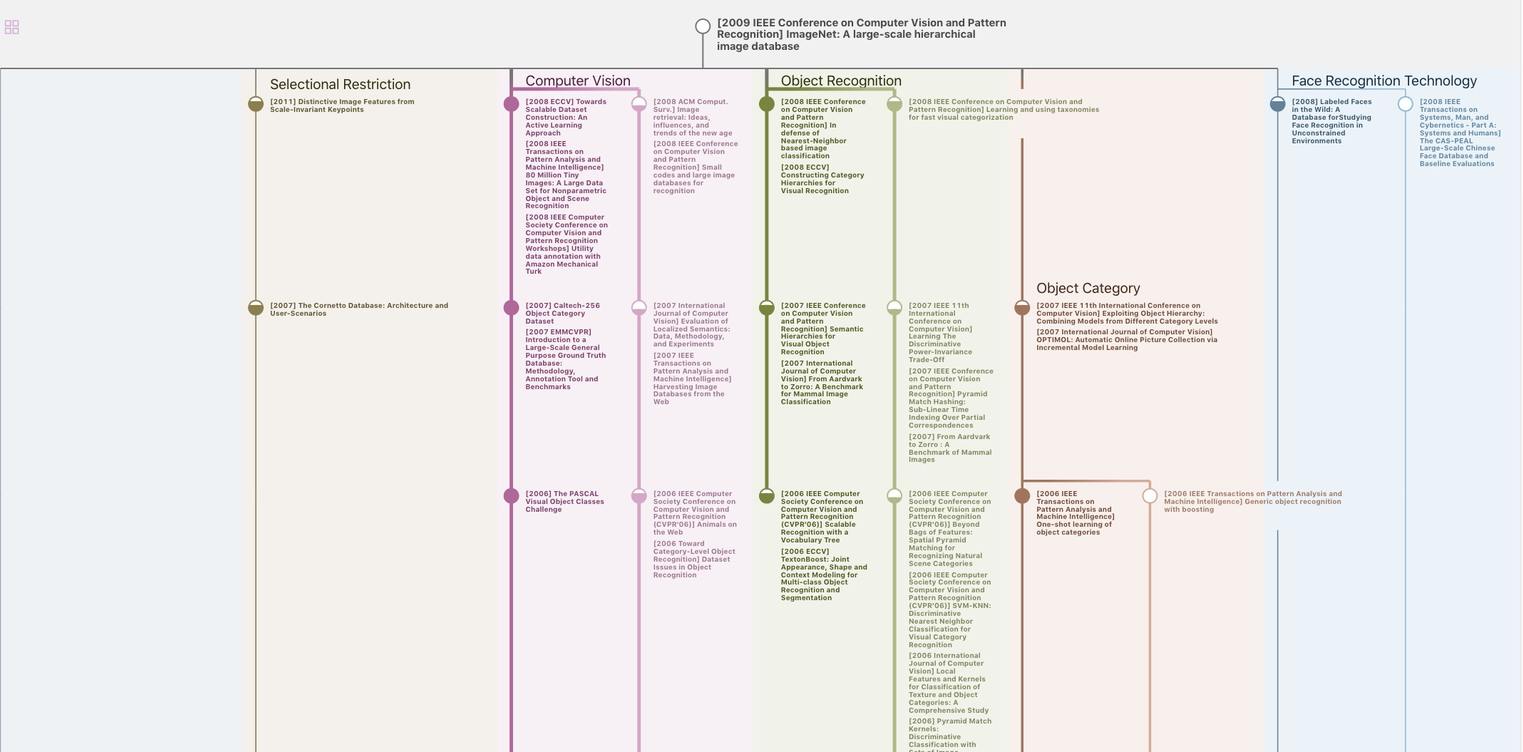
生成溯源树,研究论文发展脉络
Chat Paper
正在生成论文摘要