Personalization Improves Privacy-Accuracy Tradeoffs in Federated Optimization
arXiv (Cornell University)(2022)
摘要
Large-scale machine learning systems often involve data distributed across a collection of users. Federated optimization algorithms leverage this structure by communicating model updates to a central server, rather than entire datasets. In this paper, we study stochastic optimization algorithms for a personalized federated learning setting involving local and global models subject to user-level (joint) differential privacy. While learning a private global model induces a cost of privacy, local learning is perfectly private. We show that coordinating local learning with private centralized learning yields a generically useful and improved tradeoff between accuracy and privacy. We illustrate our theoretical results with experiments on synthetic and real-world datasets.
更多查看译文
关键词
federated
AI 理解论文
溯源树
样例
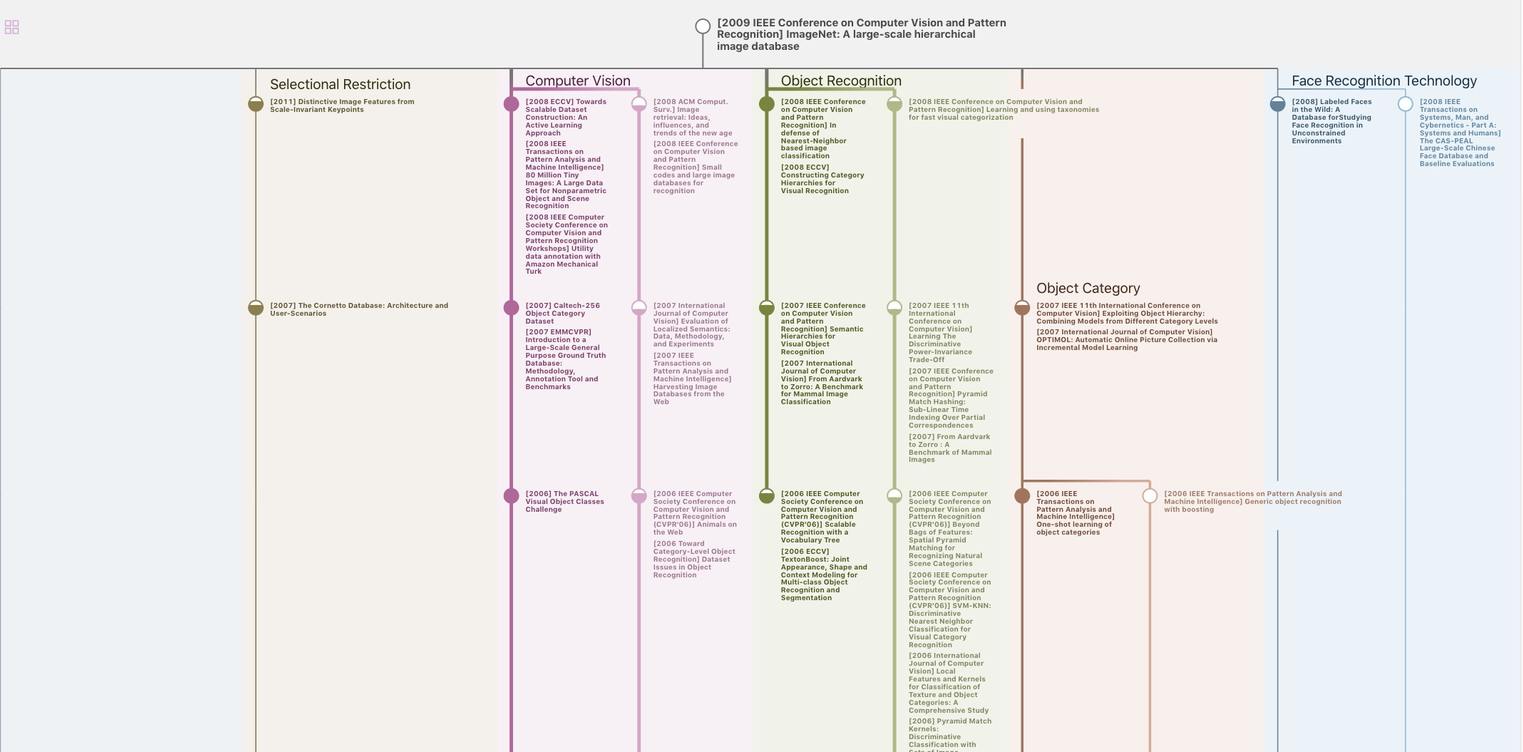
生成溯源树,研究论文发展脉络
Chat Paper
正在生成论文摘要