Towards a Post-clustering Test for Differential Expression.
Research in Computational Molecular Biology(2019)
摘要
Abstract Single-cell technologies have seen widespread adoption in recent years. The datasets generated by these technologies provide information on up to millions or more individual cells; however, the identities of the cells are often only determined computationally. Single-cell computational pipelines involve two critical steps: organizing the cells in a biologically meaningful way (clustering) and identifying the markers driving this organization (differential expression analysis). Because clustering algorithms force separation, performing differential expression analysis after clustering on the same dataset will generate artificially low p-values, potentially resulting in false discoveries. In this work, we introduce the truncated normal (TN) test, a test based on the truncated normal distribution that significantly corrects for this problem. We present a data-splitting-based framework that leverages the TN test to return reasonable p-values for arbitrary clustering schemes. We demonstrate the efficacy of our solution on simulated and real datasets, and we provide our code at https://github.com/jessemzhang/tn_test.
更多查看译文
关键词
differential expression,test,post-clustering
AI 理解论文
溯源树
样例
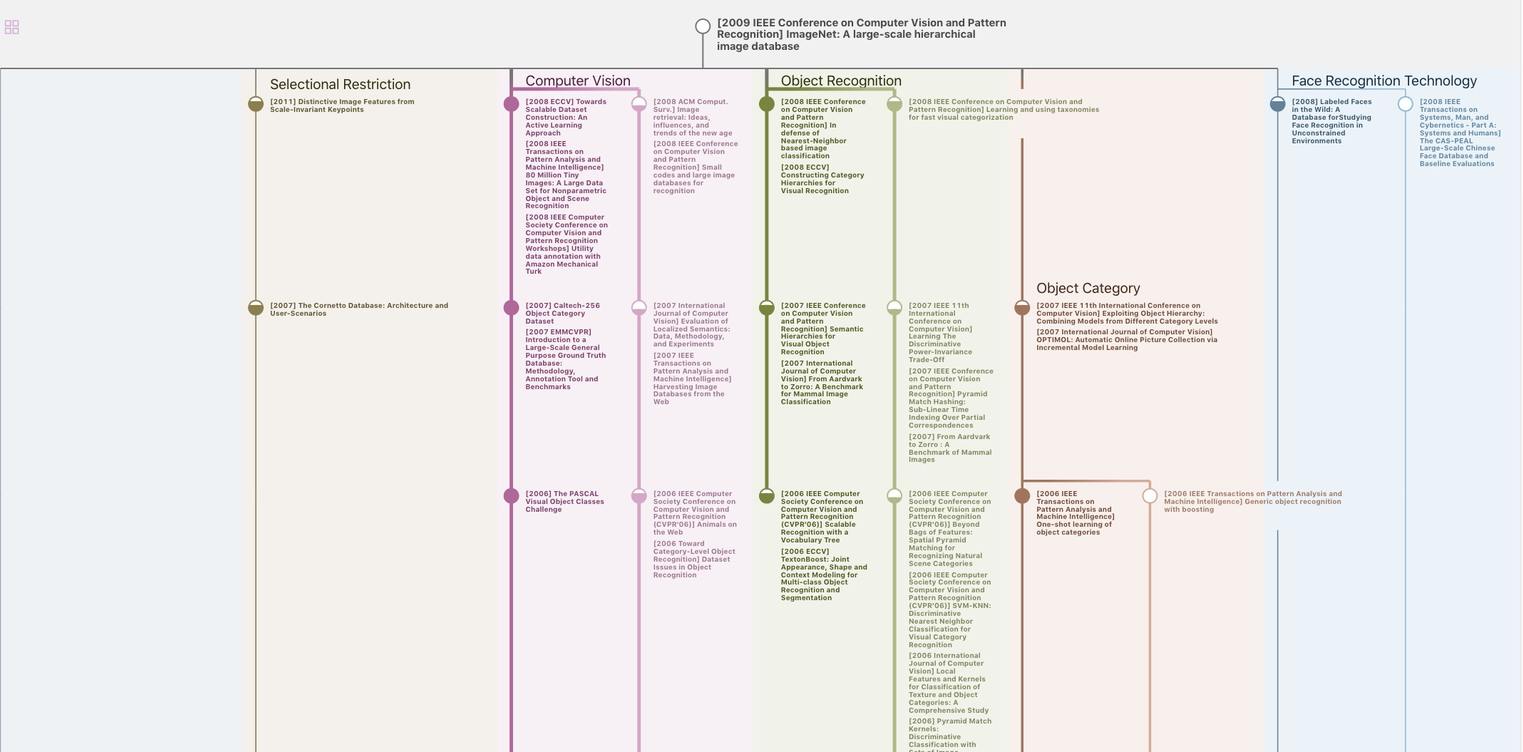
生成溯源树,研究论文发展脉络
Chat Paper
正在生成论文摘要