Training Context-Sensitive Neural Networks with Few Relevant Examples for the TREC-9 Routing.
Text REtrieval Conference(2000)
摘要
The present paper describes our second participation to the routing task; it features improvements over our previous approach [Stricker et al., 2000]. Our former model used a bag of for text representation with a feature selection, and a neural network without hidden neuron (i.e. a logistic regression), to estimate the probability of relevance of each document. This approach was close to the ones proposed by [Schutze et al., 1995] or [Wiener et al., 1995] but its original feature was the use of very few relevant features for text representation (25 features per topic on the average for the TREC-8 Routing). In this paper, two main improvements are proposed: • The feature selection defines target words for which vectors of local contexts are subsequently defined. These vectors help disambiguate the target words and are defined by an analysis of both the relevant and the irrelevant documents of the training set. • This new representation requires large neural networks, which are therefore prone to overfitting. A regularization technique is applied during training to favor smoother network mappings, thereby avoiding overfitting. This was achieved by adding a weight decay term to the usual cost function. This approach led to good results on the MeSH Sample topics (S2RNsamp) and on the OHSUMED topics (S2RNr1 and S2RNr2).
更多查看译文
关键词
networks,training,context-sensitive
AI 理解论文
溯源树
样例
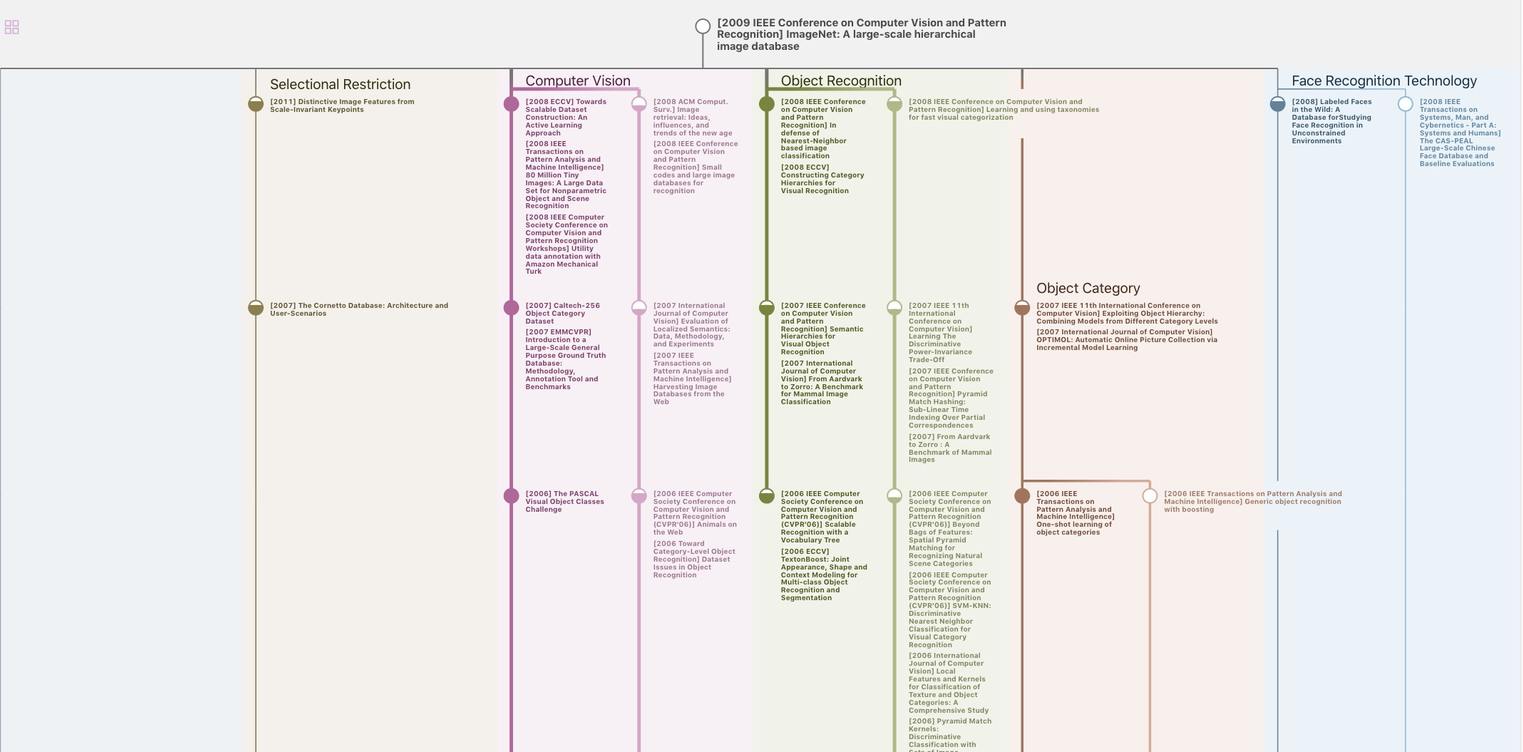
生成溯源树,研究论文发展脉络
Chat Paper
正在生成论文摘要