Linear Bandits on Uniformly Convex Sets.
Journal of Machine Learning Research(2021)
摘要
Linear bandit algorithms yield O~(n√T) pseudo-regret bounds on compact convex action sets K⊂Rn and two types of structural assumptions lead to better pseudo-regret bounds. When K is the simplex or an lp ball with p∈]1,2], there exist bandits algorithms with O~(√n√T) pseudo-regret bounds. Here, we derive bandit algorithms for some strongly convex sets beyond lp balls that enjoy pseudo-regret bounds of O~(√n√T), which answers an open question from [BCB12, §5.5.]. Interestingly, when the action set is uniformly convex but not necessarily strongly convex, we obtain pseudo-regret bounds with a dimension dependency smaller than O(√n). However, this comes at the expense of asymptotic rates in T varying between O(√T) and O(T).
更多查看译文
关键词
uniformly convex sets
AI 理解论文
溯源树
样例
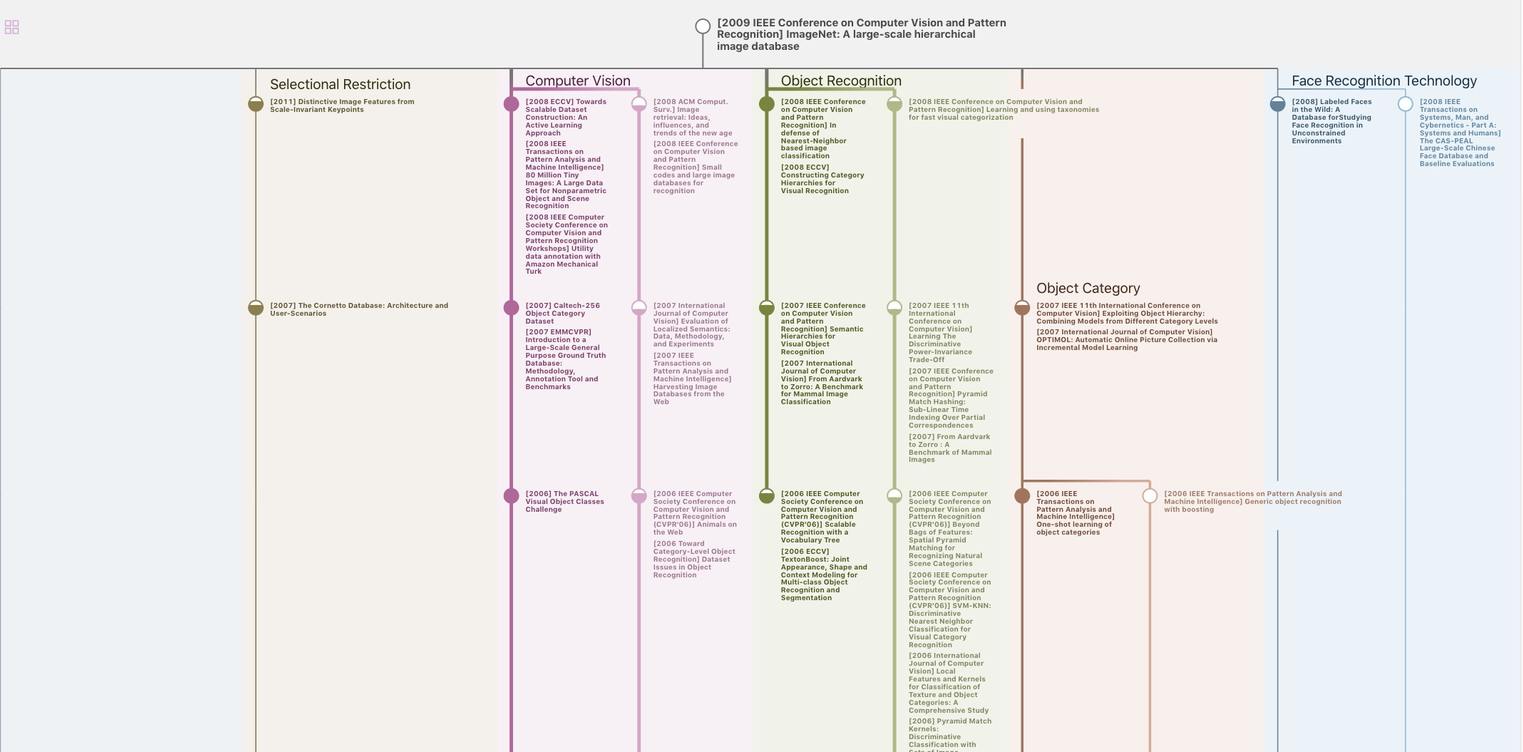
生成溯源树,研究论文发展脉络
Chat Paper
正在生成论文摘要