LSB: Local Self-Balancing MCMC in Discrete Spaces.
arXiv (Cornell University)(2021)
摘要
Markov Chain Monte Carlo (MCMC) methods are promising solutions to sample from target distributions in high dimensions. While MCMC methods enjoy nice theoretical properties, like guaranteed convergence and mixing to the true target, in practice their sampling efficiency depends on the choice of the proposal distribution and the target at hand. This work considers using machine learning to adapt the proposal distribution to the target, in order to improve the sampling efficiency in the purely discrete domain. Specifically, (i) it proposes a new parametrization for a family of proposal distributions, called locally balanced proposals, (ii) it defines an objective function based on mutual information and (iii) it devises a learning procedure to adapt the parameters of the proposal to the target, thus achieving fast convergence and fast mixing. We call the resulting sampler as the Locally Self-Balancing Sampler (LSB). We show through experimental analysis on the Ising model and Bayesian networks that LSB is indeed able to improve the efficiency over a state-of-the-art sampler based on locally balanced proposals, thus reducing the number of iterations required to converge, while achieving comparable mixing performance.
更多查看译文
关键词
discrete spaces,self-balancing
AI 理解论文
溯源树
样例
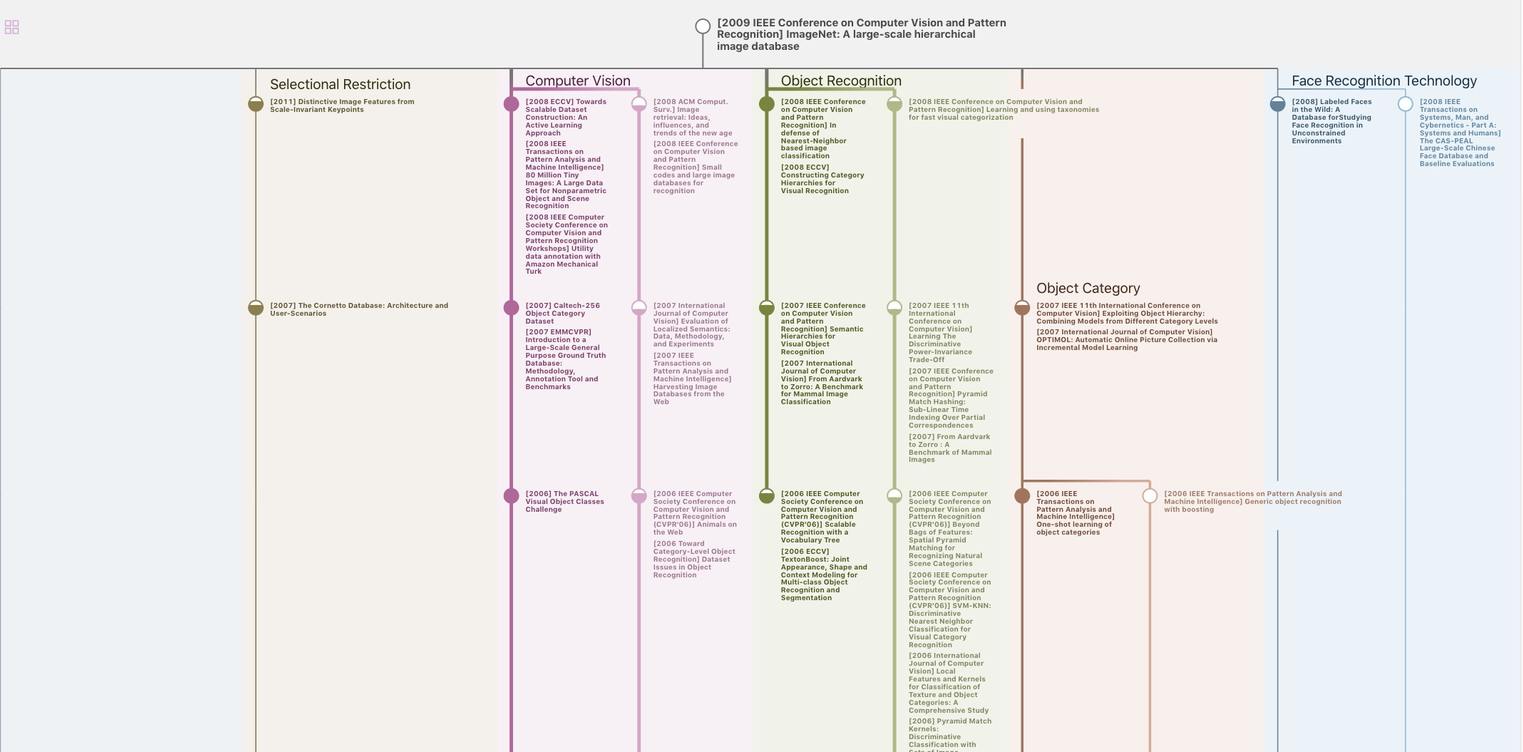
生成溯源树,研究论文发展脉络
Chat Paper
正在生成论文摘要