Multivariable times series classification through an interpretable representation
arXiv (Cornell University)(2020)
摘要
Multivariate time series classification is a task with increasing importance due to the proliferation of new problems in various fields (economy, health, energy, transport, crops, etc.) where a large number of information sources are available. Direct extrapolation of methods that traditionally worked in univariate environments cannot frequently be applied to obtain the best results in multivariate problems. This is mainly due to the inability of these methods to capture the relationships between the different variables that conform a multivariate time series. The multivariate proposals published to date offer competitive results but are hard to interpret. In this paper we propose a time series classification method that considers an alternative representation of time series through a set of descriptive features taking into account the relationships between the different variables of a multivariate time series. We have applied traditional classification algorithms obtaining interpretable and competitive results.
更多查看译文
关键词
multivariable times series classification,representation
AI 理解论文
溯源树
样例
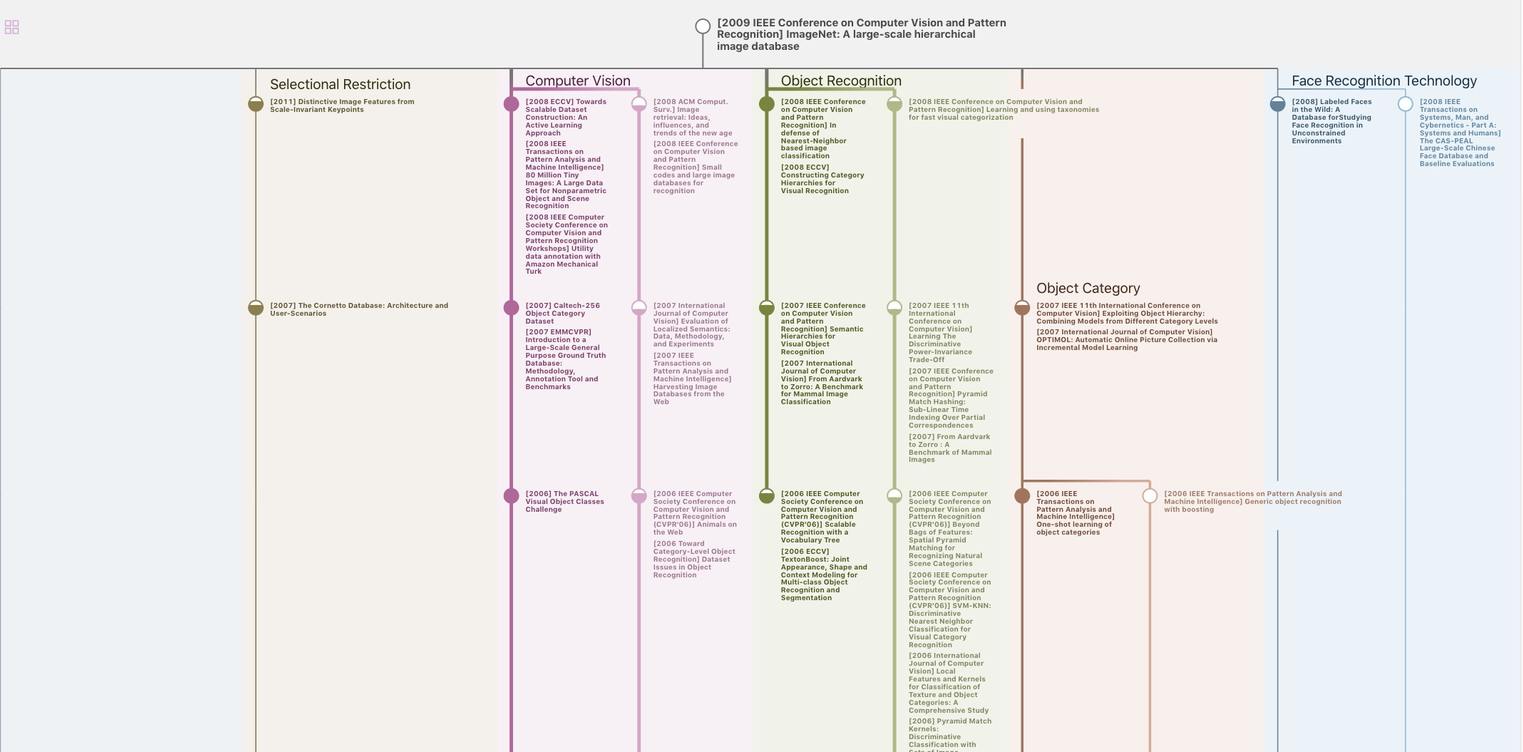
生成溯源树,研究论文发展脉络
Chat Paper
正在生成论文摘要