ProtTrans: Towards Cracking the Language of Life's Code Through Self-Supervised Deep Learning and High Performance Computing.
arXiv (Cornell University)(2021)
摘要
Currently, a growing number of mature natural language processing applications make people's life more convenient. Such applications are built by source code - the language in software engineering. However, the applications for understanding source code language to ease the software engineering process are under-researched. Simultaneously, the transformer model, especially its combination with transfer learning, has been proven to be a powerful technique for natural language processing tasks. These breakthroughs point out a promising direction for process source code and crack software engineering tasks. This paper describes CodeTrans - an encoder-decoder transformer model for tasks in the software engineering domain, that explores the effectiveness of encoder-decoder transformer models for six software engineering tasks, including thirteen sub-tasks. Moreover, we have investigated the effect of different training strategies, including single-task learning, transfer learning, multi-task learning, and multi-task learning with fine-tuning. CodeTrans outperforms the state-of-the-art models on all the tasks. To expedite future works in the software engineering domain, we have published our pre-trained models of CodeTrans. this https URL
更多查看译文
关键词
code,language,deep learning,high performance computing,self-supervised
AI 理解论文
溯源树
样例
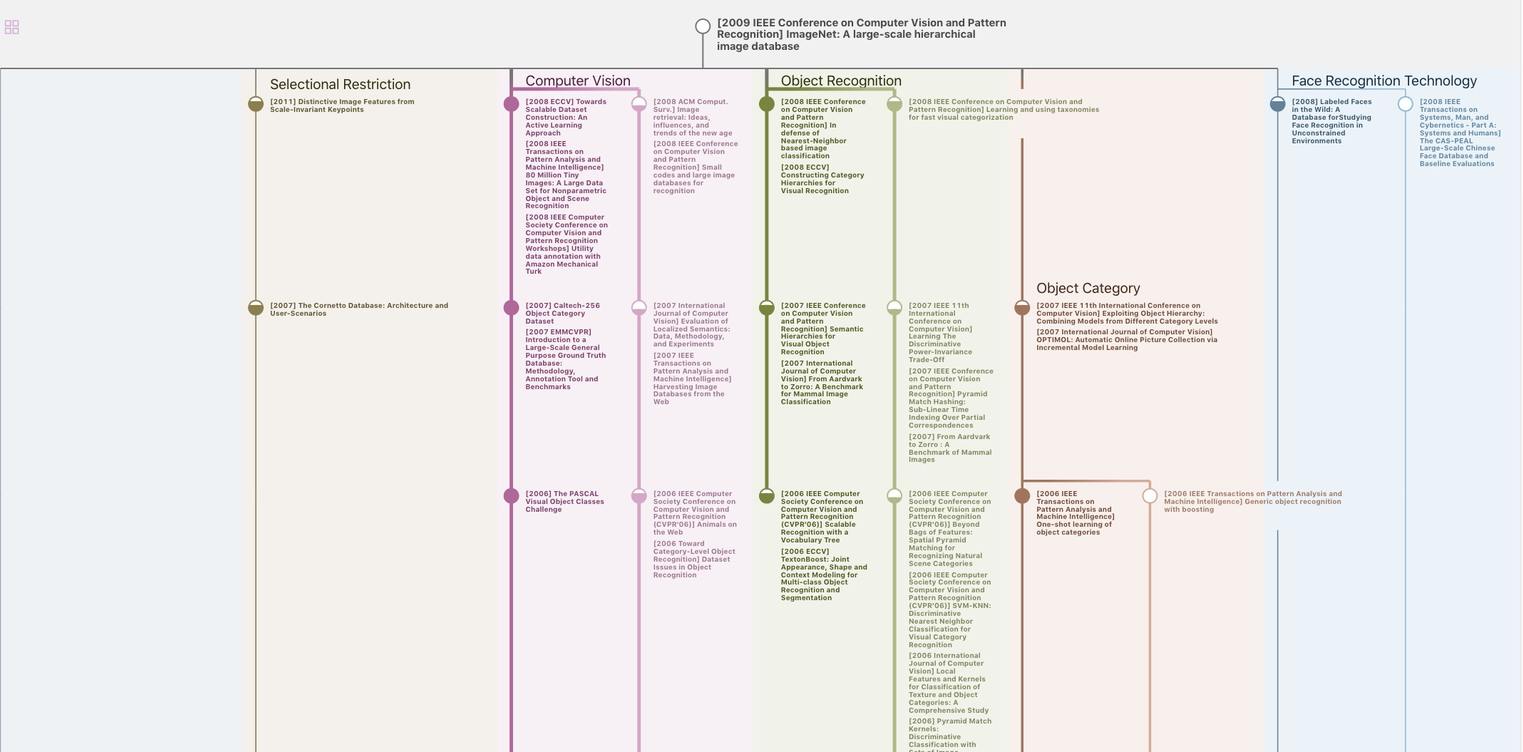
生成溯源树,研究论文发展脉络
Chat Paper
正在生成论文摘要