Guided Sampling by Neighborhood Information and Matching Scores for Multi-Structure Data
IEEE SIGNAL PROCESSING LETTERS(2023)
摘要
The success of most robust model estimation methods heavily relies on their used data sampling algorithms. This letter proposes a novel sampling algorithm, called Guided Sampling by Neighborhood Information and Matching Scores (NIMS), to efficiently sample promising hypotheses for fitting multi-structure data. Specifically, NIMS follows a specific sampling process. First, the proposed NIMS randomly selects a data point. Then, NIMS selects the neighbors of the selected data by using the neighborhood information to remove most of the outlier neighbors. Finally, NIMS samples a data subset using matching scores from the selected neighbors, which encourages NIMS to sample inliers from the selected neighbors. Experimental results on the publicly available AdelaideRMF dataset demonstrate that the proposed NIMS outperforms several state-of-the-art sampling algorithms.
更多查看译文
关键词
Matching scores,multi-structure data,neighborhood information,robust model estimation
AI 理解论文
溯源树
样例
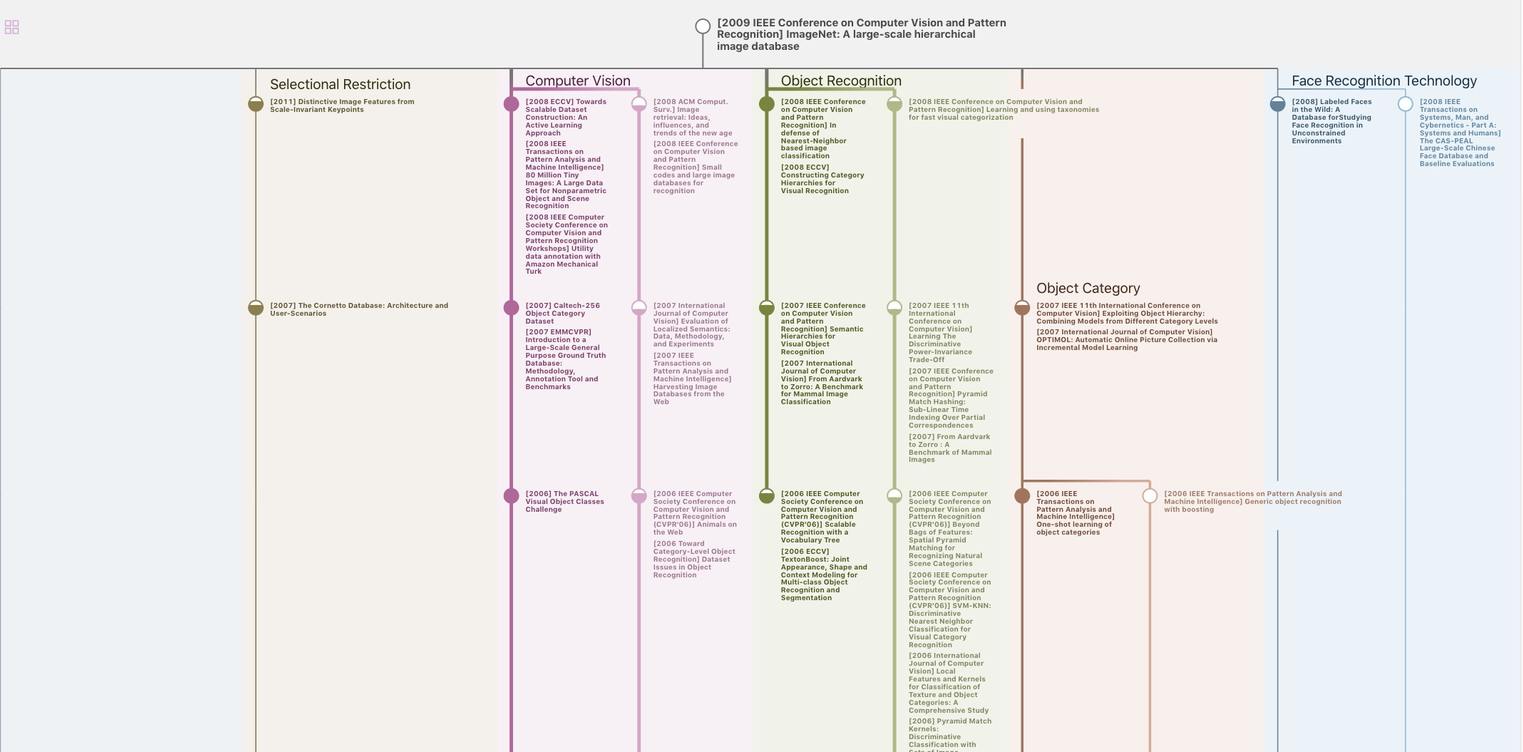
生成溯源树,研究论文发展脉络
Chat Paper
正在生成论文摘要