Robust and Generalized Physical Adversarial Attacks via Meta-GAN
IEEE TRANSACTIONS ON INFORMATION FORENSICS AND SECURITY(2024)
摘要
Deep neural networks are known to be vulnerable to adversarial examples, where adding carefully crafted adversarial perturbations to the inputs can mislead the DNN model. However, it is challenging to generate effective adversarial examples in the physical world due to many uncontrollable physical dynamics, which pose security and safety threats in the real world. Current physical attack methods aim to generate robust physical adversarial examples by simulating all possible physical dynamics. If attacking a new image or a new DNN model, they require expensive manual efforts for simulating physical dynamics or considerable time for iteratively optimizing. To tackle these limitations, we propose a robust and generalized physical adversarial attack method with Meta-GAN (Meta-GAN Attack), which is able to not only generate robust physical adversarial examples, but also generalize to attacking novel images and novel DNN models by accessing a few digital and physical images. First, we propose to craft robust physical adversarial examples with a generative attack model via simulating color and shape distortions. Second, we formulate the physical attack as a few-shot learning problem and design a novel class-agnostic and model-agnostic meta-learning algorithm to solve this problem. Extensive experiments on two benchmark datasets with four challenging experimental settings verify the superior robustness and generalization of our method by comparing to state-of-the-art physical attack methods. The source code is released at github.
更多查看译文
关键词
Adversarial attacks,physical attacks,generative adversarial networks,meta-learning
AI 理解论文
溯源树
样例
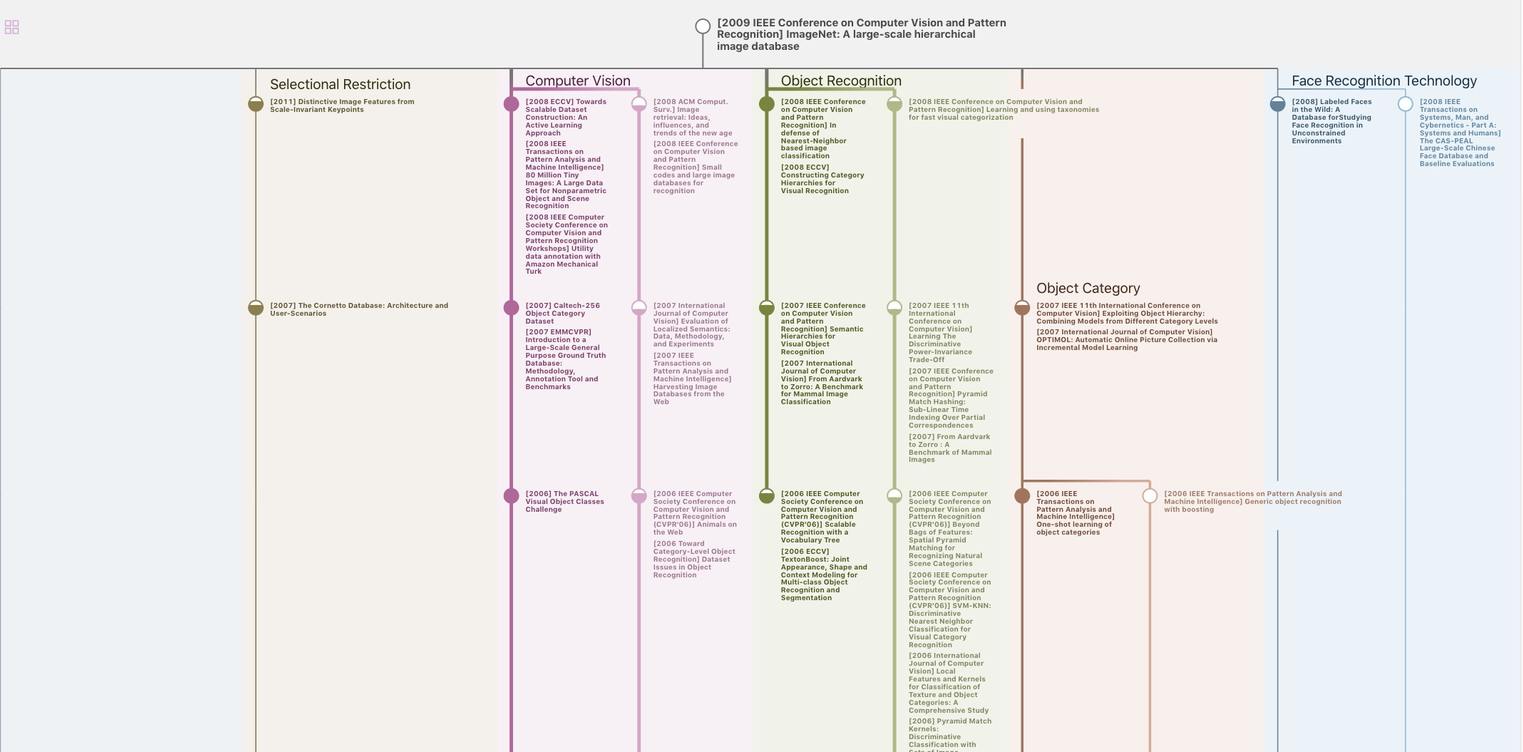
生成溯源树,研究论文发展脉络
Chat Paper
正在生成论文摘要