Pixel-wise Wildfire Burn Severity Classification with Bi-temporal Sentinel-2 Data and Deep Learning.
ICBDT '23: Proceedings of the 2023 6th International Conference on Big Data Technologies(2023)
摘要
Wildfires result in extensive damage and pose significant threats to the natural environment and human society. Accurate and timely assessment of wildfire damage is critical for effective post-fire management and restoration efforts. Much progress has been made for monitoring and mapping burned areas based on manual feature extraction and machine learning. However, current approaches are often hindered by several limitations, including poor generalizability, complex and time-consuming procedures, and a primary focus on binary classification, only distinguishing between burned and non-burned areas. As such, this paper intends to develop an advanced framework that not only can reliably detect burned areas, but also has the capability to assess the burn severity (low, medium and high) for the detected areas. Within this framework, pixel-wise multiclass models for detecting wildfire burn severity level are developed based on the standard U-Net architecture using pre- and post-fire Sentinel-2 remote sensing images. The experimental results demonstrated the power of the Bi-temporal U-Net model for both multi-class and binary mapping of wildfire detection, achieving an overall accuracy (OA) of over 95%, and outperformed baseline algorithms, including fully convolutional network, random forest, eXtreme gradient boosting, and support vector machine, with an average improvement of 18% in F1-score and 15% in mean intersection over union.
更多查看译文
AI 理解论文
溯源树
样例
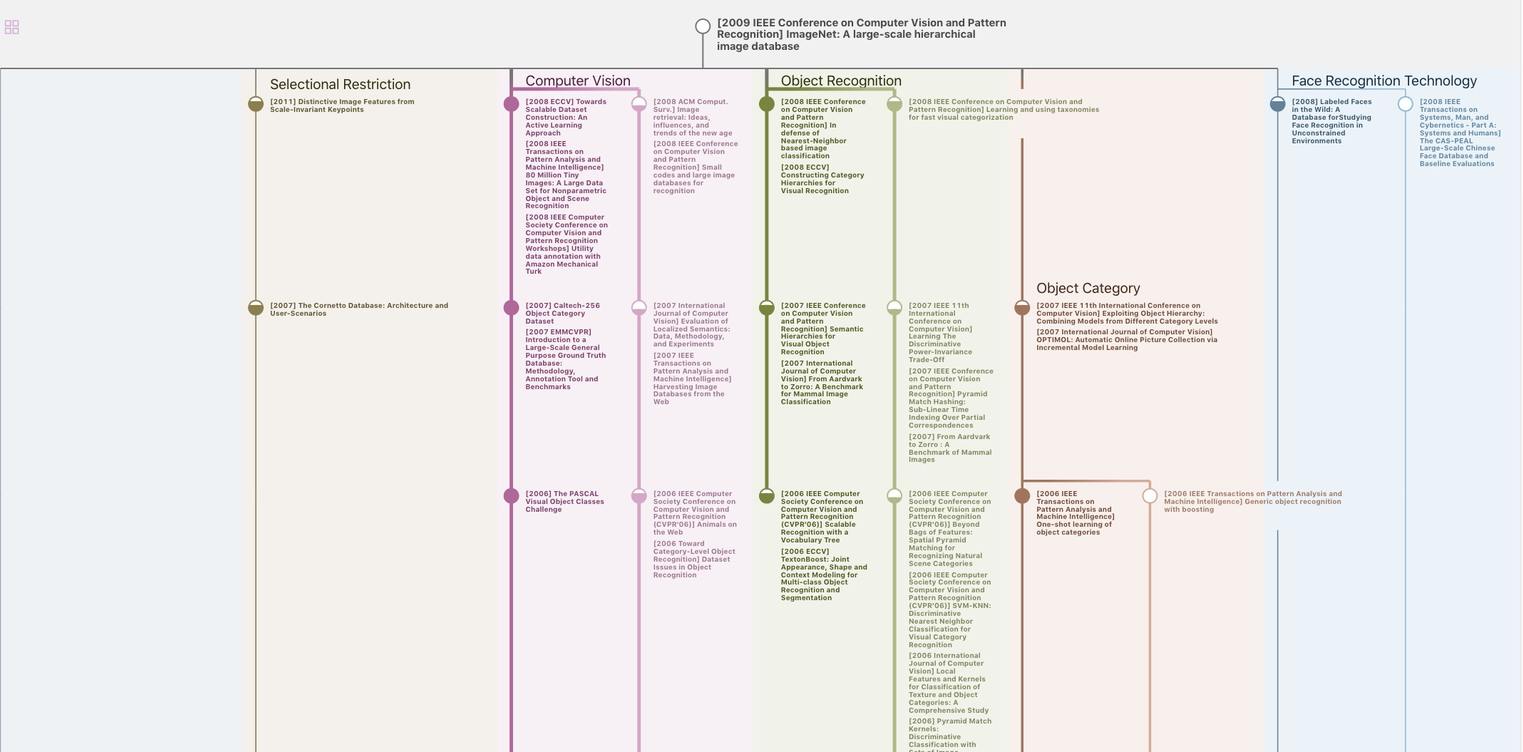
生成溯源树,研究论文发展脉络
Chat Paper
正在生成论文摘要