Efficient Text-to-Code Retrieval with Cascaded Fast and Slow Transformer Models
PROCEEDINGS OF THE 31ST ACM JOINT MEETING EUROPEAN SOFTWARE ENGINEERING CONFERENCE AND SYMPOSIUM ON THE FOUNDATIONS OF SOFTWARE ENGINEERING, ESEC/FSE 2023(2023)
摘要
The goal of semantic code search or text-to-code search is to retrieve a semantically relevant code snippet from an existing code database using a natural language query. When constructing a practical semantic code search system, existing approaches fail to provide an optimal balance between retrieval speed and the relevance of the retrieved results. We propose an efficient and effective text-to-code search framework with cascaded fast and slow models, in which a fast transformer encoder model is learned to optimize a scalable index for fast retrieval followed by learning a slow classification-based re-ranking model to improve the accuracy of the top K results from the fast retrieval. To further reduce the high memory cost of deploying two separate models in practice, we propose to jointly train the fast and slow model based on a single transformer encoder with shared parameters. Empirically our cascaded method is not only efficient and scalable, but also achieves state-of-the-art results with an average mean reciprocal ranking (MRR) score of 0.7795 (across 6 programming languages) on the CodeSearchNet benchmark as opposed to the prior state-of-the-art result of 0.744 MRR. Our codebase can be found at this link.
更多查看译文
关键词
Developer Productivity,Code retrieval,Text to Code Search,Transformer models,Cascaded retrieval schemes,top K retrieval
AI 理解论文
溯源树
样例
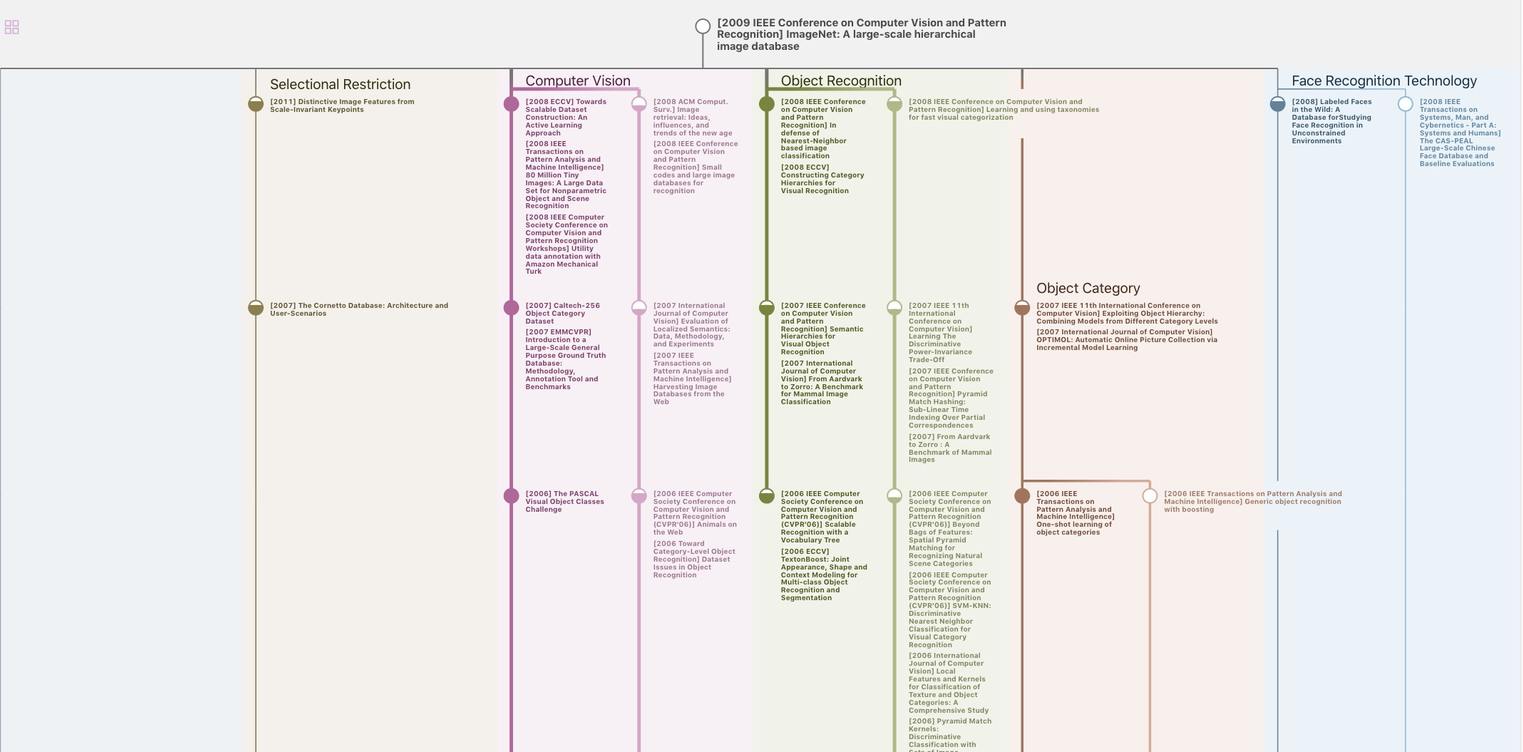
生成溯源树,研究论文发展脉络
Chat Paper
正在生成论文摘要