Optimizing Honeypot Placement Strategies with Graph Neural Networks for Enhanced Resilience via Cyber Deception.
GNNet '23: Proceedings of the 2nd on Graph Neural Networking Workshop 2023(2023)
摘要
In the ever-evolving realm of cybersecurity, strategic honeypot placement is pivotal for enhanced network deception. This paper introduces a novel approach, leveraging Graph Neural Networks (GNNs), to optimize honeypot placements, outstripping traditional game-theoretic methods. Traditional techniques often face computational inefficiencies due to the "curse of dimensionality" in complex dynamic environments. Our GNN model, through extensive exploration of architectures including Graph Attention Transformers (GAT) and Graph Transformer models, showcases superior performance. With the integration of game-theoretic edge features, the model achieves a remarkable test accuracy of 92.34%. Additionally, our GNN solution provides a 139x inference speedup over classical methods, underpinning its efficiency and potential to revolutionize cybersecurity strategies.
更多查看译文
AI 理解论文
溯源树
样例
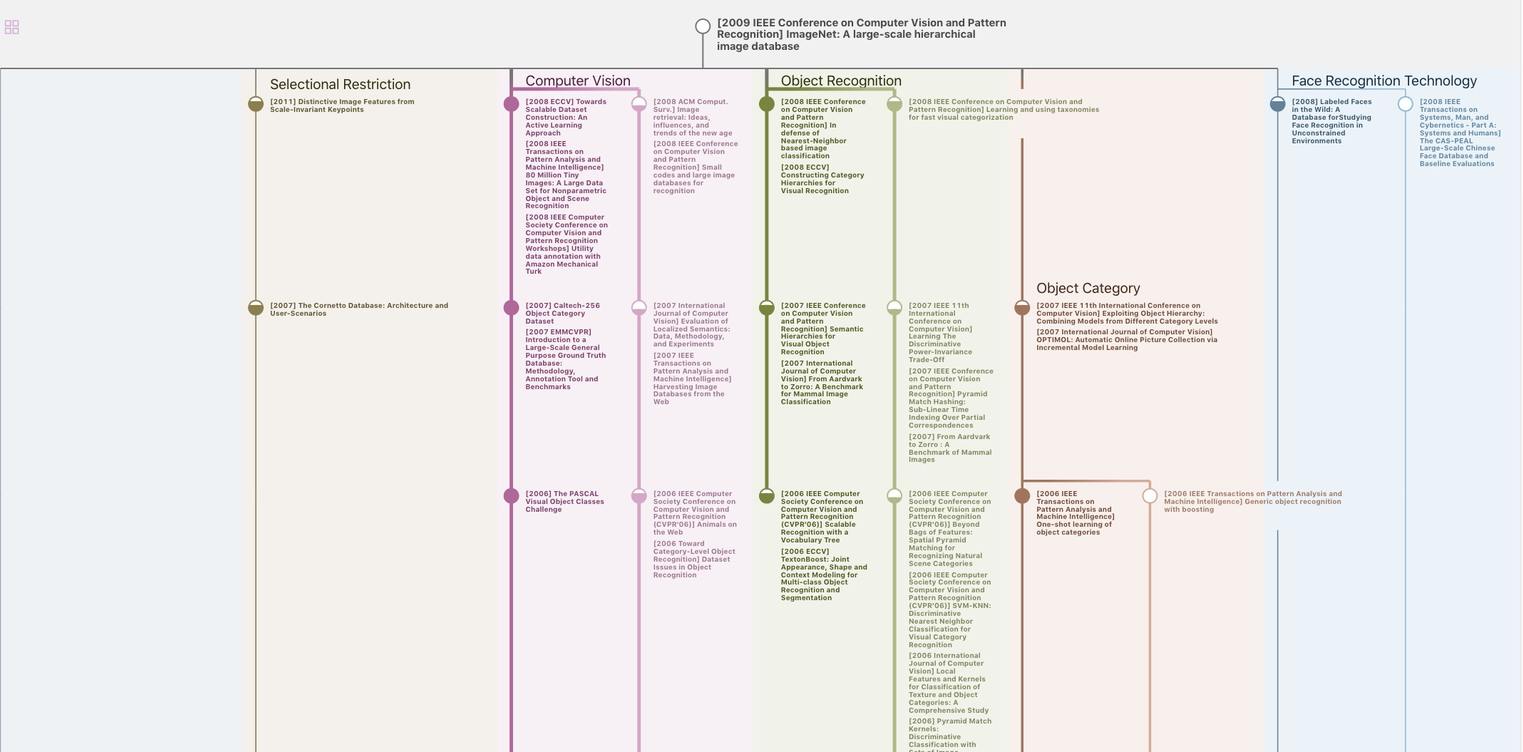
生成溯源树,研究论文发展脉络
Chat Paper
正在生成论文摘要