Feature Enhancement and Alignment for Oriented Object Detection
IEEE JOURNAL OF SELECTED TOPICS IN APPLIED EARTH OBSERVATIONS AND REMOTE SENSING(2024)
摘要
Over the last few years, developments in fields such as aviation and remote sensing have drawn increasing attention to the detection of rotated objects. Unlike general object detection, rotated object detection requires overcoming certain challenges, such as detecting objects with different directions and high aspect ratios. Recently proposed rotated object detectors have achieved good results, but most of them rely on hand-designed anchors, which require manual adjustment of the anchors settings in different scenarios. On the contrary, this article presents a method called FEADet, an anchor-free detector that utilizes feature enhancement and alignment to achieve competitive performance, without the use of anchors. Specifically, in order to better fuse features across different layers, we design an attention feature fusion (AAF) module to reduce the aliasing effect produced by the fusion of different layers. To deal with feature misalignment in detecting objects with orientation, we propose an adaptive alignconv (AAC) module, which is implemented by the constrained deformable convolution and align convolution. The ACC module can efficiently extract object features according to the decoded boxes and predicted constrained offsets. On the two benchmark datasets, dataset for object detection in aerial images (DOTA) and high resolution ship collection 2016 (HRSC2016), a comprehensive evaluation of our method has been conducted to demonstrate the effectiveness of these method in comparison with state-of-the-art methods.
更多查看译文
关键词
Deep learning,feature alignment,feature enhancement,oriented object detection,remote sensing images
AI 理解论文
溯源树
样例
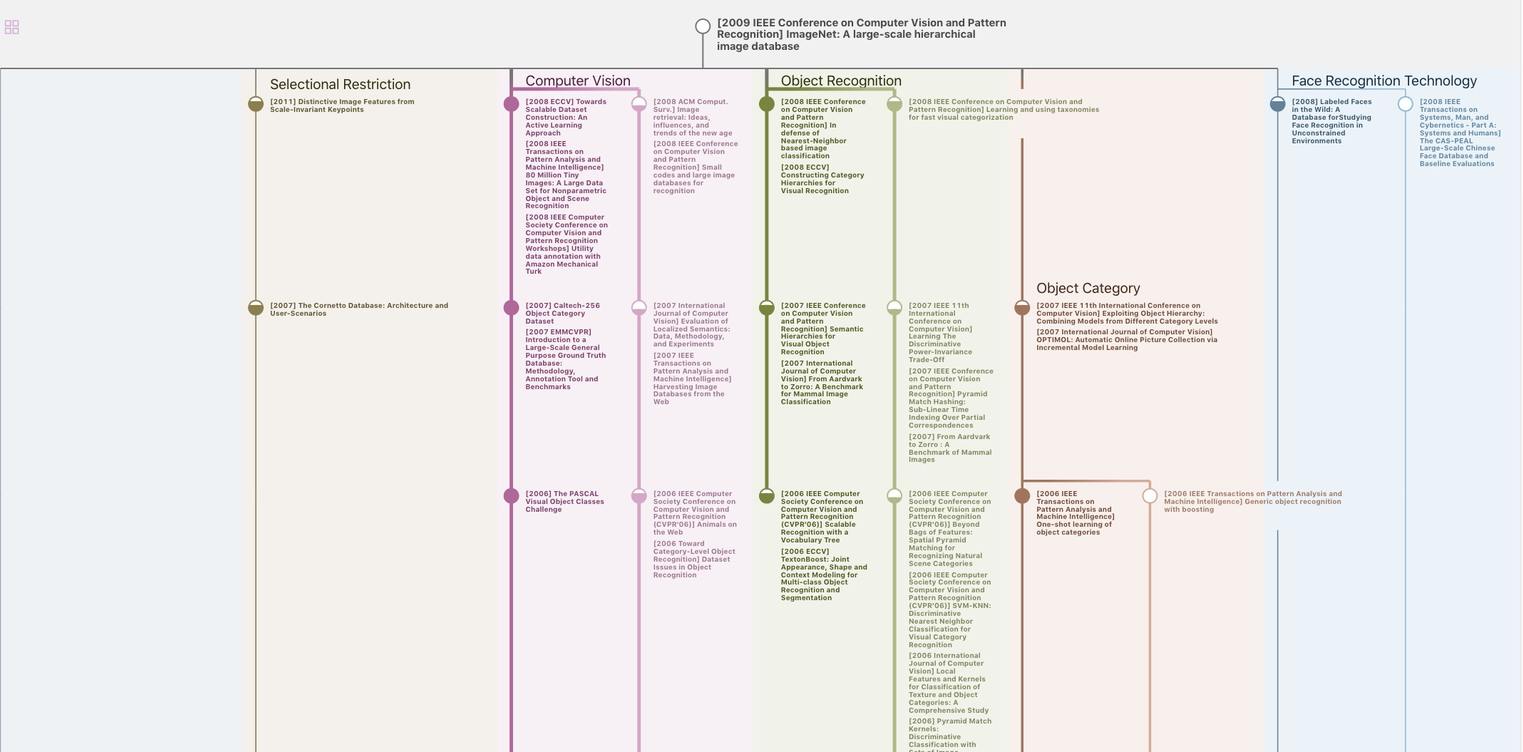
生成溯源树,研究论文发展脉络
Chat Paper
正在生成论文摘要