Unsupervised Approach to Evaluate Sentence-Level Fluency: Do We Really Need Reference?
CoRR(2023)
摘要
Fluency is a crucial goal of all Natural Language Generation (NLG) systems. Widely used automatic evaluation metrics fall short in capturing the fluency of machine-generated text. Assessing the fluency of NLG systems poses a challenge since these models are not limited to simply reusing words from the input but may also generate abstractions. Existing reference-based fluency evaluations, such as word overlap measures, often exhibit weak correlations with human judgments. This paper adapts an existing unsupervised technique for measuring text fluency without the need for any reference. Our approach leverages various word embeddings and trains language models using Recurrent Neural Network (RNN) architectures. We also experiment with other available multilingual Language Models (LMs). To assess the performance of the models, we conduct a comparative analysis across 10 Indic languages, correlating the obtained fluency scores with human judgments. Our code and human-annotated benchmark test-set for fluency is available at https://github.com/AnanyaCoder/TextFluencyForIndicLanaguges.
更多查看译文
AI 理解论文
溯源树
样例
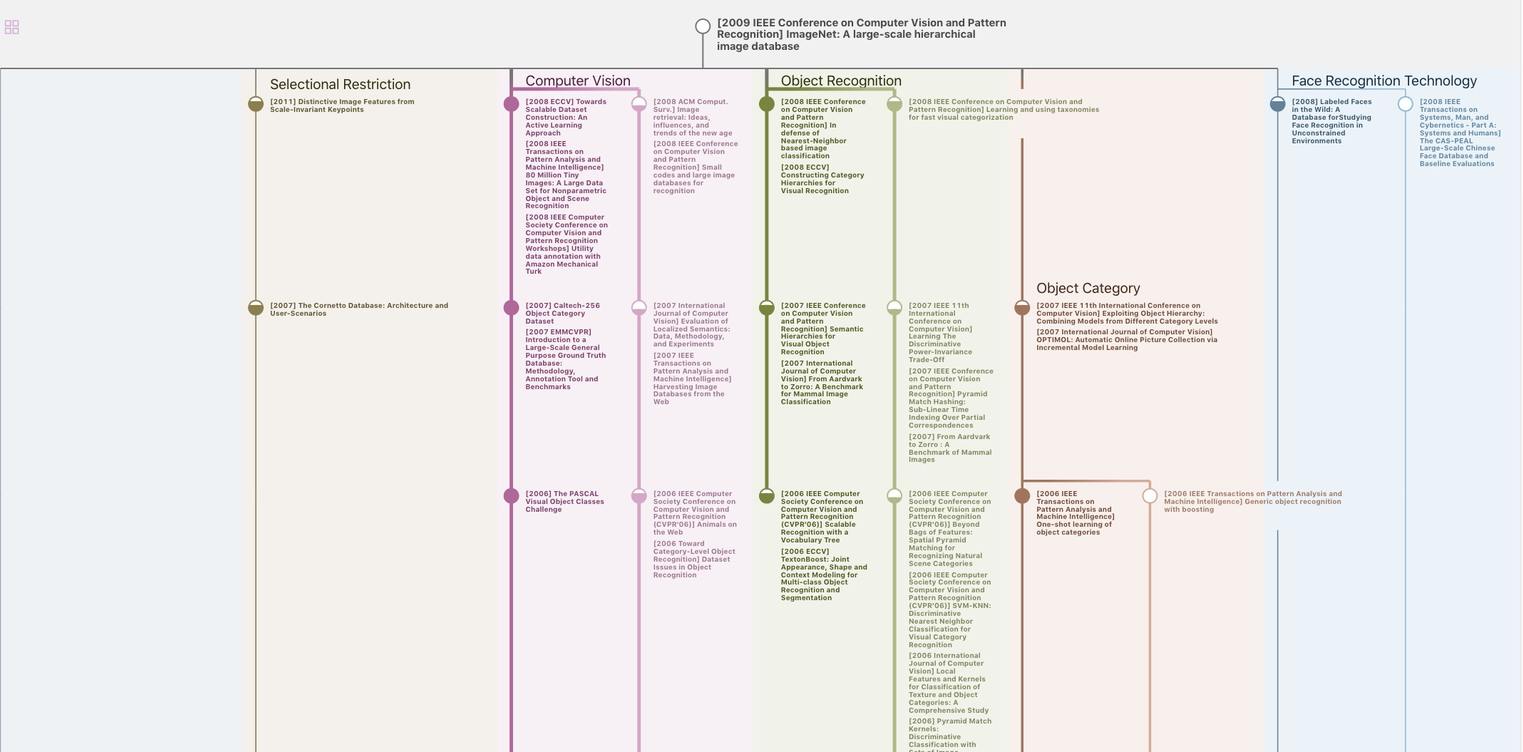
生成溯源树,研究论文发展脉络
Chat Paper
正在生成论文摘要