From Voices to Validity: Leveraging Large Language Models (LLMs) for Textual Analysis of Policy Stakeholder Interviews
CoRR(2023)
摘要
Obtaining stakeholders' diverse experiences and opinions about current policy
in a timely manner is crucial for policymakers to identify strengths and gaps
in resource allocation, thereby supporting effective policy design and
implementation. However, manually coding even moderately sized interview texts
or open-ended survey responses from stakeholders can often be labor-intensive
and time-consuming. This study explores the integration of Large Language
Models (LLMs)--like GPT-4--with human expertise to enhance text analysis of
stakeholder interviews regarding K-12 education policy within one U.S. state.
Employing a mixed-methods approach, human experts developed a codebook and
coding processes as informed by domain knowledge and unsupervised topic
modeling results. They then designed prompts to guide GPT-4 analysis and
iteratively evaluate different prompts' performances. This combined
human-computer method enabled nuanced thematic and sentiment analysis. Results
reveal that while GPT-4 thematic coding aligned with human coding by 77.89% at
specific themes, expanding to broader themes increased congruence to 96.02%,
surpassing traditional Natural Language Processing (NLP) methods by over 25%.
Additionally, GPT-4 is more closely matched to expert sentiment analysis than
lexicon-based methods. Findings from quantitative measures and qualitative
reviews underscore the complementary roles of human domain expertise and
automated analysis as LLMs offer new perspectives and coding consistency. The
human-computer interactive approach enhances efficiency, validity, and
interpretability of educational policy research.
更多查看译文
AI 理解论文
溯源树
样例
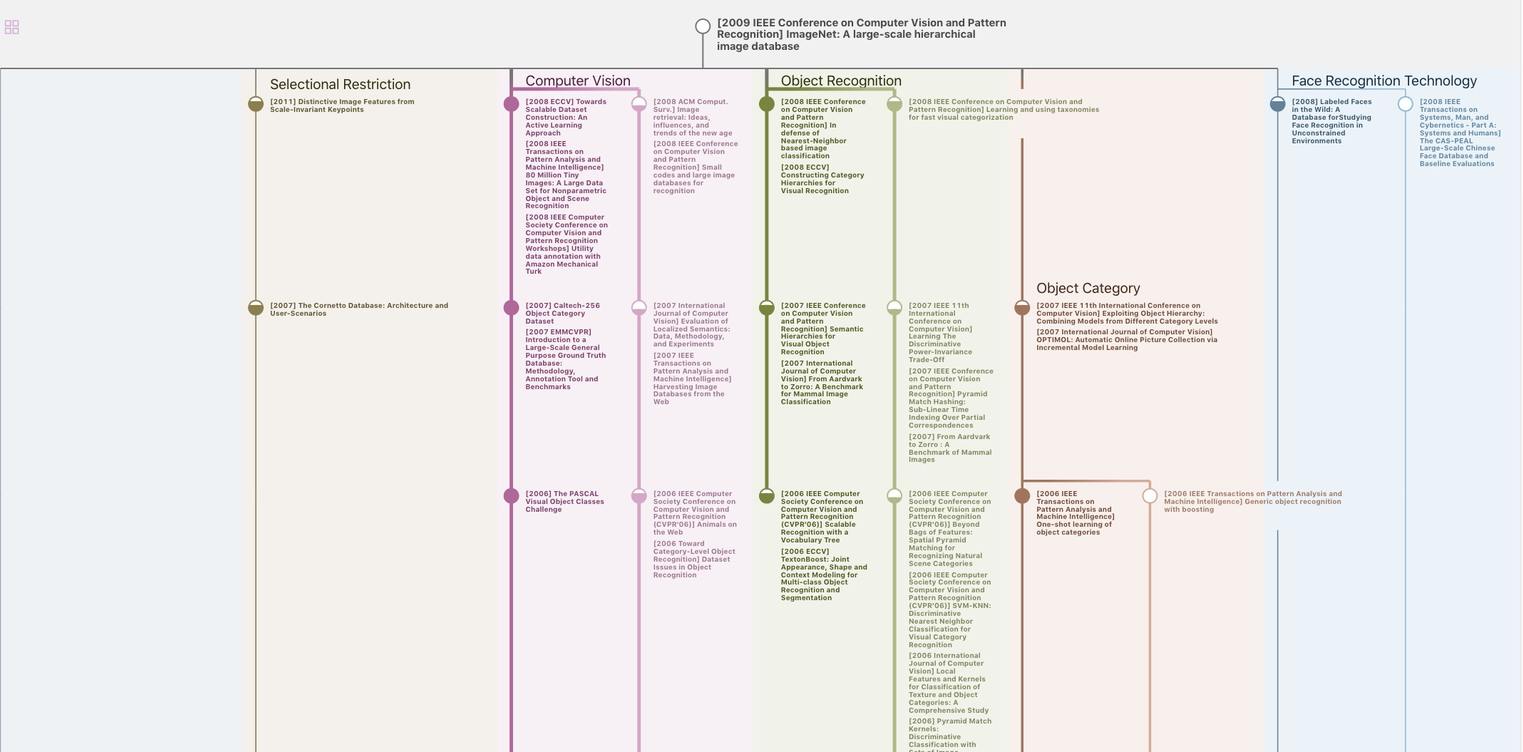
生成溯源树,研究论文发展脉络
Chat Paper
正在生成论文摘要