Fast and Robust Sparsity-Aware Block Diagonal Representation
IEEE TRANSACTIONS ON SIGNAL PROCESSING(2024)
摘要
The block diagonal structure of an affinity matrix is a commonly desired property in cluster analysis because it represents clusters of feature vectors by non-zero coefficients that are concentrated in blocks. However, recovering a block diagonal affinity matrix is challenging in real-world applications, in which the data may be subject to outliers and heavy-tailed noise that obscure the hidden cluster structure. To address this issue, we first analyze the effect of different fundamental outlier types in graph-based cluster analysis. A key idea that simplifies the analysis is to introduce a vector that represents a block diagonal matrix as a piece-wise linear function of the similarity coefficients that form the affinity matrix. We reformulate the problem as a robust piece-wise linear fitting problem and propose a Fast and Robust Sparsity-Aware Block Diagonal Representation (FRS-BDR) method, which jointly estimates cluster memberships and the number of blocks. Comprehensive experiments on a variety of real-world applications demonstrate the effectiveness of FRS-BDR in terms of clustering accuracy, robustness against corrupted features, computation time and cluster enumeration performance.
更多查看译文
关键词
Eigenvalues and eigenfunctions,Sparse matrices,Laplace equations,Symmetric matrices,Indexes,Robustness,Data models,Block diagonal representation,affinity matrix,similarity matrix,eigenvalues,subspace clustering
AI 理解论文
溯源树
样例
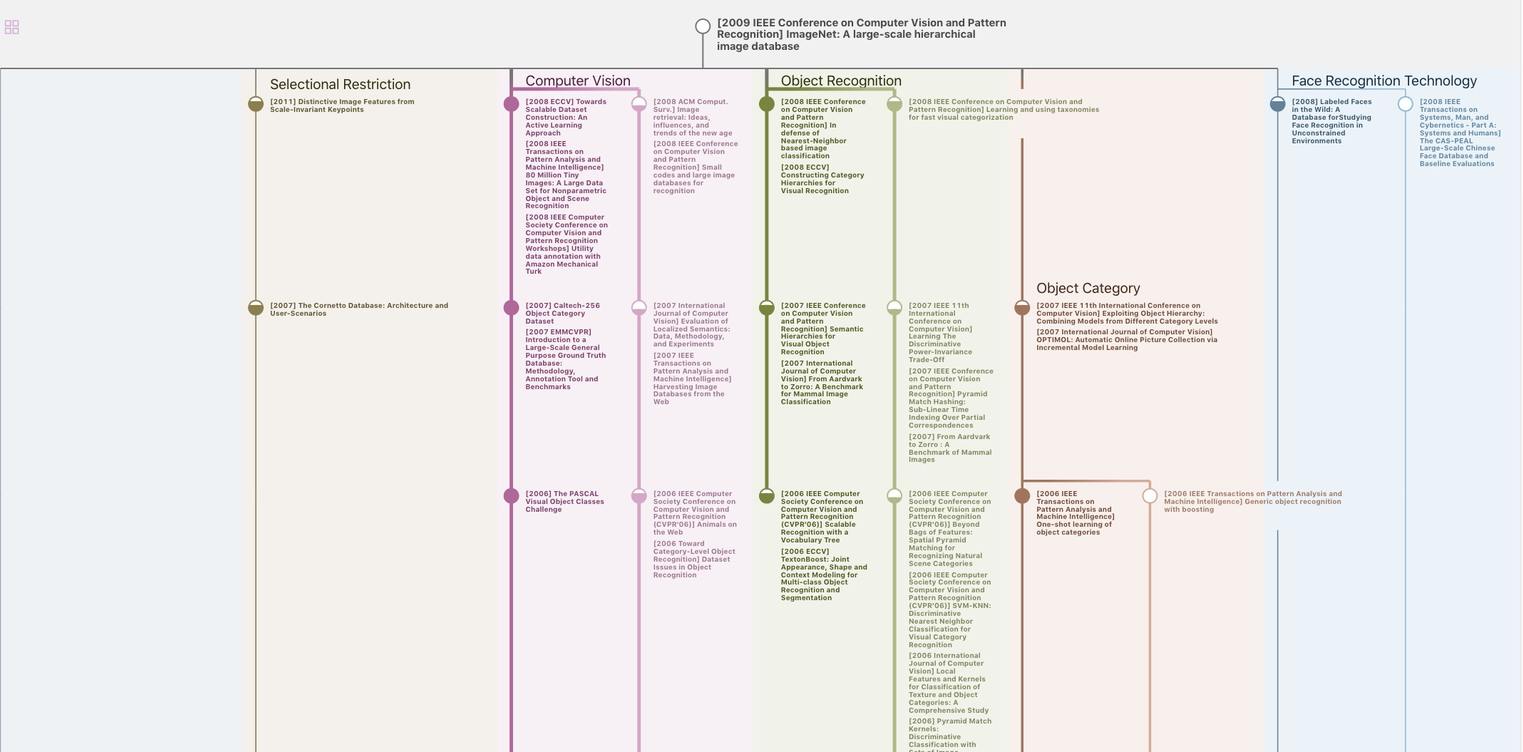
生成溯源树,研究论文发展脉络
Chat Paper
正在生成论文摘要