OplixNet: Towards Area-Efficient Optical Split-Complex Networks with Real-to-Complex Data Assignment and Knowledge Distillation
CoRR(2023)
摘要
Having the potential for high speed, high throughput, and low energy cost,
optical neural networks (ONNs) have emerged as a promising candidate for
accelerating deep learning tasks. In conventional ONNs, light amplitudes are
modulated at the input and detected at the output. However, the light phases
are still ignored in conventional structures, although they can also carry
information for computing. To address this issue, in this paper, we propose a
framework called OplixNet to compress the areas of ONNs by modulating input
image data into the amplitudes and phase parts of light signals. The input and
output parts of the ONNs are redesigned to make full use of both amplitude and
phase information. Moreover, mutual learning across different ONN structures is
introduced to maintain the accuracy. Experimental results demonstrate that the
proposed framework significantly reduces the areas of ONNs with the accuracy
within an acceptable range. For instance, 75.03% area is reduced with a 0.33%
accuracy decrease on fully connected neural network (FCNN) and 74.88% area is
reduced with a 2.38% accuracy decrease on ResNet-32.
更多查看译文
AI 理解论文
溯源树
样例
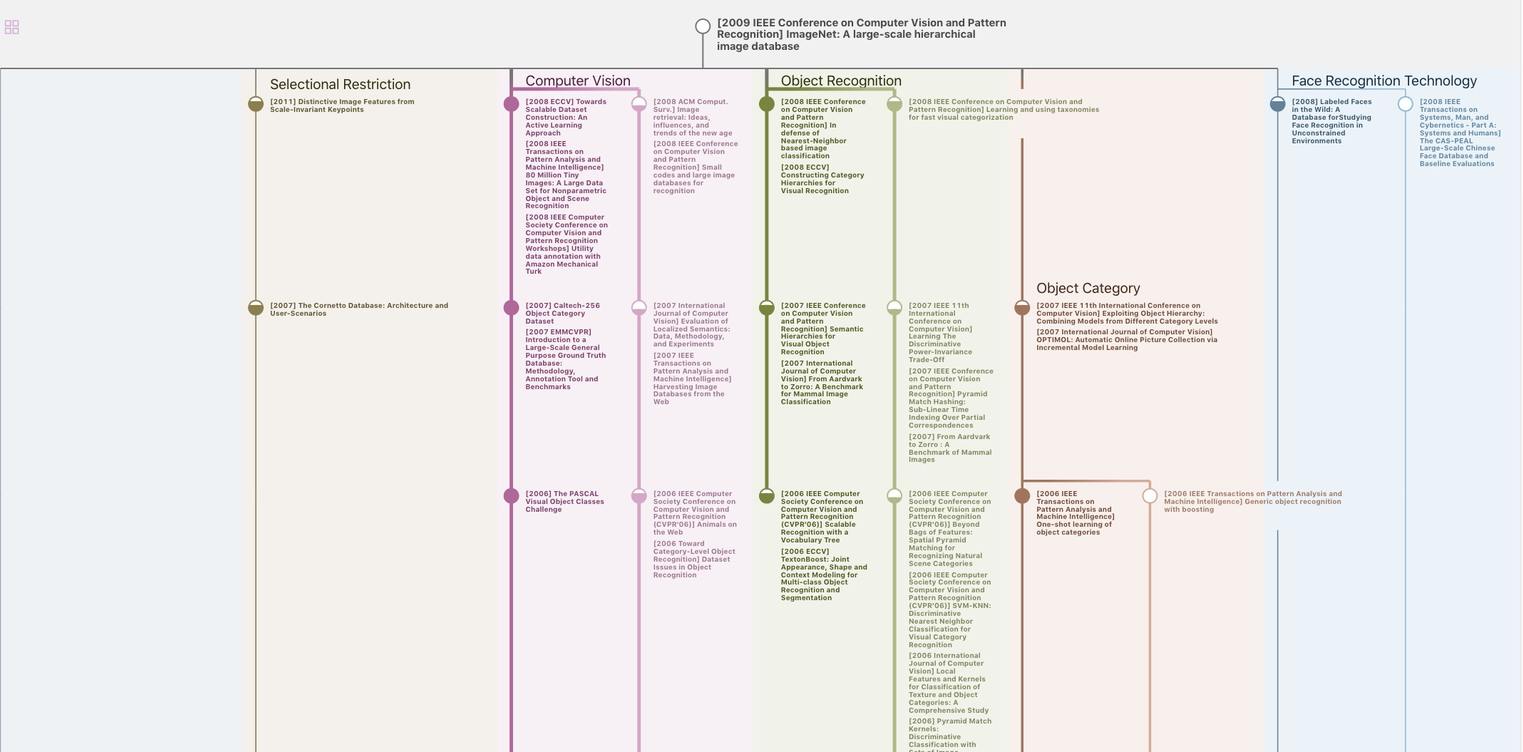
生成溯源树,研究论文发展脉络
Chat Paper
正在生成论文摘要