Level Set Learning for Poincar\'e Plots of Symplectic Maps
arxiv(2023)
摘要
Many important qualities of plasma confinement devices can be determined via the Poincar\'e plot of a symplectic return map. These qualities include the locations of periodic orbits, magnetic islands, and chaotic regions of phase space. However, every evaluation of the magnetic return map requires solving an ODE, meaning a detailed Poincar\'e plot can be expensive to create. Here, we propose a kernel-based method of learning a single labeling function that is approximately invariant under the symplectic map. From the labeling function, we can recover the locations of invariant circles, islands, and chaos with few evaluations of the underlying symplectic map. Additionally, the labeling function comes with a residual, which serves as a measure of how invariant the label function is, and therefore as an indirect measure of chaos and map complexity.
更多查看译文
AI 理解论文
溯源树
样例
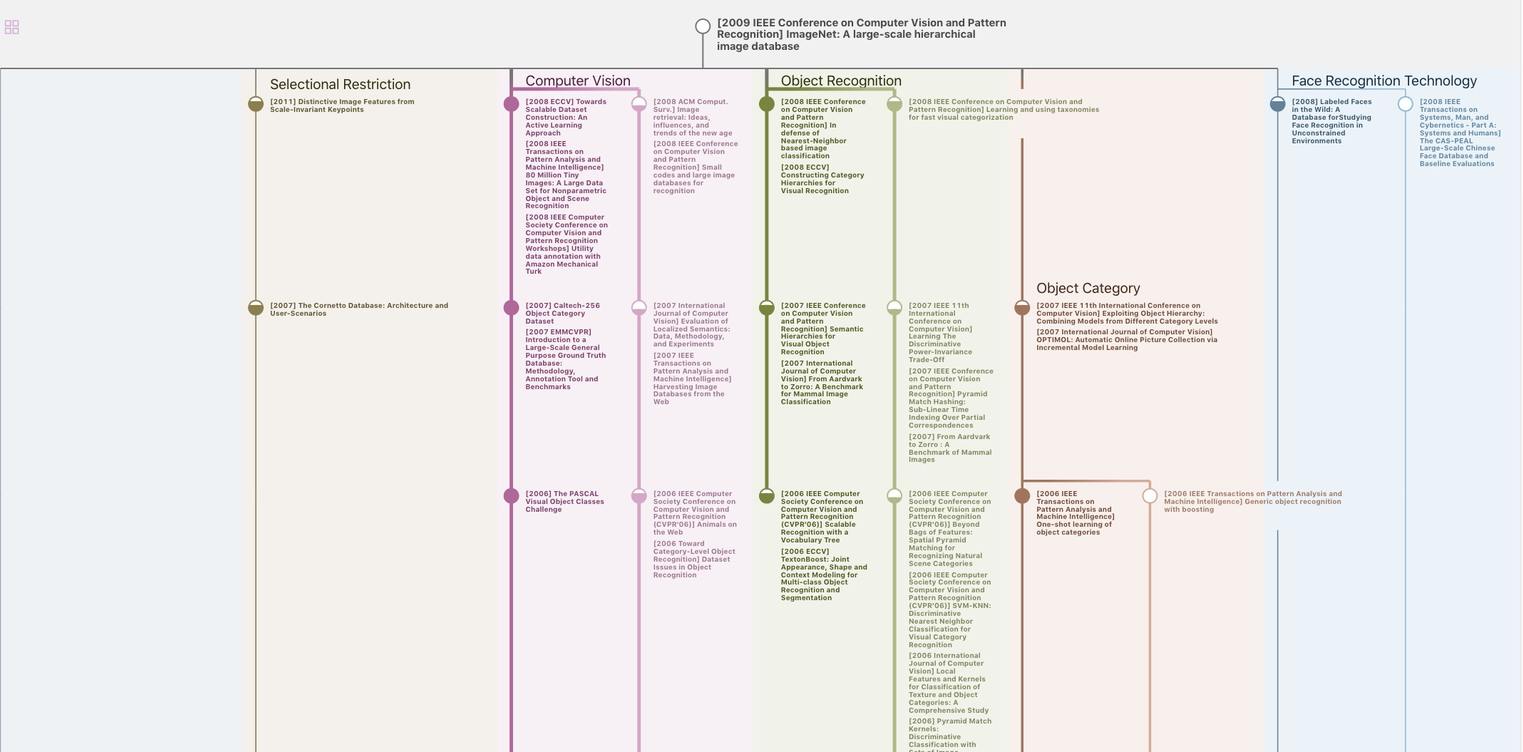
生成溯源树,研究论文发展脉络
Chat Paper
正在生成论文摘要