Quantifying climatic and socioeconomic drivers of urban malaria in Surat, India: a statistical spatiotemporal modelling study
LANCET PLANETARY HEALTH(2023)
摘要
Background Cities are becoming increasingly important habitats for mosquito vectors of disease. The pronounced heterogeneity of urban landscapes challenges our understanding of the effects of climate and socioeconomic factors on mosquito-borne disease dynamics at different spatiotemporal scales. Here, we quantify the impact of climatic and socioeconomic factors on urban malaria risk, using an extensive dataset in both space and time for reported Plasmodium falciparum cases in the city of Surat, northwest India. Methods We analysed 10 years of monthly Pfalciparum cases resolved at three nested spatial resolutions (seven zones, 32 units, and 478 worker units) with a Bayesian hierarchical mixed model that incorporates the effects of population density, poverty, relative humidity, and temperature, in addition to random effects (structured and unstructured). To reduce dimensionality and avoid correlation of covariates, socioeconomic variables from survey data were summarised into main axes of variation using principal component analysis. With model selection, we identified the main drivers of spatiotemporal variation in malaria incidence rates at each of the three spatial resolutions. We also compared observations to model-fitted cases by quantifying the percentage of predictions within five discrete levels of malaria risk. Findings The spatial variation of urban malaria cases was stationary over time, whereby locations with high and low yearly cases remained largely consistent across years. Local socioeconomic variation could be summarised with three principal components accounting for approximately 80% of the variance. The model that incorporated local temperature and relative humidity together with two of these principal components, largely representing population density and poverty, best explained monthly malaria patterns in models formulated at the three different spatial scales. As model resolution increased, the effect size of humidity decreased, whereas those of temperature and the principal component associated with population density increased. Model predictions accurately captured aggregated total monthly cases for the city; in space-time, they more closely matched observations at the intermediate scale, with around 57% of units estimated to fall in the observed category on average across years. The mean absolute error was lower at the intermediate level, showing that this is the best aggregation level to predict the space-time dynamics of malaria incidence rates across the city with the selected model. Interpretation This statistical modelling framework provides a basis for development of a climate-driven early warning system for urban malaria for the units of Surat, including spatially explicit prediction of malaria risk several weeks to months in advance. Results indicate environmental and socioeconomic covariates for which further measurement at high resolution should lead to model improvement. Advanced warning combined with local surveillance and knowledge of disease hotspots within the city could inform targeted intervention as part of urban malaria elimination efforts.
更多查看译文
AI 理解论文
溯源树
样例
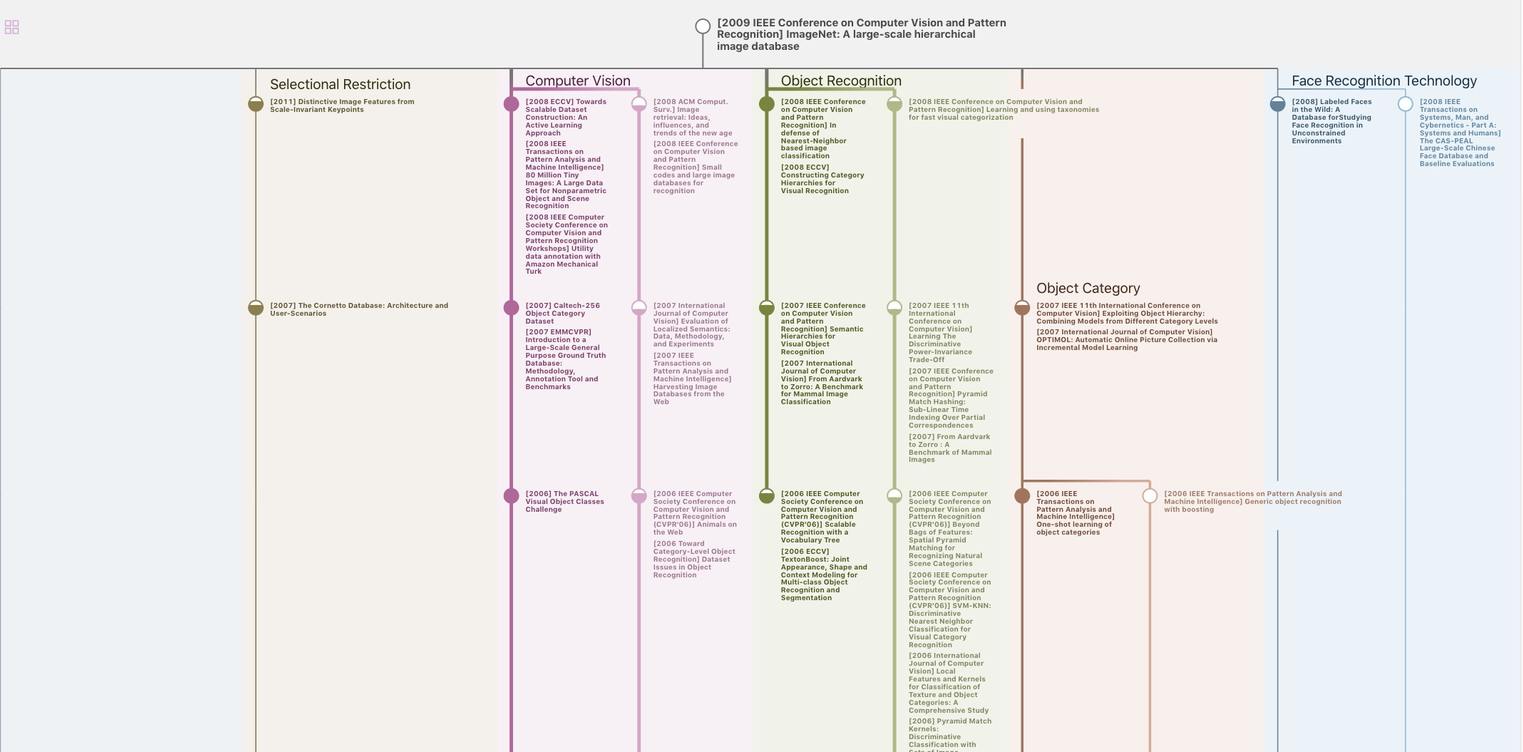
生成溯源树,研究论文发展脉络
Chat Paper
正在生成论文摘要