Environmental factors for outdoor jogging in Beijing: Insights from using explainable spatial machine learning and massive trajectory data
LANDSCAPE AND URBAN PLANNING(2024)
摘要
Outdoor jogging, as a physical exercise beneficial for health, has proliferated worldwide. However, under -standing the nonlinear and heterogeneous associations between environmental factors and jogging behavior remains challenging. This study established an explainable spatial machine learning framework combining Geographically Weighted-Random Forest (GW-RF) and SHapley Additive exPlanation (SHAP) model to address the nonlinearity, spatial heterogeneity, and interpretability. Using large-scale GPS trajectories and multi-source big data, this study provides the global and local explanations of nonlinear associations in Beijing, China. Our findings highlight that (1) Built Environment (BE) factors play a more important role than visual landscape factors in determining jogging behavior, with sports facilities such as tracks and parks having higher contribu-tions. (2) All environmental variables, including the BE, visual landscape, and social economics, exhibit nonlinear and threshold effects on jogging behavior. (3) Certain factors such as population density, number of parks, Greening View Index, and Sky View Index exhibit differential effects across different periods, regions, and mobility patterns of jogging. (4) Compared with the Ordinary Least Squares model, Geographically Weighted Regression model, and Random Forest model, GW-RF model demonstrates improved performance in modeling and predicting the jogging flow. The findings have important implications for urban planners seeking to create a supportive environment that promotes outdoor jogging.
更多查看译文
关键词
Jogging activity,Environmental factors,Geographically weighted random forest,Nonlinear associations,Explainable machine learning
AI 理解论文
溯源树
样例
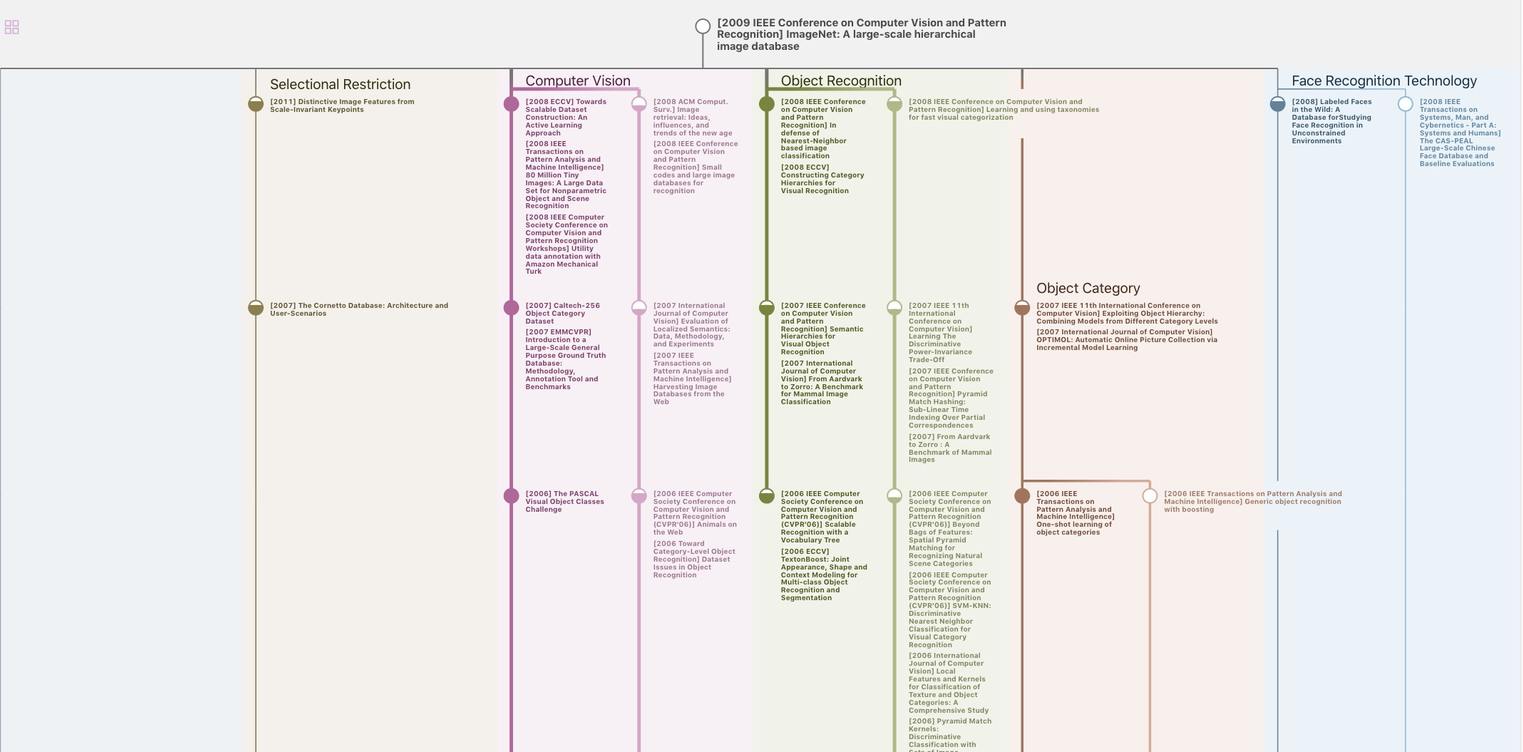
生成溯源树,研究论文发展脉络
Chat Paper
正在生成论文摘要