Mixed-type wafer defect detection based on multi-branch feature enhanced residual module
EXPERT SYSTEMS WITH APPLICATIONS(2024)
摘要
Wafer testing is crucial in semiconductor manufacturing. Accurate identification of wafer defects enables precise localization of manufacturing issues, thereby enhancing chip production yield. Mixed-type wafer defect patterns are complex, resulting from the combination of different single-type defects, exhibiting variations in shape, size, and distribution. Currently, most deep learning models for classifying mixed-type wafer defect patterns are complex and require further refinement to enhance their effectiveness. Therefore, this study proposes a deep learning model called MER-Net (Multi-branch Feature Enhanced Residual Network). By adjusting deformable convolution and Ghost convolution, a multi-branch feature enhanced residual module is crafted. This module serves as the foundation for a deep learning model designed to classify mixed-type wafer defects. To validate the effectiveness of the proposed model, experiments were conducted using a dataset comprising 38 types of mixedtype wafer defects. The experimental results reveal that this method achieves a recognition accuracy of 98.84% on the mixed-type wafer defect dataset. In comparison to existing deep learning models, this method demonstrates higher accuracy and superior performance. These findings further underscore the feasibility and effectiveness of the approach presented in this study.
更多查看译文
关键词
Semiconductor manufacturing,Wafer map,Defect detection,Feature enhancement
AI 理解论文
溯源树
样例
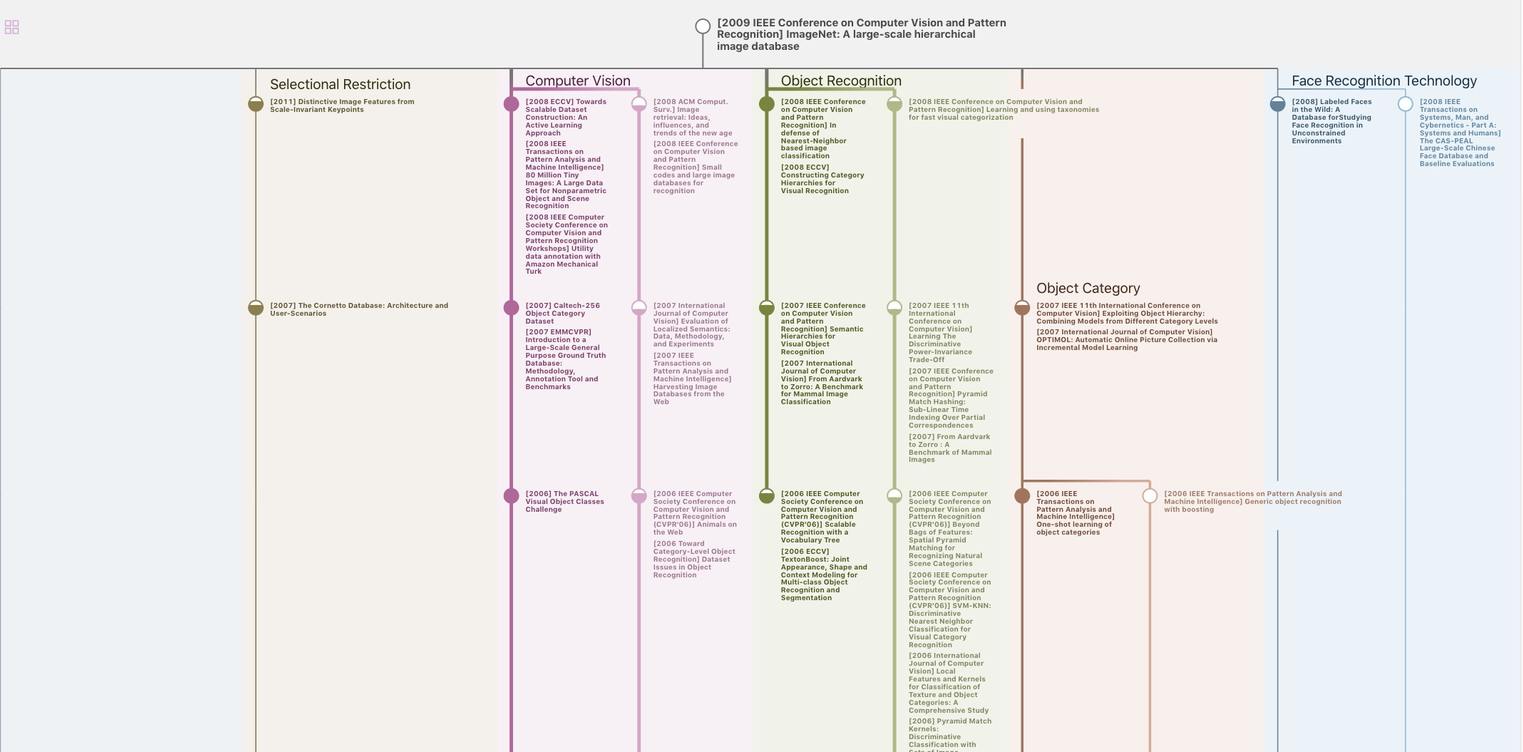
生成溯源树,研究论文发展脉络
Chat Paper
正在生成论文摘要