Numerical optimization of Lenalidomide immunomodulatory drug inside the supercritical carbon dioxide system using different machine learning models
JOURNAL OF MOLECULAR LIQUIDS(2024)
摘要
supercritical fluids (SCFs) have been introduced as a reliable alternative for conventional organic solvents due to their unique gases-like and liquids-like behavior above the critical point. Nowadays, industrial-based employment of SCFs (especially SCCO2) for increasing the solubility of low water-soluble orally-taken medicines has increased significantly due to their noteworthy advantages like eco-friendliness, low carcinogenicity/toxicity, high technical efficiency, accessible critical points and affordable cost. The main aim is to design, optimize and modify the solubility of the Lenalidomide orally-administered immunomodulatory drug in SC-CO2 as a function of temperature (T) and pressure (P). Models are built as a novel model for using a variety of machine learning techniques, such as the Least Absolute Shrinkage and Selection Operator (LASSO), Multilayer Perceptron (MLP), and Support Vector Model (SVM). These models tuned on their hyper-parameters and then evaluated using different metrics. In terms of RMSE, LASSO, MLP, and SVM have error values equal to 9.978 x 10-2, 2.545 x 10-2, and 1.259 x 10-1, respectively. Also, the LASSO model has an R2 score of 0.9043, this score is 0.9950 for MLP and 0.9258 for SVM models. Accordingly, the MLP is the model that fits best on the solubility of Lenalidomide among these models and selected for rest of analysis in this study.
更多查看译文
关键词
Solubility,Optimization,Oral administration,Predictive modeling,Machine learning
AI 理解论文
溯源树
样例
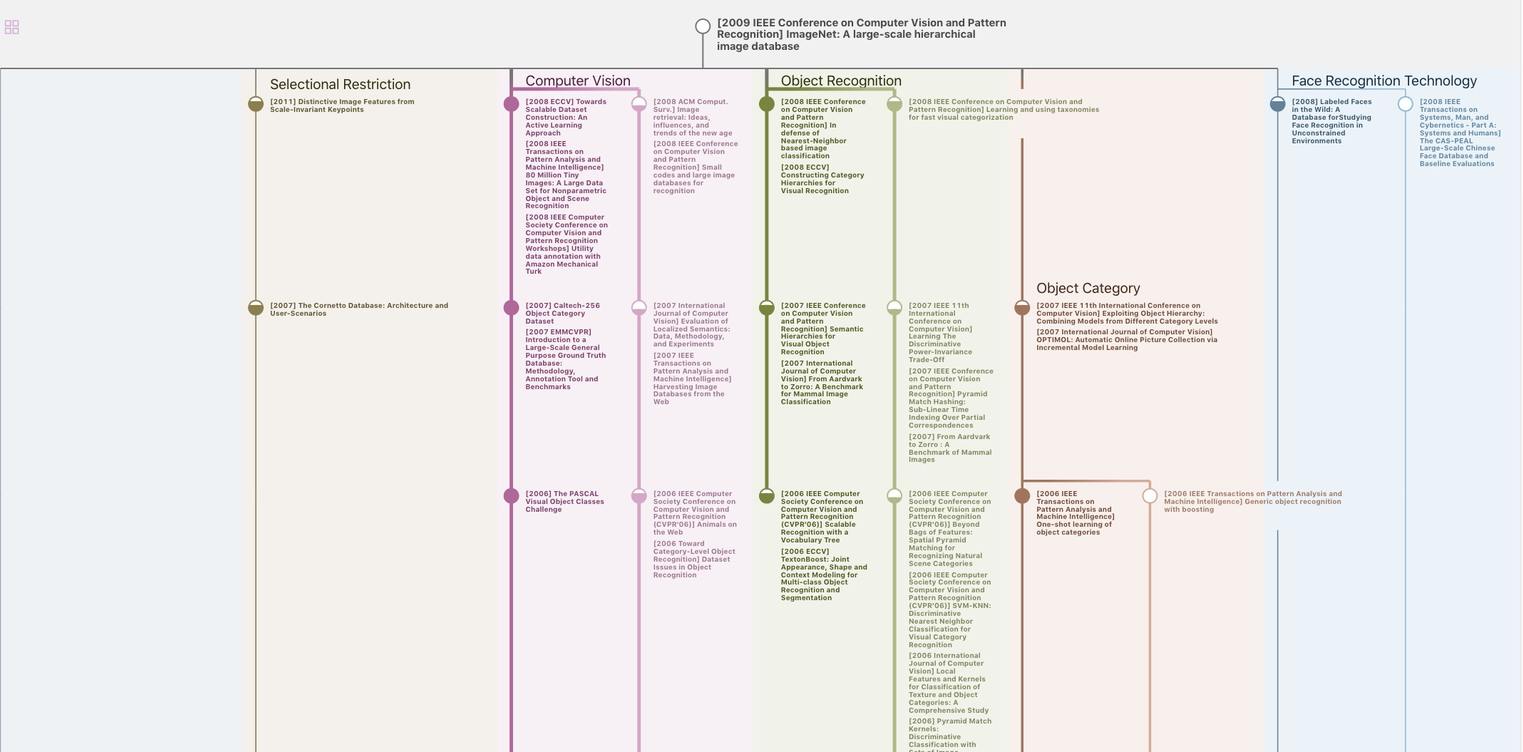
生成溯源树,研究论文发展脉络
Chat Paper
正在生成论文摘要