The triple refinement of self-paced learning style for unsupervised cross-domain person re-identification
IMAGE AND VISION COMPUTING(2024)
摘要
Unsupervised cross-domain person re-identification based on clustering typically involves generating pseudo -labels for all training data. However, some data are prone to generating incorrect pseudo-labels in the early stages of training, which leads to a decrease in the accuracy of the model. Based on the idea of self-paced learning, from easy to difficult and from a few to many samples, we propose the Triple Refinement of Self -paced Learning style (TRSL) method to solve this problem. Firstly, we use the density-based clustering algo-rithm DBSCAN to perform the first data refinement on the unlabeled data. Then, in the second refinement step, we calculate the challenge value by exploring the distance distribution between the clustering data and the centroids to refine the data. In the third refinement step, we calculate the credibility value of the data by comparing the k-nearest neighbor ranking lists of the local and global features of the images, use the credibility value to recall the discarded data from the dual refinement process, and perform a third filtering on the retained data. Finally, the processed data is input into a pair of mutually beneficial learning models for training. On top of a strong baseline, our method performs better than the recent state-of-the-art methods in some evaluation metrics.
更多查看译文
关键词
Self-paced learning,Data refinement,Person re-identification,Mutually learning models
AI 理解论文
溯源树
样例
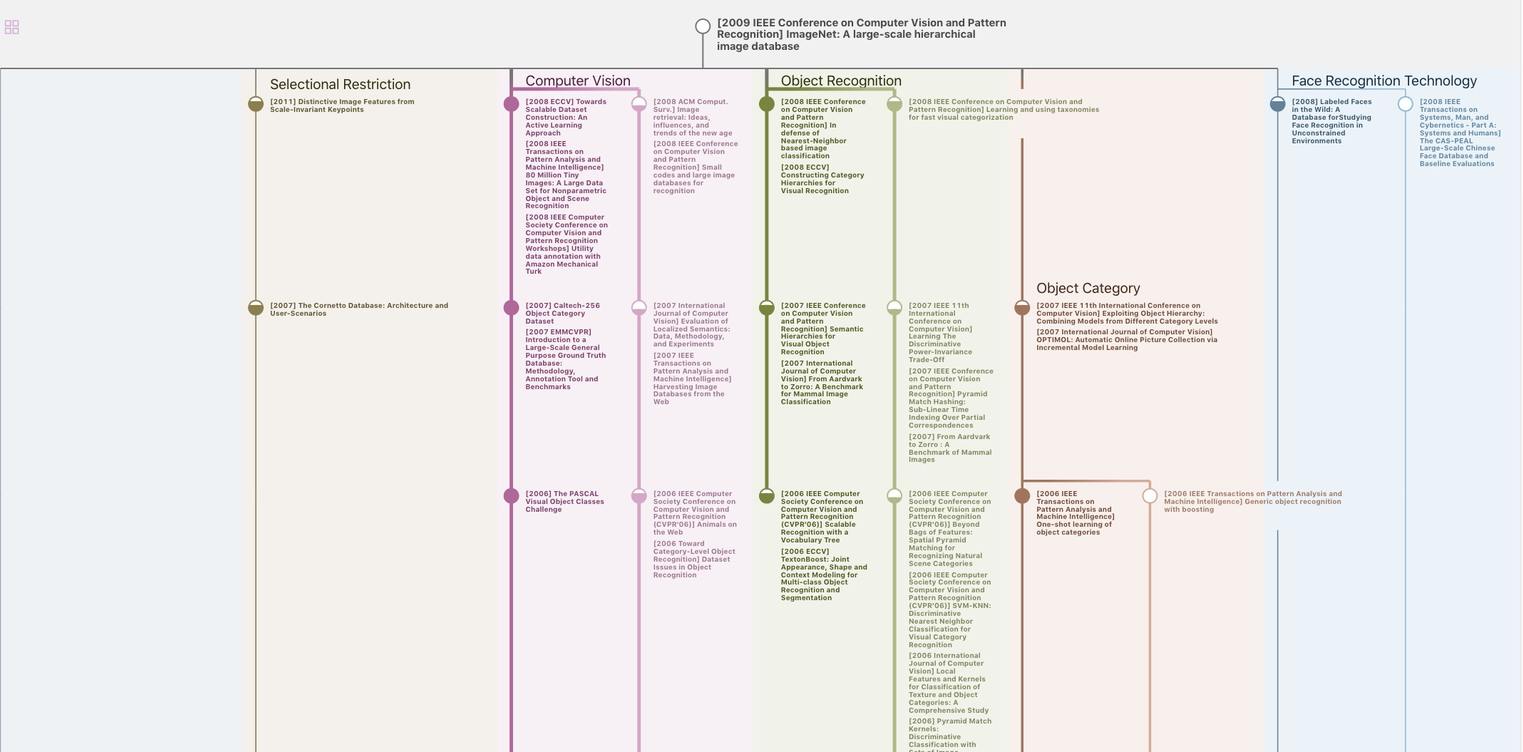
生成溯源树,研究论文发展脉络
Chat Paper
正在生成论文摘要