Segmentation of hard exudate lesions in color fundus image using two-stage CNN-based methods
EXPERT SYSTEMS WITH APPLICATIONS(2024)
摘要
The presence of hard exudate (EX) lesions is an early clinical symptom of Diabetic Retinopathy (DR); its accurate segmentation is essential for diagnosis and treatment. Automatic segmentation of EX lesions is challenging because they have a wide range of sizes, shapes, and brightness, and can be confused with soft exudate lesions. In this paper, we present and assess an efficient segmentation method for interactively segmenting EX lesions in fundus images from DR patients. Our approach consisted of two main stages: (1) the first stage generates an automatic segmentation using a CNN-based method. (2) If the obtained segmentation is suboptimal, the second stage is used to refine each inaccurately segmented region using a CNN-based interactive segmentation method. To train and evaluate method performance, we used two public datasets (IDRiD and DDR) and two local datasets from two medical centers (EHos and EWT) containing a wide range of EX lesions. Several state-of-the art CNN-based models and the proposed method were trained and evaluated on IDRiD, DDR and EHos datasets using the area under the precision-recall curve (AUPR), the dice similarity coefficient (Dice) and the intersection over union (IoU). In addition, EWT dataset was used to evaluate the methods without retraining the CNN models. The evaluation results using IDRiD dataset showed that the proposed method achieved AUPR, mean Dice and mean IoU scores of 0.893, 76.6% and 62.4%, respectively, which are comparable to the state-of-the-art method on EX segmentation. When evaluated on the DDR dataset with large portion of small EX lesions, the proposed method achieved AUPR, mean Dice and mean IoU scores of 0.693, 60.8% and 45.5%, yielding state-of-the-art performance. Furthermore, with the main evaluation metric being AUPR, the evaluation results on two datasets, EHos and ETW, with scores of 0.689 and 0.667 demonstrated superiority of the proposed method over the other segmentation methods. The proposed two -stage CNN-based segmentation method is an useful approach for accurately segmenting EX lesions in color fundus images, enabling lesion quantification to aid experts in treating patients with DR. The source code, the trained CNN models and the data are publicly available at: https://github.com/dvquang2000/Interactive_ HardExudates_Segmentation.
更多查看译文
关键词
Hard exudates,Deep learning,Automatic segmentation,Interactive segmentation
AI 理解论文
溯源树
样例
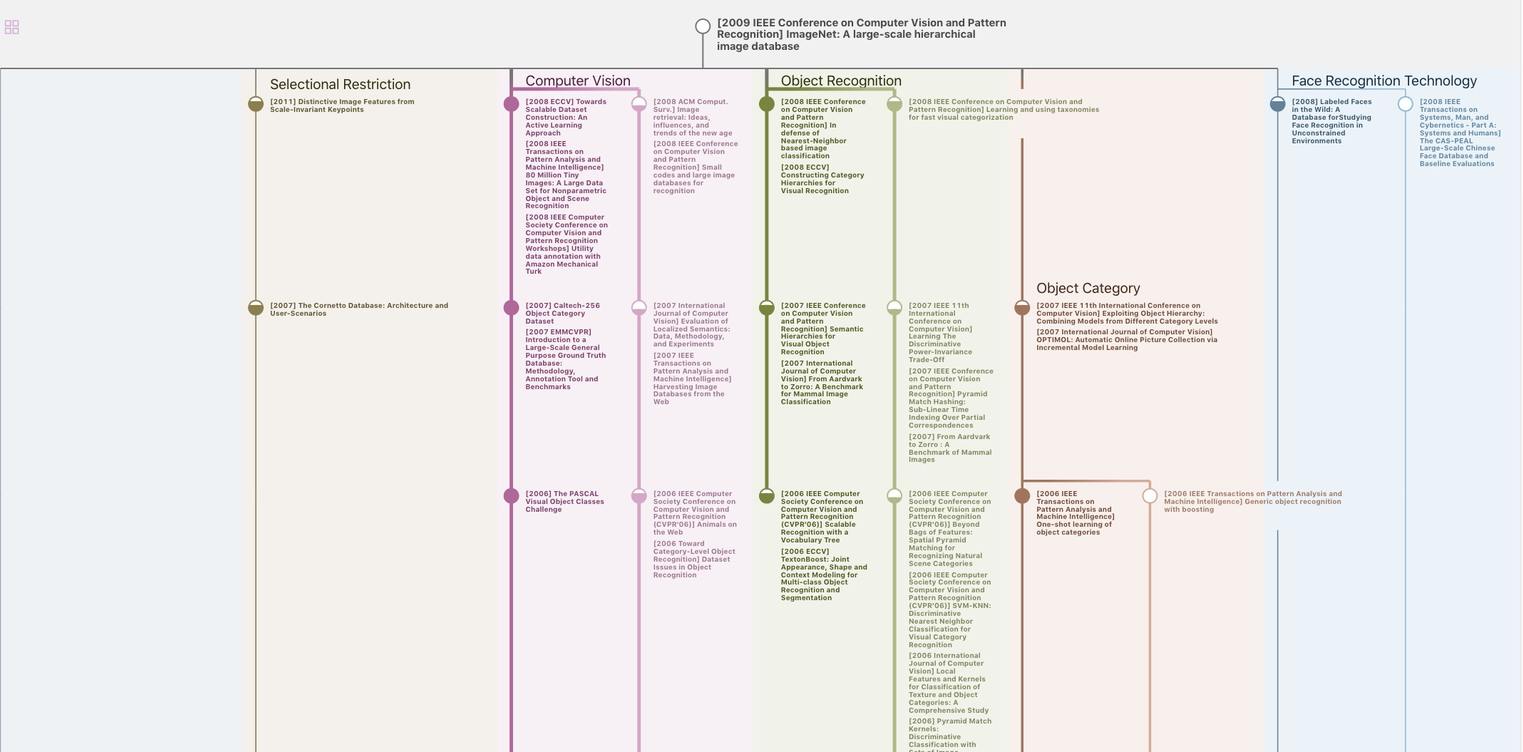
生成溯源树,研究论文发展脉络
Chat Paper
正在生成论文摘要