An empirical evaluation of unsupervised event log abstraction techniques in process mining
INFORMATION SYSTEMS(2024)
摘要
These days, businesses keep track of more and more data in their information systems. Moreover, this data becomes more fine-grained than ever, tracking clicks and mutations in databases at the lowest level possible. Faced with such data, process discovery often struggles with producing comprehensible models, as they instead return spaghetti-like models. Such finely granulated models do not fit the business user's mental model of the process under investigation. To tackle this, event log abstraction (ELA) techniques can transform the underlying event log to a higher granularity level. However, insights into the performance of these techniques are lacking in literature as results are only based on small-scale experiments and are often inconclusive. Against this background, this paper evaluates state-of-the-art abstraction techniques on 400 event logs. Results show that ELA sacrifices fitness for precision, but complexity reductions heavily depend on the ELA technique used. This study also illustrates the importance of a larger-scale experiment, as sub-sampling of results leads to contradictory conclusions.
更多查看译文
关键词
Process mining,Event log abstraction,Unsupervised learning,Comprehensibility
AI 理解论文
溯源树
样例
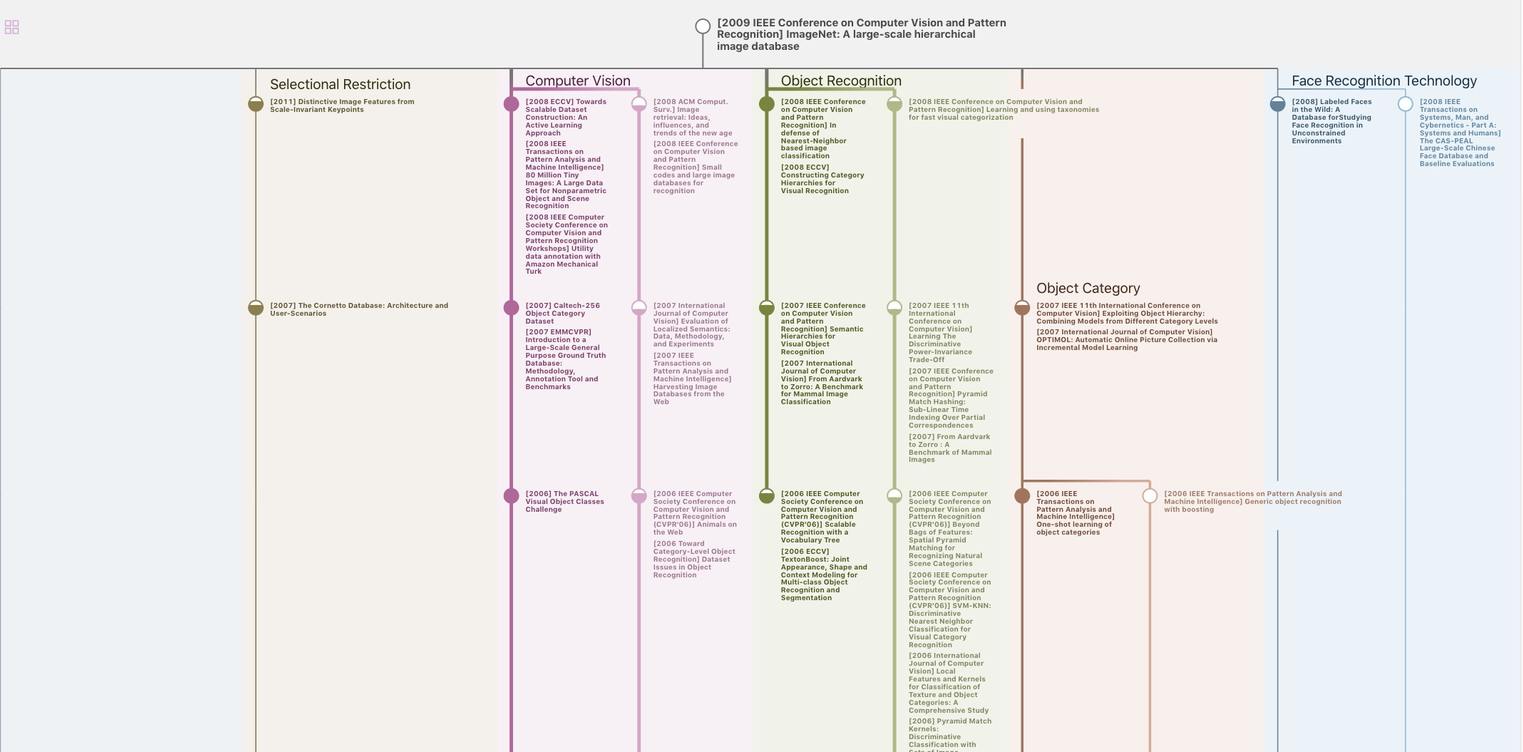
生成溯源树,研究论文发展脉络
Chat Paper
正在生成论文摘要