Predicting post-liver transplant outcomes in patients with acute-on-chronic liver failure using Expert-Augmented Machine Learning
AMERICAN JOURNAL OF TRANSPLANTATION(2023)
摘要
Liver transplantation (LT) is a treatment for acute-on-chronic liver failure (ACLF), but high post-LT mortality has been reported. Existing post-LT models in ACLF have been limited. We developed an Expert-Augmented Machine Learning (EAML) model to predict post-LT outcomes. We identified ACLF patients who underwent LT in the University of California Health Data Warehouse. We applied the RuleFit machine learning (ML) algorithm to extract rules from decision trees and create intermediate models. We asked human experts to rate the rules generated by RuleFit and incorporated these ratings to generate final EAML models. We identified 1384 ACLF patients. For death at 1 year, areas under the receiver-operating characteristic curve were 0.707 (confidence interval [CI] 0.625-0.793) for EAML and 0.719 (CI 0.640-0.800) for RuleFit. For death at 90 days, areas under the receiver-operating characteristic curve were 0.678 (CI 0.581-0.776) for EAML and 0.707 (CI 0.615-0.800) for RuleFit. In pairwise comparisons, both EAML and RuleFit models outperformed cross-sectional models. Significant discrepancies between experts and ML occurred in rankings of biomarkers used in clinical practice. EAML may serve as a method for ML -guided hypothesis generation in further ACLF research.
更多查看译文
关键词
ACLF,big data,UCHDW,machine learning,posttransplant outcomes
AI 理解论文
溯源树
样例
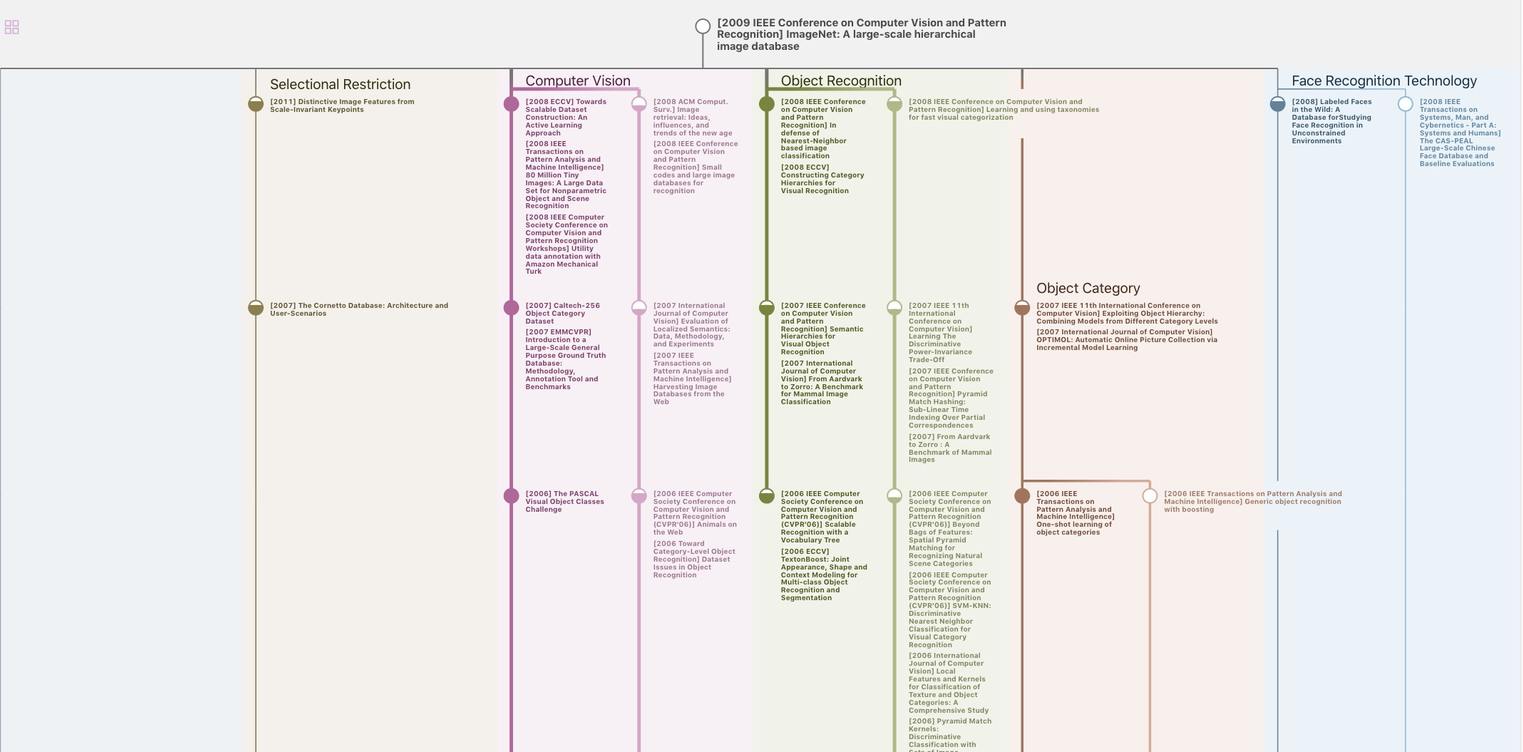
生成溯源树,研究论文发展脉络
Chat Paper
正在生成论文摘要