Transfer learning in environmental remote sensing
REMOTE SENSING OF ENVIRONMENT(2024)
摘要
Machine learning (ML) has proven to be a powerful tool for utilizing the rapidly increasing amounts of remote sensing data for environmental monitoring. Yet ML models often require a substantial amount of ground truth labels for training, and models trained using labeled data from one domain often demonstrate poor performance when directly applied to other domains. Transfer learning (TL) has emerged as a promising strategy to address domain shift and alleviate the need for labeled data. Here we provide the first systematic review of TL studies in environmental remote sensing. We start by defining the different forms of domain shift and then describe five commonly used TL techniques. We then present the results of a systematic search for peer-reviewed articles published between 2017 and 2022, which identified 1676 papers. Applications of TL in remote sensing have increased rapidly, with nearly 10 times more publications in 2022 than in 2017. Across seven categories of applications (land cover mapping, vegetation monitoring, soil property estimation, crop yield prediction, biodiversity monitoring, water resources management, and natural disaster management) we identify several recent successes of TL as well as some remaining research gaps. Finally, we highlight the need to organize benchmark datasets explicitly for TL in remote sensing for model evaluation. We also discuss potential research directions for TL studies in environmental remote sensing, such as realizing scale transfer, improving model interpretability, and leveraging foundation models for remote sensing tasks.
更多查看译文
关键词
Remote sensing,Domain shift,Transfer learning,Unsupervised domain adaptation,Multi-task learning,Few-shot learning,Self-supervised learning
AI 理解论文
溯源树
样例
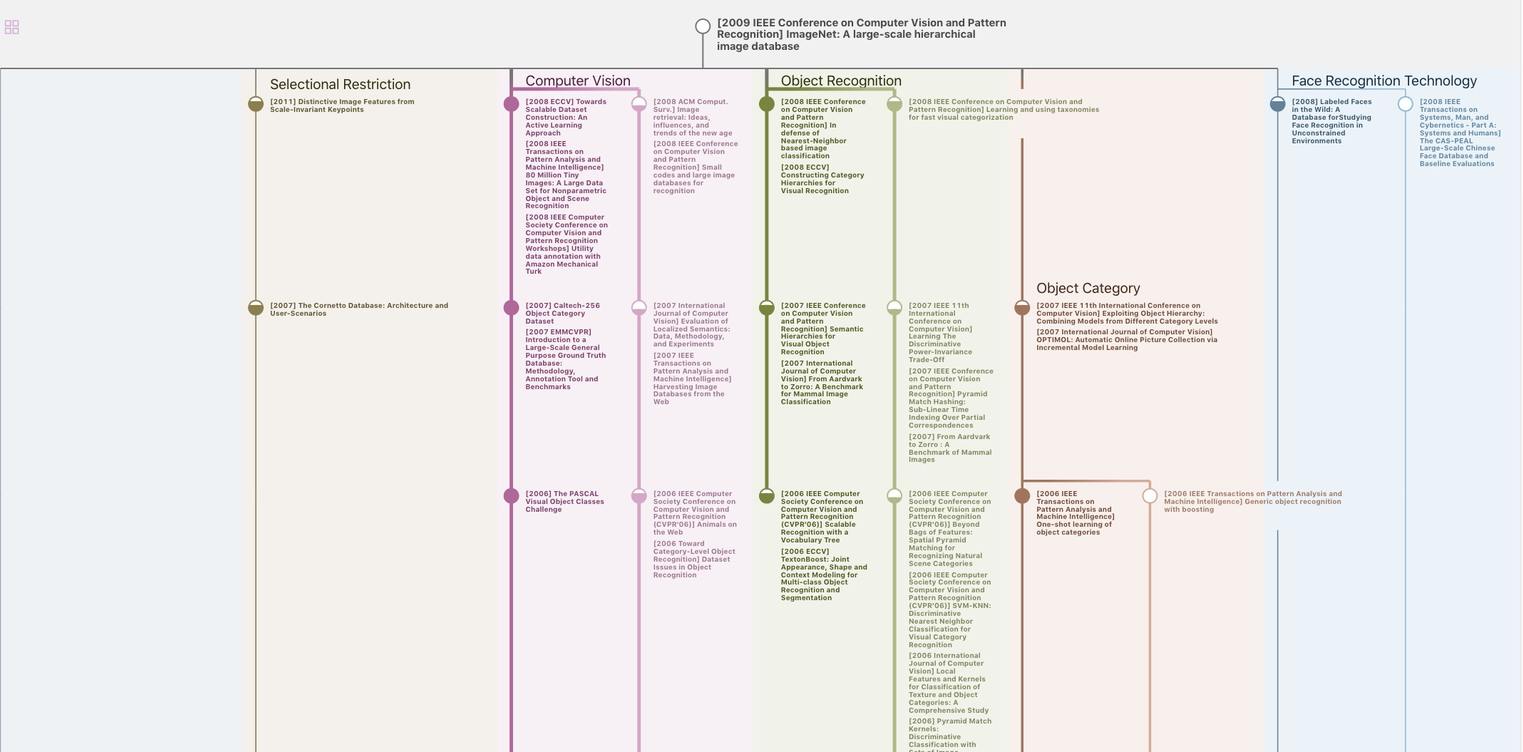
生成溯源树,研究论文发展脉络
Chat Paper
正在生成论文摘要