Rotation-equivariant correspondence matching based on a dual-activation mixer
NEUROCOMPUTING(2024)
摘要
Learning-based correspondence matching methods have become the mainstream techniques in many computer vision and robotics applications due to their robustness to large illumination and viewpoint changes. However, it is difficult for conventional convolutional neural networks (CNNs) to extract rotation-equivariant local features. Recent work has shown that CNNs combined with group-equivariant architectures are surprisingly effective at matching correspondences even when the images are rotated to a dramatic extent. However, the inherent shape (square) of convolution kernels causes the performance bottleneck of such rotation-equivariant CNNs. To address this issue, we propose an adaptive dual rotation-equivariant correspondence matching algorithm, which performs stably at all angles. We mathematically analyze the effectiveness of our proposed rotation-equivariant correspondence matching approach and its performance with respect to different convolution kernels. Extensive experiments on the Rotated-HPatches, SIM2E, and MegaDepth datasets demonstrate the effectiveness of our proposed algorithm.
更多查看译文
关键词
Correspondence matching,Convolutional neural networks,Rotation-equivariant visual feature,Rotation-invariant visual feature,Inlier ratio
AI 理解论文
溯源树
样例
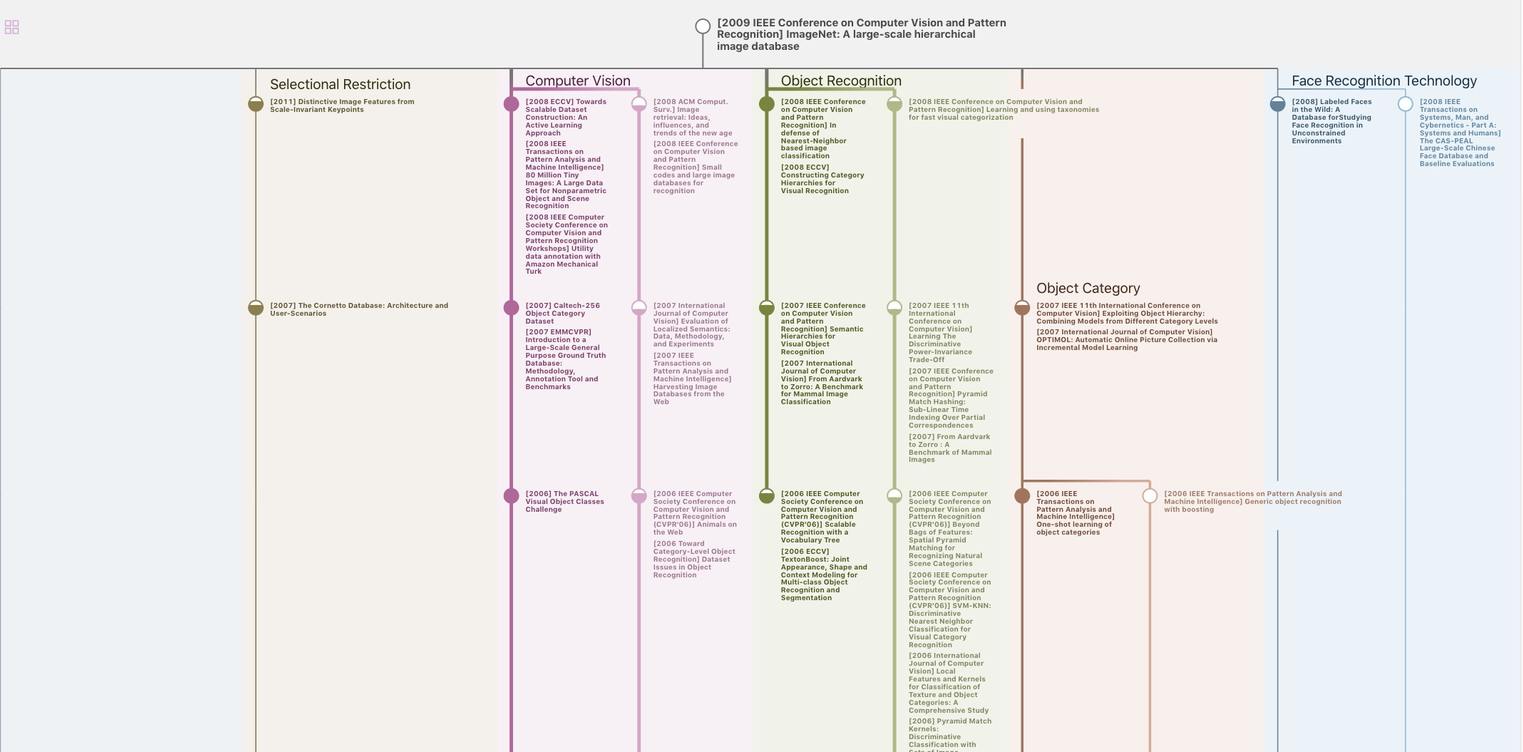
生成溯源树,研究论文发展脉络
Chat Paper
正在生成论文摘要